
- Doing a PhD in Artificial Intelligence
Artificial intelligence and intelligent systems are thought to be the key to the next ‘industrial revolution’. In a data-rich world, developing an artificial intelligence which can learn from its experience and call on human behaviour to make decisions could change the way we live and offer endless economic, social and scientific applications. Nationwide, there is an increasing demand for AI workers, as the world is becoming more reliant on developing technology and automated systems. Consequently, more and more people are pursuing postgraduate research in AI.

What Does a PhD in Artificial Intelligence Focus On?
A PhD in artificial intelligence will give you a deep understanding of AI, allow you to contribute to the development of emerging technology, and equip you with highly applicable technical skills. For example, engineering applications of artificial intelligence include automation of tasks and parametric modelling. Medical applications include using data-science approaches to identify patterns of illness in clinical data. Financial applications include using machine learning platforms to crunch huge amounts of data and help credit lenders in analysing risk and assess potential borrowers.
Artificial Intelligence PhD programme can focus on:
- Machine learning
- Deep learning
- Natural language processing
- Deep neural network architecture
- Human-machine interaction
- Augmented reality
- And countless other areas
Browse PhDs in Artificial Intelligence
Application of artificial intelligence to multiphysics problems in materials design, from text to tech: shaping the future of physics-based simulations with ai-driven generative models, study of the human-vehicle interactions by a high-end dynamic driving simulator, coventry university postgraduate research studentships, discovery of solid state electrolytes using deep learning, entry requirements for a phd in artificial intelligence.
The entry requirements for a PhD in AI are typically an upper second-class honours degree (or international equivalent) in a relevant subject from an accredited university. Subjects considered relevant to artificial intelligence include computer science, engineering, mathematics, statistics, electronics/electrical engineering or science.
Some research courses also require applicants to possess experience in programming, the desired programming language will be specific to the research project. Academic or work experience in machine learning or data science are typically favourable for applications.
International students will also need to meet several minimum English language requirements set by the university, usually as part of a TOEFL or IELTS exam.
Duration and Programme Types
Like most PhDs, a doctoral programme in Artificial Intelligence typically takes 3-4 years full-time, or 6 years part-time .
Aside from the traditional PhD, there is also the CDT PhD. Many Universities have Centres for Doctoral Training (CDTs) which are often funded by the UKRI centre . These CDTs can offer the CDT PhD which is a specialised PhD programme in artificial intelligence. The main difference between a standard PhD in artificial intelligence and a CDT PhD in artificial intelligence is that the latter includes additional modules which give candidates training in neuroscience, entrepreneurship, high performance computing, AI ethics and science communication.
Due to the different research areas you can pursue within the artificial intelligence field, the nature of programmes can vary. Some PhD research programmes are computational based and heavily reliant on coding, mathematics and lab work. Other research programmes can be people facing, involving questionnaires, for example to determine public perception on proposed legislation.
Costs and Funding
Annual tuition fees for PhDs in Artificial Intelligence are typically around £4,000 to £5,000 for UK/EU students. Tuition fees for international students are usually much higher, typically around £25,000 per academic year.
A variety of scholarships and funding support options are available for postgraduate study. For Artificial Intelligence in particular, the UKRI and ESPRC offer a number of studentships and CDT opportunities across varies universities. Many universities have research centres which are partnered with UK research councils and offer fully funded programmes. Funding is generally available for UK/EU students. International students are also eligible for some funding opportunities, but these tend to be less widely available.
Available Career Paths in AI
One of the key advantages of Artificial Intelligence is that it has a wide range of applications, and hence there are many career paths available. As computer systems and data have become more integrated in everyday life, the demand for experts in AI has grown rapidly. This high demand has resulted in many high job security and lucrative salaries.
Examples destinations for an AI PhD student include:
Data Analyst – If you are very analytical research student, you may use your artificial intelligence PhD to pursue a career in data science or analysis. Data analysts can be found in engineering, finance, healthcare, and everywhere in between. They are responsible for data crunching and using their skills to present complex information in a clear manner – visually and orally. Typical duties include record management, maintaining automated processes, monitoring analytics and KPIs, improving algorithms, and creating dashboards for clients. The average salary for data analysts in the UK is around £30,000 – £45,000, though this number can increase drastically depending on the sector.

Cyber Security – As cyber-attacks are becoming more commonplace, industries are looking to develop their cyber security, and salaries are seeing a sharp increase accordingly. AI doctorates are well placed for a career in cyber security, and typical career destinations include security analysts, penetration testers, systems engineers, web developers and cybersecurity consultants. In these roles, you will be responsible for protecting IT infrastructure and help develop security systems.
Machine learning – Often those with a PhD in AI become machine learning engineers, responsible for the development of intelligent systems. Machine learning is a subset of AI which focuses on the idea that machines can be programmed to learn from data and experience to improve decision making without human input. Machine learning is perhaps at the forefront of AI research, and there are numerous programmes look to improve its capabilities. This is well suited for those who enjoy the mathematical and programming side of computer science. Typical duties include managing data pipelines, developing algorithms, liaising with stakeholders, analysing datasets, and leading software design. Entry level salaries are around £35,000 and can exceed £150,000 with experience. Deep learning is similar to machine learning, the main difference is that deep learning aims to create artificial ‘neural networks’.
Postgraduate research often leads to an academic career. As an academic you can propose your own research projects based on your interest and supervise students. As a professor you can shape the next generation of AI experts and as a researcher you can make use of a university’s department resources, facilities and industrial ties to work with cutting edge technology and push the boundaries of our knowledge.
Browse PhDs Now
Join thousands of students.
Join thousands of other students and stay up to date with the latest PhD programmes, funding opportunities and advice.
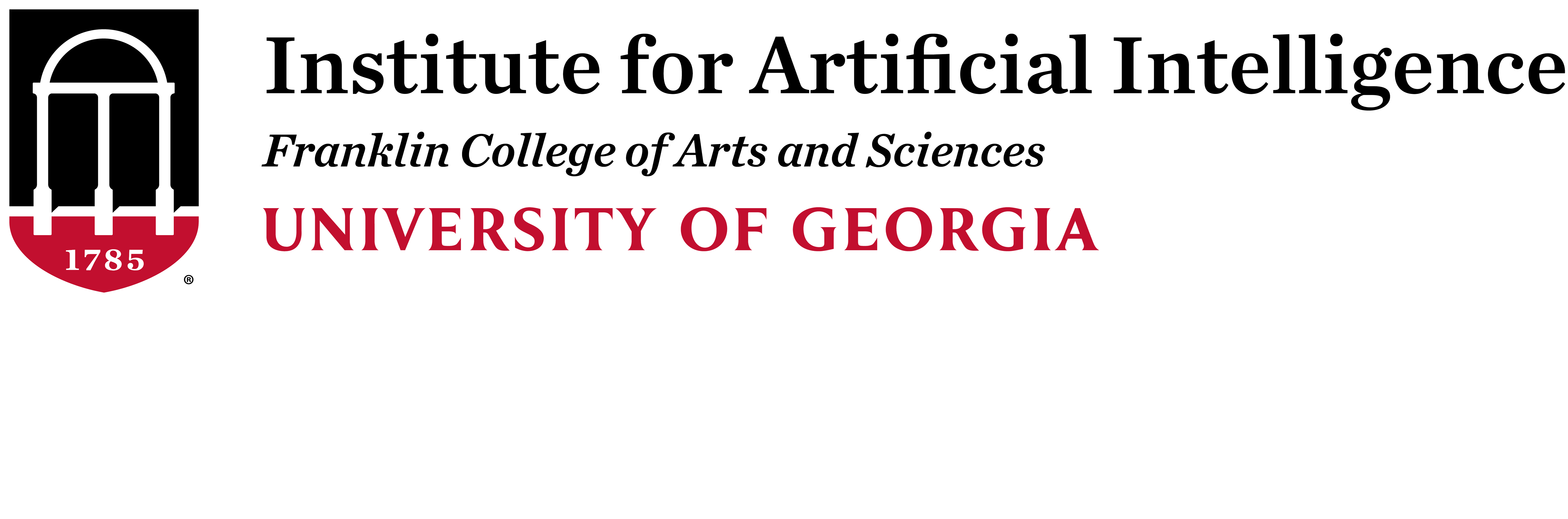
PhD in Artificial Intelligence
To enter the Doctor of Philosophy in Artificial Intelligence, you must apply online through the UGA Graduate School web page . There is an application fee, which must be paid at the time the application is submitted.
There are several items which must be included in the application:
- Standardized test scores, including the GRE.
- 3 letters of recommendation, preferably from university faculty and/or professional supervisors. We encourage you to submit the letters to the graduate school online as you complete the application process.
- A sample of scholarly writing, in English. This can be anything you've written but should give an accurate indication of your writing abilities. The writing sample can be a term paper, research report, journal article, published paper, college paper, etc.
- A completed Application for Graduate Assistantship , if you are interested in receiving funding.
- A Statement of Purpose.
- A Resume or Curriculum Vitae.
Further information on program admissions is found in the AI Institute Frequently Asked Questions (FAQ) .
International Students should also review the links on the Information for International Students page for additional information relevant to the application process.
Graduate School Policies
University of Georgia Graduate School policies and requirements apply in addition to (and, in cases of conflict, take precedence over) those described here. It is essential that graduate students familiarize themselves with Graduate School policies, including minimum and continuous enrollment and other policies contained in the Graduate School Bulletin.
Students should also familiarize themselves with Graduate School Dates and Deadlines relevant to the degree.
Degree Requirements
Students of the doctoral program must complete a minimum of 40 hours of graduate coursework and 6 hours of dissertation credit (for a total of 46 credit hours), pass a comprehensive examination, and write and defend a dissertation. In addition, the University requires that all first-year graduate students enroll in a 1-credit-hour GradFirst seminar . Each of these requirements is described in greater detail below.
The degree program is offered using an in-person format, and classes are in general scheduled for full-time students. There are currently no special provisions for part-time, online, or off-campus students. Students are expected to attend all meetings of classes for which they are registered.
Program of Study
The Program of Study must include a minimum of 40 hours of graduate course work and a minimum of 6 hours of dissertation credit. Of the 40 hours of graduate course work, at least 20 hours must be 8000-level or 9000-level hours.
Required Courses
The following courses must be completed unless specifically waived for students entering the program with a master’s degree in Artificial Intelligence or a related field, or for students with substantially related graduate course work. All waived credits may be replaced by an equal number of doctoral research or doctoral dissertation credits (ARTI 9000, Doctoral Research or ARTI 9300, Doctoral Dissertation). Substitutions must be approved for a particular student by that student's Advisory Committee and by the Graduate Coordinator.
- PHIL/LING 6510 Deductive Systems (3 hours)
- CSCI 6380 Data Mining (4 hours) or CSCI 8950 Machine Learning (4 hours)
- CSCI/PHIL 6550 Artificial Intelligence (3 hours)
- ARTI 6950 Faculty Research Seminar (1 hour)
- ARTI/PHIL 6340 Ethics and Artificial Intelligence (3 hours)
Elective Courses
In addition to the required courses above, at least 6 additional courses must be taken from Groups A and Group B below, subject to the following requirements.
- At least 2 courses must be taken from Group A, from at least 2 areas.
- At least 2 courses must be taken from Group B, from at least 2 areas.
- At least 3 courses must be taken from a single area comprising the student’s chosen area of emphasis .
Since not all courses have the same number of credit hours, Ph.D. students may need to take additional graduate courses to complete the 40 hours.
AREA 1: Artificial Intelligence Methodologies
- CSCI 6560 Evolutionary Computing (4 hours)
- CSCI 8050 Knowledge Based Systems (4 hours)
- CSCI/PHIL 8650 Logic and Logic Programming (4 hours)
- CSCI 8920 Decision Making Under Uncertainty (4 hours)
- CSCI/ENGR 8940 Computational Intelligence (4 hours)
- CSCI/ARTI 8950 Machine Learning (4 hours)
AREA 2: Machine Learning and Data Science
- CSCI 6360 Data Science II (4 hours)
- CSCI 8360 Data Science Practicum (4 hours)
- CSCI 8945 Advanced Representation Learning (4 hours)
- CSCI 8955 Advanced Data Analytics (4 hours)
- CSCI 8960 Privacy-Preserving Data Analysis (4 hours)
AREA 3: Machine Vision and Robotics
- CSCI/ARTI 6530 Introduction to Robotics (4 hours)
- CSCI 6800 Human Computer Interaction (4 hours)
- CSCI 6850 Biomedical Image Analysis (4 hours)
- CSCI 8850 Advanced Biomedical Image Analysis (4 hours)
- CSCI 8820 Computer Vision and Pattern Recognition (4 hours)
- CSCI 8530 Advanced Topics in Robotics (4 hours)
- CSCI 8535 Multi Robot Systems (4 hours)
AREA 4: Cognitive Modeling and Logic
- PHIL/LING 6300 Philosophy of Language (3 hours)
- PHIL 6310 Philosophy of Mind (3 hours)
- PHIL/LING 6520 Model Theory (3 hours)
- PHIL 8310 Seminar in Philosophy of Mind (max of 3 hours)
- PHIL 8500 Seminar in Problems of Logic (max of 3 hours)
- PHIL 8600 Seminar in Metaphysics (max of 3 hours)
- PHIL 8610 Epistemology (max of 3 hours)
- PSYC 6100 Cognitive Psychology (3 hours)
- PSYC 8240 Judgment and Decision Making (3 hours)
- CSCI 6860 Computational Neuroscience (4 hours)
AREA 5: Language and Computation
- ENGL 6885 Introduction to Humanities Computing (3 hours)
- LING 6021 Phonetics and Phonology (3 hours)
- LING 6080 Language and Complex Systems (3 hours)
- LING 6570 Natural Language Processing (3 hours)
- LING 8150 Generative Syntax (3 hours)
- LING 8580 Seminar in Computational Linguistics (3 hours)
AREA 6: Artificial Intelligence Applications
- ELEE 6280 Introduction to Robotics Engineering (3 hours)
- ENGL 6826 Style: Language, Genre, Cognition (3 hours)
- ENGL/LING 6885 Introduction to Humanities Computing (3 hours)
- FORS 8450 Advanced Forest Planning and Harvest Scheduling (3 hours)
- INFO 8000 Foundations of Informatics for Research and Practice
- MIST 7770 Business Intelligence (3 hours)
Students may under special circumstances use up to 6 hours from the following list to apply towards the Electives group requirement.
- ARTI 8800 Directed Readings in Artificial Intelligence
- ARTI 8000 Topics in Artificial Intelligence
Other courses may be substituted for those on the Electives lists, provided the subject matter of the course is sufficiently related to artificial intelligence and consistent with the educational objectives of the Ph.D. degree program. Substitutions can be made only with the permission of the student's Advisory Committee and the Graduate Coordinator.
In addition to the specific PhD program requirements, all first-year UGA graduate students must enroll in a 1 credit-hour GRSC 7001 (GradFIRST) seminar which provides foundational training in research, scholarship, and professional development. Students may enroll in a section offered by any department, but it is recommended that they enroll in a section offered by AI Faculty Fellows for AI students. More information is available at the Graduate School website .
Core Competency
Core competency must be exhibited by each student and certified by the student’s advisory committee. This takes the form of achievement in the required courses of the curriculum. Students entering the Ph.D. program with a previous graduate degree sufficient to cover this basic knowledge will need to work with their advisory committee to certify their core competency. Students entering the Ph.D. program without sufficient graduate background to certify core competency must take at least three of the required courses, and then pursue certification with their advisory committee. A grade average of at least 3.56 (e.g., A-, A-, B+) must be achieved for three required courses (excluding ARTI 6950). Students below this average may take the fourth required course and achieve a grade average of at least 3.32 (e.g., A-, B+, B+, B).
Core competency is certified by the unanimous approval of the student's Advisory Committee as well as the approval by the Graduate Coordinator. Students are strongly encouraged to meet the core competency requirement within their first three enrolled academic semesters (excluding summer semester). Core Competency Certification must be completed before approval of the Final Program of Study.
Comprehensive Examination
Each student of the doctoral program must pass a Ph.D. Comprehensive Examination covering the student's advanced coursework. The examination consists of a written part and an oral part. Students have at most two attempts to pass the written part. The oral part may not be attempted unless the written part has been passed.
Admission to Candidacy
The student is responsible for initiating an application for admission to candidacy once all requirements, except the dissertation prospectus and the dissertation, have been completed.
Dissertation and Dissertation Credit Hours
In addition to the coursework and comprehensive examination, every student must conduct research in artificial intelligence under the direction of an advisory committee and report the results of his or her research in a dissertation acceptable to the Graduate School. The dissertation must represent originality in research, independent thinking, scholarly ability, and technical mastery of a field of study. The dissertation must also demonstrate competent style and organization. While working on his/her dissertation, the student must enroll for a minimum of 6 credit hours of ARTI 9300 Doctoral Dissertation spread over at least 2 semesters.
Advisory Committee
Before the end of the third semester, each student admitted into the program should approach relevant faculty members and form an advisory committee. Until the committee is formed, the student will be advised by the graduate coordinator. The committee consists of a major professor and two other faculty members, as follows:
- The major professor and at least one other member must be full members of the Graduate Program Faculty.
- The major professor and at least one other member must be Institute for Artificial Intelligence Faculty Fellows.
Deviations from the 3-member advisory committee structure, including having more members, are in some cases permitted but must conform to Graduate School policies.
The major professor and advisory committee shall guide the student in planning the dissertation. The committee shall agree upon, document, and communicate expectations for the dissertation. These expectations may include publication or submission requirements, but, should not exceed reasonable expectations for the given research domain. During the planning stage, the student will prepare a dissertation prospectus in the form of a detailed written dissertation proposal. It should clearly define the problem to be addressed, critique the current state-of-the-art, and explain the contributions to research expected by the dissertation work. When the major professor certifies that the dissertation prospectus is satisfactory, it must be formally considered by the advisory committee in a meeting with the student. This formal consideration may not take the place of the comprehensive oral examination.
Approval of the dissertation prospectus signifies that members of the advisory committee believe that it proposes a satisfactory research study. Approval of the prospectus requires the agreement of the advisory committee with no more than one dissenting vote as evidenced by their signing an appropriate form to be filed with the graduate coordinator’s office.
Graduation Requirements - Forms and Timeline
Before the end of the third semester in residence, a student must begin submitting to the Graduate School, through the graduate coordinator, the following forms: (i) a Preliminary Program of Study Form and (ii) an Advisory Committee Form. The Program of Study Form indicates how and when degree requirements will be met and must be formulated in consultation with the student's major professor. An Application for Graduation Form must also be submitted directly to the Graduate School. Forms and Timing must be submitted as follows:
- Advisory Committee Form (G130)—end of third semester
- Core Competency Form (Internal to IAI)—beginning of fourth semester
- Preliminary Doctoral Program of Study Form—Fourth semester
- Final Program of Study Form (G138)—before Comprehensive Examination
- Application for Admission to Candidacy (G162)—after Comprehensive Examination
- Application for Graduation Form (on Athena)—beginning of last semester
- Approval Form for Doctoral Dissertation (G164)—last semester
- ETD Submission Approval Form (G129)—last semester
Students should frequently check the Graduate School Dates and Deadlines webpage to ensure that all necessary forms are completed in a timely manner.
Student Handbook
Additional information on degree requirements and AI Institute policies can be found in the AI Student Handbook .
For information regarding the graduate programs in IAI, please contact:
Evette Dunbar [email protected] Boyd GSRC, Room 516 706-542-0358
We appreciate your financial support. Your gift is important to us and helps support critical opportunities for students and faculty alike, including lectures, travel support, and any number of educational events that augment the classroom experience. Click here to learn more about giving .
Every dollar given has a direct impact upon our students and faculty.
Email forwarding for @cs.stanford.edu is changing. Updates and details here .
PhD Admissions
Main navigation.
The Computer Science Department PhD program is a top-ranked research-oriented program, typically completed in 5-6 years. There are very few course requirements and the emphasis is on preparation for a career in Computer Science research.
Eligibility
To be eligible for admission in a Stanford graduate program, applicants must meet:
- Applicants from institutions outside of the United States must hold the equivalent of a United States Bachelor's degree from a college or University of recognized good standing. See detailed information by region on Stanford Graduate Admissions website.
- Area of undergraduate study . While we do not require a specific undergraduate coursework, it is important that applicants have strong quantitative and analytical skills; a Bachelor's degree in Computer Science is not required.
Any questions about the admissions eligibility should be directed to [email protected] .
Application Checklist
An completed online application must be submitted by the CS Department application deadline and can be found here .
Application Deadlines
The online application can be found here and we will only one admissions cycle for the PhD program per respective academic term.

- [email protected]
- +91-97 91 62 64 69
PhD Research Proposal Artificial Intelligence
One of the most important subject areas of computer science is Artificial Intelligence. It provides a wide platform for building a machine with learning capabilities . Artificial intelligence makes machines think and react similarly to humans in uncertain situations. In other words, this machine intelligence to behave artificially like human intelligence is known as artificial intelligence. This page is intended to present you useful information on PhD Research Proposal Artificial Intelligence along with the latest research areas, technologies, challenges, trends, techniques, and ideas !!!
Assume that there is a situation in which a human is performing a particular task by learning and understanding the event to solve associated problems. This human task is performed by machine artificial learning abilities are known as artificial intelligence.
For instance: a self-driving car without a driver. In this, the vehicle monitors the environment and takes effective decisions for secure destination attainment.
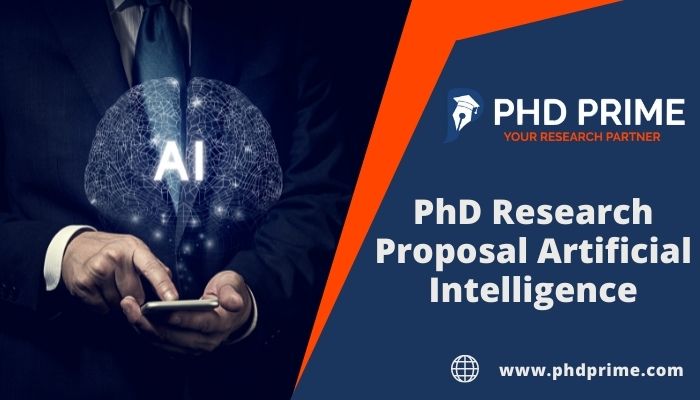
What are the requirements for good research proposal writing?
We believe that we made you are clear with the exact purpose and importance of artificial intelligence at this moment. Now, we can see about the requirements of good PhD Research Proposal Artificial Intelligence . Basically, the writing of PhD proposal needs more concern and study to create a qualified proposal. Since it is the reflection of your research activities and efforts in the form of valuable words. Here, we have given you a few important tips to prepare good proposal writing.
- Need to be adaptable to access required information and resources
- Need to be meet the expected standard and enhance interest to read
- Need to be original to create a new contribution to the handpicked research area
- Need to be related with your degree and present research areas of artificial intelligence
In general, the PhD research proposal has a standard format to write. As well, it is composed of different components such as title, abstract, introduction, literature study, methodologies, conclusion, and references. In fact, we have a native writer team to give complete assistance in perfect proposal writing. Further, we also help you in literature review writing, paper writing, and thesis writing. Here, we have given you a few important things that need to be focused on while writing PhD Research Proposal Artificial Intelligence.
What are the Components of a Good Research Proposal?
- Give a short and crisp title for your research proposal
- Choose a title that addresses your research problem and proposed solutions
- Provide a summary of your research work
- Act as detailed synopsis that answers why, how, and what questions of your research
- Present your selected research area and research problem(s)
- Highlight the significance of your study
- Provide sufficient hypothesis of research
- Mention the methodologies that going to be used as solutions
- Talk about the review of secondary research materials
- Address the identified research gaps in previous related studies
- Do a comparison of techniques and arguments in existing researches
- Describe the contribution and findings of the previous research
- List the merits and demerits of existing research works
- Present system architectural design
- Give a detailed explanation on used research tools and techniques/methodologies
- Speak about the need and importance of choosing those methodologies
- Explain the numerical formulas and used algorithms
- Give justification for your proposed research methodologies
- Mention in what way your research methodologies solve your research problem
- Again give an overview of your research
- Point out the objectives and importance of your research
- Encapsulate all highlights of your research in brief
- A present unique point of your study
- Overall, write nearly two paragraphs
- Provide citation of your referred research websites and books
- Implicitly these references mention your supportive hypothesis
- Narrow down your wide research sources
- Smart picking of research materials will impress the research committee
We hope that you are clear with the fundamentals of writing a good PhD research proposal artificial intelligence . Now, we can see about the three primary research terms of artificial intelligence. Since these terms are most widely used in many research areas of artificial intelligence. As well, it is categorized into three classifications such as,
- Exploration Areas
- Real-Time Applications
Our researchers are good at proposing modern research work in upcoming research areas for smart applications . If you are interested to know more research ideas from the following classifications, then make an online or offline connection with us.
What are three important terminologies in Artificial Intelligence?
- Genetic Evolutionary
- Logical Rationalism
- Molecular Biological
- Statistical Empiricism
- Neural Connectionism
- Smart System Design
- Learning Approaches
- Inference Mechanism
- Knowledge Representation
- Expert System
- Electronic Commerce
- Bioinformatics
- Intelligent Robots
- Natural Language Processing
- Information Retrieval
- Data Mining
In addition, we have also given you some significant research areas of artificial intelligence . We assure you that all these areas are recognized in current AI research topics and ideas.
Moreover, we also support you in other important research ideas to support you in all aspects of artificial intelligence . By the by, our first and foremost task in AI research is identifying your interesting research area. Then, we provide you list of the latest research notions and phd topics in artificial intelligence .
Research Areas for PhD Research Proposal Artificial Intelligence
- Reinforcement Learning
- Supervised Learning
- Unsupervised Learning
- Dialogue Systems
- Natural Language
- Understanding
- Recognition
- Classification
Furthermore, we have given you a few important supporting AI technologies. Due to the beneficial impact of AI, it is employed and demanding in several research fields (i.e., other technologies). For your information, here we have given you only a few of them. Once you connect with us, we let you know more about up-to-date research topics of your selected technologies . Specifically, these technologies are currently successful in creating real-time AI applications for the development of a smart society.
Converging Technologies of AI
- Internet of Things
- Big Data Analytics
- Blockchain Technology
- Lightweight Cryptography
- Cloud Computing
- Software-Defined Networking
- Fog Computing
- 6G Networks
- Industry 4.0
- UAV Communication
- Autonomous Vehicles
- Edge Networks
As a point of fact, AI is treated as the shared technology which used to solve different problems in different technologies. So, it can be recognized in many real-time applications and services. Although this field has so many developments in real-time applications, it has some technical issues that arise in the time of development and deployment . For your reference, here we have listed a few important technical issues of AI in recent research.
Artificial Intelligence Research Problems
- Optimized Modern Parameters
- Non-linearity from learning to compensate
- Hard-to-Model Issues
- Knowledge and Learning Representation
- Solution for Computational Infeasibility
- Computationally Understanding Solutions
- Training Policies
Already, we have seen converging technologies of artificial intelligence in an earlier section. To the continuation, now we can see about the current trends of AI. In order to identify these trends, our research team has studied the present and past 2-3 years’ research articles and magazines. Through this review, we analyzed and identified
1) Research gaps that need to address
2) Problems that need enhanced solutions than existing one
From this collection, we have listed only a few of them for your reference. Further, we are also ready to share more trends that are sought by active research scholars in the field of artificial intelligence.
Artificial Intelligence Current Trends
- Mainly in sustainable developments, energy usage has a key player role
- Provides productive communication plans for improving energy-efficiency
- Support significant services in 6G communication
- Human-sensed data are composed with 5D services to enhance the holographic communication
- Assure high QoS, precision, deterministic in 6G communication
- Need tremendous data rates like Tb/s
- Currently, manufacturing industries are moving towards automation technologies and precision communication
- In this, 6G is assured to give ultra-low delay and ultra-high reliability
- For real-cases, the general data transmission need industrial networks for low latency jitters
- For achieving a secure environ, wireless technologies, IoT and fog-cloud computing are advancing over global sustainability and QoS
- Presently, the 6G network understands 3D communication to enhance several applications like smart transportation, smart cities, smart healthcare, etc.
- For instance – Self-driving vehicles delay < 1ms and reliability > 99.999% for fast decisions over sudden accidents
Now, we can see emerging techniques that play a major role in bringing effective research solutions for different current research problems. As a matter of fact, our developers are proficient-enough to identify the best-fitting research techniques and algorithms for any sort of research problem .
In the case of complications in solving problems, our developers analyze the degree of problem complexity and create hybrid technologies or new algorithms accordingly. Overall, we are good to tackle the problem at any level of complexity in smart ways. Also, we suggest key parameters and development tools that enhance your system performance.
Latest Techniques in AI
- Generally, the data are collected from different formats, mode representations and sources
- Merging all these dissimilar data in one place is a tedious task
- For the data fusion, advanced neural networks and bayesian learning is used
- For instance – CNN, RBM, and DBM
- Through sensors, collect raw data and transfer it into high-computational devices for data processing
- This may cause more power usage and high traffic load over the network
- So, it is required to design a system that minimizes load and power usage without losing vital information
- Utilize ANN and perform preprocessing
- Also, network topology and architecture are required to be chosen appropriately for add-on benefits
- Prevent interference for primary user benefits through spectrum sensing
- The significant role of the primary user is to transmit data between secondary users and the succeeding layer
- This process is executed by Cooperative Spectrum Sensing (CSS) with high power usage
- The power usage increases because of report findings and spectrum sensing with respect to a centralized location
- Similarly, Convolutional Neural Network is utilized in Deep Corporate Sensing
Additionally, we have given you some growing ideas about artificial intelligence. These ideas are selected from different trending research areas that gain more attraction from the research community. If you have your own ideas to implement an artificial intelligence project, then we support you to upgrade your idea to match the latest advancements of artificial intelligence. So, create a bond with us, to know new interesting PhD research propsoal artificial intelligence . Overall, we give assistance on not only these ideas but also beyond this list of ideas.
Emerging Ideas on AI
- Artificial Intelligence for Internet of Things
- Privacy-Aware AI-assisted Edge System for Trustable Services
- Fast AI Services Migration from Cloud into Edge
- Secure Data Dissemination on AI-assisted Edge Systems
- In-depth Learning Services over Edge Network
- Energy-Aware AI-assisted Edge System for Quality of Services
- Real-time AI-assisted Edge Systems with Optimized Solutions
- Edge-intensive Distributed / Collaborate / Federated Smart Services
On the whole, we are here to update you about the recent research updates of artificial intelligence in every possible area. Particularly, we help you in research problem selection, corresponding solutions selection, PhD Research Proposal Artificial Intelligence Writing, code development, paper writing, paper publication, and thesis writing. So, think smartly and hold your hands with our technical experts to shine your AI research career.

Opening Hours
- Mon-Sat 09.00 am – 6.30 pm
- Lunch Time 12.30 pm – 01.30 pm
- Break Time 04.00 pm – 04.30 pm
- 18 years service excellence
- 40+ country reach
- 36+ university mou
- 194+ college mou
- 6000+ happy customers
- 100+ employees
- 240+ writers
- 60+ developers
- 45+ researchers
- 540+ Journal tieup
Payment Options

Our Clients

Social Links

- Terms of Use

Opening Time
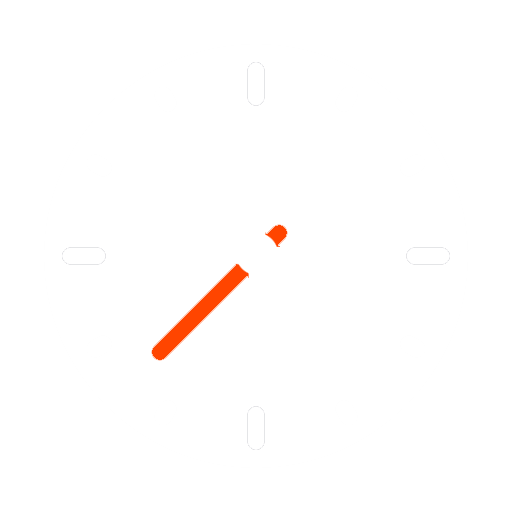
Closing Time
- We follow Indian time zone

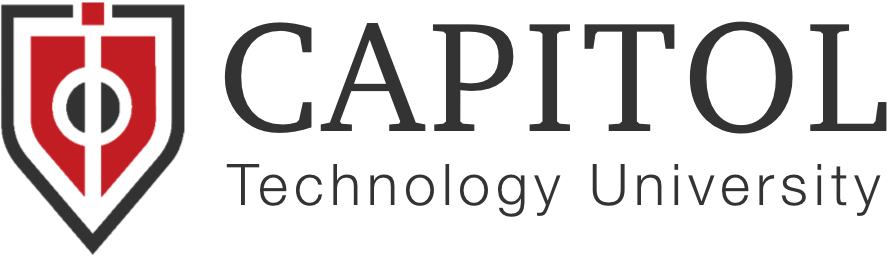
- Aviation and Astronautical Sciences
- Computer Science, Artificial Intelligence and Data Science
- Construction and Facilities
- Critical Infrastructure
- Cyber & Information Security
- Cyberpsychology
- Engineering
- Engineering Technologies
- Intelligence and Global Security Studies
- Management of Technology
- Occupational Safety and Health
- Uncrewed Systems
- Doctoral Degrees
- Master's Degrees
- Bachelor's Degrees
- Online Programs
- Associate Degrees
- Certificates
- Minor Degrees
- STEM Events
- Webinars and Podcasts
- Master's
- Undergraduate
- Transfer Students
- Military and Veterans
- International Students
- Admissions Counselor
- Capitol Connections
- Accepted Students
- Project Lead the Way
- Builder Culture
- Campus Life
- Clubs and Organizations
- Centers and Labs
- Online Classes
- The Capitol Commitment
- Top Employers
- Co-ops and Internships
- Professional Education
- Find a Mentor
- Career Services
- Capitol Online Job Board
- Recruiters and Employers
- Why Capitol Tech
- At a Glance
- Mission, Vision and Goals
- Diversity, Equity and Inclusion
- Washington, D.C.
- Capitol History
- Capitol Partners
- News and Events
- Visitors/Campus
- Accreditation
- Recognitions & Awards
- Current Students
- Faculty & Staff
- Alumni & Giving
- News & Events
- Capitology Blog
- Maps / Directions

- Degrees and Programs
Doctor of Philosophy (PhD) in Artificial Intelligence
- Request Information

Earn a doctorate degree in Artificial Intelligence, help lead innovation in a growing industry
The PhD in Artificial Intelligence is centered upon how computers operate to match the human decision making process in the brain. Your research will be led by AI experts with both research and industrial expertise. This emerging subject is starting to attract attention on the wider issues as the IOT and other advanced computer systems work in our lives.
This is a research based doctorate PhD degree where you will be assigned an academic supervisor almost immediately to guide you through your program and is based on mostly independent study through the entire program. It typically takes a minimum of two years but typically three years to complete if a student works closely with their assigned academic advisor. Under the guidance of your academic supervisor, you will conduct unique research in your chosen field before submitting a Thesis or being published in three academic journals agreed to by the academic supervisor. If by publication route it will require original contribution to knowledge or understanding in the field you are investigating.
As your PhD progresses, you move through a series of progression points and review stages by your academic supervisor. This ensures that you are engaged in a process of research that will lead to the production of a high-quality Thesis and/or publications and that you are on track to complete this in the time available. Following submission of your PhD Thesis or accepted three academic journal articles, you have an oral presentation assessed by an external expert in your field.
Why Capitol?
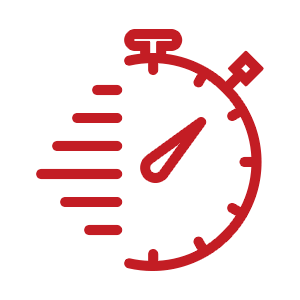
Learn around your busy schedule
Program is 100% online, with no on-campus classes or residencies required, allowing you the flexibility needed to balance your studies and career.
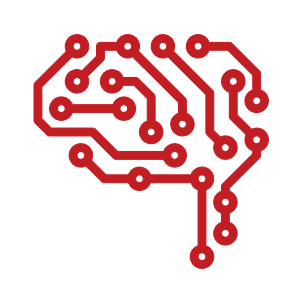
Proven academic excellence
Study at a university that specializes in industry-focused education in technology fields, with a faculty that includes many industrial and academic experts.
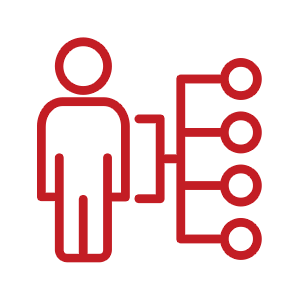
Expert guidance in doctoral research
Capitol’s doctoral programs are supervised by faculty with extensive experience in chairing doctoral dissertations and mentoring students as they launch their academic careers. You’ll receive the guidance you need to successfully complete your doctoral research project and build credentials in the field.
Key Faculty
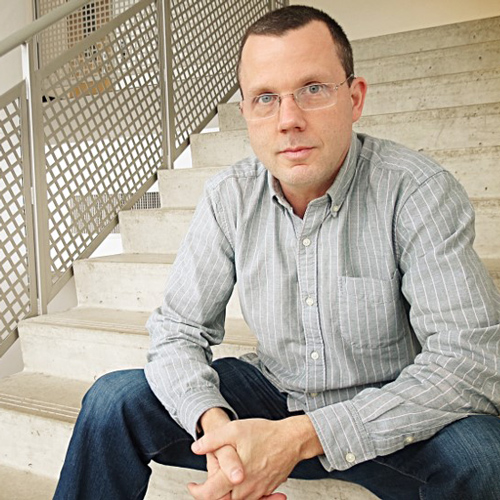
Dissertation Chair
Degree Details
This program may be completed with a minimum of 60 credit hours, but may require additional credit hours, depending on the time required to complete the dissertation/publication research. Students who are not prepared to defend after completion of the 60 credits will be required to enroll in RSC-899, a one-credit, eight-week continuation course. Students are required to be continuously enrolled/registered in the RSC-899 course until they successfully complete their dissertation defense/exegesis.
The student will produce, present, and defend a doctoral dissertation after receiving the required approvals from the student’s Committee and the PhD Review Boards.
Prior Achieved Credits May Be Accepted
Doctor of Philosophy - 60 credits
(Prerequisite: None) | 6 |
(Prerequisite: AIT-800) | 6 |
(Prerequisite: AIT-810) | 6 |
(Prerequisite: AIT-820) | 6 |
(Prerequisite: AIT-830) | 6 |
(Prerequisite: AIT-840) | 6 |
(Prerequisite: AIT-900) | 6 |
(Prerequisite: AIT-910) | 6 |
(Prerequisite: AIT-920) | 6 |
(Prerequisite: AIT-930) | 6 |
Program Objectives:
- Students will integrate and synthesize alternate, divergent, or contradictory perspectives or ideas fully within the field of Artificial Intelligence.
- Students will demonstrate advance knowledge and competencies in Artificial Intelligence.
- Students will analyze existing theories to draw data-supported consultations in Artificial Intelligence.
- Students will analyze theories, tools, and frameworks used in Artificial Intelligence.
- Students will execute a plan to complete a significant piece of scholarly work in Artificial Intelligence.
- Students will evaluate the legal, social, economic, environmental, and ethical impact of actions within Artificial Intelligence and demonstrate advance skill in integrating the results in to the leadership decision-making process.
Learning Outcomes:
Upon graduation, graduates will:
- integrate the theoretical basis and practical applications on Artificial Intelligence in to their professional work;
- demonstrate the highest mastery of Artificial Intelligence;
- evaluate complex problems, synthesize divergent/alternative/contradictory perspectives and ideas fully, and develop advanced solutions to Artificial Intelligence challenges; and
- contribute to the body of knowledge in the study of Artificial Intelligence.
Tuition & Fees
Tuition rates are subject to change.
The following rates are in effect for the 2024-2025 academic year, beginning in Fall 2024 and continuing through Summer 2025:
- The application fee is $100
- The per-credit charge for doctorate courses is $950. This is the same for in-state and out-of-state students.
- Retired military receive a $50 per credit hour tuition discount
- Active duty military receive a $100 per credit hour tuition discount for doctorate level coursework.
- Information technology fee $40 per credit hour.
- High School and Community College full-time faculty and full-time staff receive a 20% discount on tuition for doctoral programs.
Find additional information for 2024-2025 doctorate tuition and fees.
When I was comparing multiple universities, Capitol Tech's admissions staff was responsive, helpful, and caring. This was valuable my deciding factor in choosing Capitol Tech.
-Dr. Jason Collins-Baker PhD in Artificial Intelligence
I chose Capitol for its great reputation in the field I am interested in, as well as its flexibility offering a fully Online program that works very well with my work and family commitments. Another reason was its European PhD program, with which I am familiar since I grew up and studied in Europe (Spain), although I currently live in the United States (California).
-Hector Garcia Villa PhD in Artificial Intelligence
Need more info, or ready to apply?
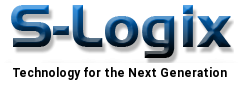
Office Address
- 2nd Floor, #7a, High School Road, Secretariat Colony Ambattur, Chennai-600053 (Landmark: SRM School) Tamil Nadu, India
- [email protected]
- +91- 81240 01111
Social List
Latest research proposal in artificial intelligence (ai).

Best Masters and PhD Research Proposal in Artificial Intelligence (AI)
Nowadays, people live their life with more advancements and ease of access to the developed technologies. Artificial intelligence is the process of mimicking behaviors, or functions of the human brain by the computes or machines in problem-solving and learning information. The advanced artificial intelligence model greatly assists computer vision, natural language processing, prediction, and decision-making functions in various real-world application domains. With the real-life successes in machine learning, artificial intelligence has gained enormous attention among people. Owing to the advances in artificial intelligence, the applications of self-driving cars, smart speakers, image recognition, and computer vision have been assisted day-to-day human lives. With the rise of big data, artificial intelligence plays a significant role in public life and the business environment. Spam classification, handwritten digits recognition, medical decision-making, gaming, opinion mining, next-word prediction, chatbot, fake review recognition, automatic attendance, and music recommendation are the rapidly emerging artificial intelligence research areas.
- Guidelines for Preparing a Phd Research Proposal
Latest Research Proposal Ideas in Artificial Intelligence
- Research Proposal on AI for air quality monitoring and control in smart cities
- Research Proposal on Machine learning for drug efficacy and safety prediction in clinical trials using AI
- Research Proposal on Natural language processing for student modeling in AI
- Research Proposal on Explainable Scene Understanding and Context-Aware Vision for AI
- Research Proposal on Multi-Agent System Security and Privacy in Decentralized Systems for AI
- Research Proposal on AI-based Algorithmic Game Theory for Multi-Agent Systems
- Research Proposal on Aligning AI with Human Values
- Research Proposal on Privacy-Preserving Multi-Agent Communication Protocols for AI
- Research Proposal on Hierarchical Planning and Scheduling for AI
- Research Proposal on Knowledge representation and reasoning for educational systems in AI
- Research Proposal on Design and evaluation of educational robotics curricula with AI
- Research Proposal on Visualization of Neural Network Decisions for AI
- Research Proposal on AI Regulation and Policy
- Research Proposal in AI for Context-Aware Machine Learning
- Research Proposal on Privacy and Confidentiality in AI
- Research Proposal on Decentralized Multi-Agent Control in AI
- Research Proposal on Multi-Agent Communication and Coordination in Distributed Systems for AI
- Research Proposal on Game-Theoretic Approaches for Multi-Agent Communication in AI
- Research Proposal on User-Centered Explanations in Recommender Systems for AI
- Research Proposal on Explainable Fraud Detection with AI
- Research Proposal on Reinforcement Learning for Autonomous Vehicle Energy Management in AI
- Research Proposal on Visual Explanations for Explainable Anomaly Detection in AI
- Research Proposal on Deep Learning for Computer Vision with AI
- Research Proposal on Transparent and Explainable AI
- Research Proposal on Multi-Agent Communication and Coordination in Decentralized Systems for AI
- Research Proposal on Deep Generative Models for Image Restoration and Enhancement in AI
- Research Proposal on Reinforcement Learning for Autonomous Vehicles using AI
- Research Proposal on User-Centered Interpretable Machine Learning for AI
- Research Proposal on Contextual Reasoning in Explainable AI
- Research Proposal on Bias and Fairness in AI
- Research Proposal on AI-based Computational Game Theory for Multi-Agent Systems
- Research Proposal on Visual Explanations for Deep Learning Models in AI
- Research Proposal on Counterfactual Explanations in Explainable AI
- Research Proposal on Weakly Supervised Learning for Computer Vision in AI
- Research Proposal on Multi-Agent System Privacy Threats and Attacks in AI
- Research Proposal on Natural language processing for smart city citizen engagement with AI
- Research Proposal on AI-based drug discovery and target prediction for cancer treatment
- Research Proposal on AI for resource-constrained devices in IoT
- Research Proposal on Reinforcement learning for treatment recommendation with AI
- Research Proposal on Predictive analytics for clinical trial recruitment and retention with AI
- Research Proposal on Fraud Detection and Waste Reduction in Healthcare Management for AI
- Research Proposal on AI-based fraud detection in business operations
- Research Proposal on AI-based Predictive modeling for personalized medicine
- Research Proposal in AI for Impact of educational robotics on student motivation and engagement
- Research Proposal on Real-time Planning and Scheduling for Robotics and Automation in AI
- Research Proposal on Real-Time Image and Video Pattern Matching for AI
- Research Proposal on Nash Equilibrium in Multi-Agent Systems for AI
- Research Proposal on Deep learning for early disease diagnosis and prediction in AI
- Research Proposal on Bayesian deep learning for uncertainty modeling in AI
- Research Proposal on Machine learning for clinical trial design and optimization in AI
- Research Proposal on Measuring and evaluating the impact and ROI of AI investments in business
- Research Proposal on Personalized learning recommendation systems for AI
- Research Proposal on Deep Reinforcement Learning for Planning and Scheduling with AI
- Research Proposal on AI-based Integration of educational robotics in the classroom
- Research Proposal on AI-enabled student performance assessment and analysis
- Research Proposal on Chatbots and Virtual Assistants for Patient Support with AI
- Research Proposal on AI-powered traffic management in smart cities
- Research Proposal on Reinforcement Learning for Autonomous Vehicle Trajectory Prediction in AI
- Research Proposal on Explainable Risk Assessment with AI
- Research Proposal on Transfer Learning for Context-Aware Vision in AI
- Research Proposal on Deep learning based models for automated essay grading in AI
- Research Proposal on Deep Learning for Medical Image Analysis using AI
- Research Proposal on Causal inference methods for medical decision making with AI
- Research Proposal on Multi-modal learning analytics for AI
- Research Proposal on Deep Learning for Human Pose Estimation in AI
- Research Proposal on Non-monotonic Reasoning for Defeasible Logics in AI
- Research Proposal on Explainable AI for IoT decision-making
- Research Proposal on Human-in-the-loop essay grading systems for AI
- Research Proposal on Formal Methods for Multi-Agent Communication Protocols in AI
- Research Proposal on Human-Centered Visual Explanations for AI
- Research Proposal on Personalized learning and Adaptive educational systems for AI
- Research Proposal on Multi-modal fusion for disease diagnosis and prognosis using AI
- Research Proposal on AI-enabled data analysis for improved clinical trial outcomes
- Research Proposal on Reinforcement Learning for Autonomous Vehicle Interaction with Other Agents using AI
- Research Proposal on AI-based prediction of disease progression and treatment response
- Research Proposal on AI-based device management and control in IoT
- Research Proposal on AI-powered patient stratification for personalized clinical trials
- Research Proposal on Ethical and privacy considerations in AI-powered classroom management
- Research Proposal on AI-based Reinforcement Learning for Treatment Planning
- Research Proposal on Personalized student learning using AI algorithms for AI
- Research Proposal on End-to-end Multi-modal Fusion for AI
- Research Proposal on Image Restoration and Enhancement for Low-Quality Images in AI
- Research Proposal on Multi-Agent System Security and Privacy in Multi-Agent Learning for AI
- Research Proposal on Game-Theoretic Approaches to Multi-Agent System Design in AI
- Research Proposal on Scalable Multi-Agent Reinforcement Learning in AI
- Research Proposal in AI for Scene Understanding and Context-Aware Vision
- Research Proposal on Non-monotonic Reasoning for Belief Revision in AI
- Research Proposal on Counterfactual Explanations for Credit Scoring with AI
- Research Proposal on Deep Reinforcement Learning for Autonomous Driving with AI
- Research Proposal on Deep Learning for Autonomous Vehicle Perception using AI
- Research Proposal on Human-Centered Explainable AI
- Research Proposal on Image Restoration and Enhancement for AI
- Research Proposal on Multi-Agent System Security and Privacy in Resource Constrained Systems for AI
- Research Proposal on AI-based Transfer Learning for Image Restoration and Enhancement
- Research Proposal on Planning and Scheduling with Constraints and Preferences for AI
- Research Proposal on Ensemble methods for enhanced cancer prediction and diagnosis using AI
- Research Proposal on Deep Learning for Autonomous Vehicles
- Research Proposal on Artificial intelligence for the integration of real-world data into clinical trial design
- Research Proposal on Image Super Resolution Using Deep Learning
- Research Proposal on AI-powered care pathway optimization and decision support
- Research Proposal on Action Recognition using Deep Learning
- Research Proposal on Multi-Agent Communication and Coordination in Multi-Agent Decision Making for AI
- Research Proposal on Human Motion Recognition using Deep Learning
- Research Proposal on International Cooperation on AI Governance
- Research Proposal on Object Detection using Deep Learning
- Research Proposal on Dropout as a method for uncertainty modeling in deep learning using AI
- Research Proposal on Image Segmentation using Deep Learning
- Research Proposal on Deep learning for early cancer diagnosis and prediction using AI
- Research Proposal on Knowledge Representation and Reasoning for AI
- Research Proposal on Predictive modeling for patient response to treatment in AI
- Research Proposal on Ethical issues of AI
- Research Proposal on Pattern Matching for AI
- Research Proposal on AI for supply chain optimization and management
- Research Proposal on Multi-Agent Systems for AI
- Research Proposal on Real-time student performance prediction with AI
- Research Proposal on Planning and Scheduling for AI
- Research Proposal on Multi-Agent Communication and Coordination in Multi-Agent Learning for AI
- Research Proposal on Multimodal Learning for Autonomous Driving
- Research Proposal on AI and Intellectual Property
- Research Proposal on Commonsense Reasoning
- Research Proposal on Human Pose Estimation using AI
- Research Proposal on Artificial Intelligence in Education
- Research Proposal on Non-monotonic Reasoning for Diagnosis and Debugging in AI
- Research Proposal on Artificial Intelligence in Agriculture
- Research Proposal on Planning and Scheduling under Uncertainty for AI
- Research Proposal on Artificial Intelligence in Business Management
- Research Proposal on Predictive models for educational policy and resource allocation in AI
- Research Proposal on Artificial Intelligence in Marketing
- Research Proposal on AI-based Machine learning for drug efficacy and safety prediction in clinical trials
- Research Proposal on Artificial Intelligence in Healthcare
- Research Proposal on AI-based Deep reinforcement learning for IoT network optimization
- Research Proposal on Artificial Intelligence in Internet of Things
- Research Proposal on Ensemble methods for improved disease prediction in AI
- Research Proposal on Uncertainty Modeling for AI
- Research Proposal on Development of pedagogical methods for learning with educational robots in AI
- Research Proposal on Explainable Artificial Intelligence
- Research Proposal on Adversarial Training for Image Restoration and Enhancement in AI
- Research Proposal on Non-monotonic Reasoning for Abduction in AI
- Research Proposal on Reinforcement Learning for Autonomous Vehicle Safety using AI
- Research Proposal on Multi-modal Sensor Fusion for Autonomous Vehicles with Deep Learning in AI
- Research Proposal on Contextual Reasoning for Healthcare with AI
- Research Proposal on AI and Human Rights
- Research Proposal on Multi-Agent System Security and Privacy in Dynamic Environments for AI
- Research Proposal on Transfer Learning for Image and Video Pattern Matching with AI
- Research Proposal on Human Pose Estimation from Single Images for AI
- Research Proposal on Predictive models for teacher effectiveness in AI
- Research Proposal in AI for Early detection of chronic diseases using machine learning
- Research Proposal on Predictive algorithms for clinical trial dropout prediction in AI
- Research Proposal on Uncertainty modeling for robust decision-making in AI
- Research Proposal on AI-powered personalized medicine for cancer treatment
- Research Proposal on AI-based Predictive modeling for resource allocation in healthcare
- Research Proposal on Representation learning for disease prediction in medical imaging using AI
- Research Proposal on Natural language processing for voice-controlled IoT devices using AI
- Research Proposal on Multi-Agent Coordination Mechanisms in AI
- Research Proposal on Trustworthy AI Systems
- Research Proposal on Scalable Multi-Agent System Architecture and Infrastructure in AI
- Research Proposal on Semi-Supervised and Weakly-Supervised Image and Video Pattern Matching for AI
- Research Proposal on Human Pose Estimation from Depth Data for AI
- Research Proposal on Multi-modal Deep Metric Learning for Autonomous Driving with AI
- Research Proposal on Multi-Agent Communication and Coordination in Resource Constrained Systems for AI
- Research Proposal on Contextual Reasoning for Predictive Maintenance with AI
- Research Proposal on Visual Explanations for Image Classification with AI
- Research Proposal on Deep Learning for Autonomous Vehicle Navigation in AI
- Research Proposal on AI for Uncertainty quantification in neural network interpretability
- Research Proposal on AI-powered student behavior analysis and prediction
- Research Proposal on Human-in-the-loop Multi-modal Perception for AI
- Research Proposal on Multi-Agent Communication and Coordination in Dynamic Environments for AI
- Research Proposal on AI-based predictive maintenance in IoT
- Research Proposal on Visual Explanations for Object Detection and Segmentation using AI
- Research Proposal in AI for Autonomous Vehicle Navigation with Reinforcement Learning
- Research Proposal on Counterfactual Explanations for Fairness and Bias in AI
- Research Proposal on AI and Competition Policy
- Research Proposal on Scalable Multi-Agent System Performance Evaluation in AI
- Research Proposal on Image and Video Pattern Matching in Complex Environments for AI
- Research Proposal on Multi-modal Imitation and Reinforcement Learning for AI
- Research Proposal on Predictive models for student engagement and motivation in AI
- Research Proposal on AI-powered teacher training and support
- Research Proposal on Natural Language Processing for Clinical Text in AI
- Research Proposal on Federated learning for privacy-preserving medical data analysis using AI
- Research Proposal on Deep uncertainty modeling in generative models for AI
- Research Proposal on AI-based Development of robotic tools for assessment and feedback in education
- Research Proposal on Predictive Health Analytics for AI
- Research Proposal on Ensemble methods for uncertainty modeling in AI
- Research Proposal on Machine learning for cancer prognosis and survival prediction in AI
- Research Proposal on Multi-Agent System Security and Privacy in Ad Hoc Networks for AI
- Research Proposal on The Impact of AI on Jobs and Employment
- Research Proposal on Contextual Reasoning for Fairness and Bias in AI
- Research Proposal on Counterfactual Explanations in Decision Making with AI
- Research Proposal on Autonomous Vehicle Control with Deep Learning for AI
- Research Proposal on Scalable Multi-Agent System Monitoring and Debugging in AI
- Research Proposal on Epistemic and aleatoric uncertainty in AI
- Research Proposal on AI-based Transfer learning for improved cancer prediction in under-resourced settings
- Research Proposal on Interactive Visual Explanations for AI
- Research Proposal on Reinforcement Learning for Autonomous Vehicle Control in AI
- Research Proposal on Contextual Reasoning in Decision Making with AI
- Research Proposal on AI for real-time monitoring and management of smart city resources
- Research Proposal on AI for security and privacy in IoT
- Research Proposal on AI-based student engagement monitoring and improvement
- Research Proposal on Artificial intelligence for real-time monitoring of adverse events in clinical trials
- Research Proposal on Model uncertainty in deep neural networks for AI
- Research Proposal on Representation learning in medical imaging for cancer prediction using AI
- Research Proposal on User-Centered Explanations for Deep Learning Models in AI
- Research Proposal on Transfer Learning for Computer Vision using AI
- Research Proposal on Scalable Multi-Agent System Deployment in AI
- Research Proposal on Multi-Agent Communication and Coordination in Ad Hoc Networks for AI
- Research Proposal on Robust Image and Video Pattern Matching under variations for AI
- Research Proposal on AI-based Scene Understanding and Context-Aware Vision for Robotics and Autonomous Systems
- Research Proposal on Student engagement and motivation for AI
- Research Proposal on AI-driven process automation in business operations
- Research Proposal in AI for customer behavior prediction and market trend analysis
- Research Proposal on Deep learning for imaging-based biomarker discovery in clinical trials using AI
- Research Proposal on AI-enabled Transfer learning for disease prediction in under-resourced settings
- Research Proposal on Uncertainty-aware reinforcement learning for AI
- Research Proposal on Deep learning-based image analysis for medical imaging diagnosis using AI
- Research Proposal in AI for enhancing HR processes
- Research Proposal on Game-based and gamified learning for AI
- Research Proposal on Fair and Ethical Decision Making with AI
- Research Proposal on Explainable Predictive Maintenance with AI
- Research Proposal on User-Centered Explanations for Fraud Detection with AI
- Research Proposal in AI for Object Detection and Segmentation
- Research Proposal on AI Risk Management
- Research Proposal on Scalable Multi-Agent System Design in AI
- Research Proposal on Real-time Human Pose Estimation for AI
- Research Proposal on Personalized feedback generation in automated essay grading with AI
- Research Proposal on Development of robotic platforms for STEM education with AI
- Research Proposal on Transfer learning for efficient deployment of AI models in IoT devices
- Research Proposal on Predictive modeling for personalized medicine in AI
- Research Proposal on AI-based Machine learning for clinical trial design and optimization
- Research Proposal on Ethics and bias in AI-powered business decisions
- Research Proposal on Contextual Explanations in Deep Learning for AI
- Research Proposal on Explainable AI for Trust and Confidence
- Research Proposal on Scalable Multi-Agent Decision Making in AI
- Research Proposal on Ethical AI and Governance
- Research Proposal on Mechanism Design for Multi-Agent Systems using AI
- Research Proposal on Content-Based Image and Video Retrieval for AI
- Research Proposal on Human Pose Estimation in Challenging Scenes for AI
- Research Proposal on Predictive models for student dropout and retention in AI
- Research Proposal on Automated classroom administration using AI
- Research Proposal in AI for content creation and digital advertising
- Research Proposal on AI-enabled drug discovery and development
- Research Proposal on Predictive modeling for patient response to treatment using AI
- Research Proposal on Natural language processing for electronic health records analysis using AI
- Research Proposal on Gaussian processes for uncertainty modeling in AI
- Research Proposal on Personalized public services using AI in smart cities
- Research Proposal on AI-based Multi-omics data integration for cancer prediction and diagnosis
- Research Proposal on Deep Learning for Autonomous Vehicle Safety using AI
- Research Proposal on Explainable Visual Analytics for AI
- Research Proposal on Counterfactual Explanations for Deep Learning Models using AI
- Research Proposal on User-Centered Explanations for Healthcare with AI
- Research Proposal on Human-centered AI for Trust
- Research Proposal on Scalable Multi-Agent Resource Allocation in AI
- Research Proposal on Multi-Agent System Security Threats and Attacks in AI
- Research Proposal on Multi-Person Pose Estimation for AI
- Research Proposal on Multi-modal Scene Graph Generation for AI
- Research Proposal on Stackelberg Equilibrium in Multi-Agent Systems for AI
- Research Proposal on Predictive modelling of cancer treatment response using AI
- Research Proposal in AI for personalized marketing and customer segmentation
- Research Proposal on AI-assisted grading and feedback generation
- Research Proposal on Handling multi-modal input in automated essay grading with AI
- Research Proposal on Multi-modal Generative Adversarial Networks for AI
- Research Proposal on Multi-Task Scene Understanding and Context-Aware Vision for AI
- Research Proposal on Multi-Agent System Security and Privacy in Distributed Systems for AI
- Research Proposal on Large-Scale Image and Video Pattern Matching for AI
- Research Proposal on Transfer Reinforcement Learning for Autonomous Vehicles in AI
- Research Proposal on Counterfactual Reasoning in Machine Learning using AI
- Research Proposal on User-Centered Explanations in Decision Making with AI
- Research Proposal on Contextual Reasoning in Recommender Systems for AI
- Research Proposal on Non-monotonic Logics for AI
- Research Proposal on Visual Explanations for Decision Making with AI
- Research Proposal on Deep Learning for Autonomous Vehicle Motion Planning with AI
- Research Proposal on Machine learning for personalized medicine and disease prediction in AI
- Research Proposal on Explainable AI for decision-making in cancer diagnosis and treatment using AI
- Research Proposal on Adversarial machine learning for robust medical AI systems
- Research Proposal on Predictive patient monitoring and triage for AI
- Research Proposal on Multi-modal learning with educational robots for AI
- Research Proposal on Multi-modal Attention Mechanisms for AI
- PhD Guidance and Support Enquiry
- Masters and PhD Project Enquiry
- PhD Research Guidance in Artificial Intelligence
- PhD Research Guidance in Machine Learning
- Research Topics in Artificial Intelligence
- Research Topics in Machine Learning
- PhD Research Proposal in Artificial Intelligence
- PhD Research Proposal in Machine Learning
- Latest Research Papers in Artificial Intelligence
- Latest Research Papers in Machine Learning
- Literature Survey in Artificial Intelligence
- Literature Survey in Machine Learning
- PhD Thesis in Artificial Intelligence
- PhD Thesis in Machine Learning
- PhD Projects in Artificial Intelligence
- PhD Projects in Machine Learning
- Leading Journals in Artificial Intelligence
- Leading Journals in Machine Learning
- Leading Research Books in Artificial Intelligence
- Leading Research Books in Machine Learning
- Research Topics in Federated Learning
- Research Topics in Medical Machine Learning
- Research Topics in Depression Detection based on Deep Learning
- Research Topics in Recent Advances in Deep Recurrent Neural Networks
- Research Topics in Multi-Objective Evolutionary Federated Learning
- Research Topics in Recommender Systems based on Deep Learning
- Research Topics in Computer Science
- PhD Thesis Writing Services in Computer Science
- PhD Paper Writing Services in Computer Science
- How to Write a PhD Research Proposal in Computer Science
- Ph.D Support Enquiry
- Project Enquiry
- Research Guidance in Artificial Intelligence
- Research Proposal in Artificial Intelligence
- Research Papers in Artificial Intelligence
- Ph.D Thesis in Artificial Intelligence
- Research Projects in Artificial Intelligence
- Project Titles in Artificial Intelligence
- Project Source Code in Artificial Intelligence
- Corpus ID: 209436745
PHD PROPOSAL IN ARTIFICIAL INTELLIGENCE AND MACHINE LEARNING
- Published 2019
- Computer Science
25 References
Large-scale machine learning and applications, a survey on transfer learning, distributed gaussian processes, deterministic execution on gpu architectures, exact gaussian processes on a million data points, a loewner-based approach for the approximation of engagement-related neurophysiological features, the worst-case execution-time problem—overview of methods and survey of tools, electrocardiogram generation with a bidirectional lstm-cnn generative adversarial network, pgans: personalized generative adversarial networks for ecg synthesis to improve patient-specific deep ecg classification, supplementary for: deep learning with convolutional neural networks for eeg decoding and visualization, related papers.
Showing 1 through 3 of 0 Related Papers
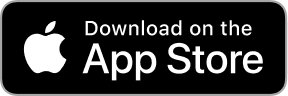
🤖 AI Research Proposal Generator
Seize the opportunity to revolutionize AI research with our AI Research Proposal Generator. Craft compelling and comprehensive proposals that will secure funding and set your project on the path to success.
Discover the power at your fingertips when you master the art of crafting a compelling research proposal. An indispensable communication tool for academics and professionals alike, a well-structured proposal paves the way for successful research journeys, enabling innovative theories, decisive findings, and groundbreaking insights.
Embrace this guide to create a strong, persuasive, and impactful research proposal. Unleash the potential to stimulate interest, secure funding, kickstart your career or elevate your academic pursuits. Gain the confidence to contribute to your field with clarity, credibility, and conviction. It all starts with a proposal – your key to the world of profound discoveries and thought leadership.
What is a Research Proposal?
A research proposal is a systematic and concise synopsis of proposed research. Encompassing the key information, it serves as a roadmap to the principal components of the study. The main aim of a research proposal is to establish the necessity of the research, elucidate its aims and objectives, and illustrate the originality or significance of the proposed study. In essence, it is an application to conduct research, which is usually a crucial requisition for obtaining approval or funding.
The research proposal also elucidates the research methodology that will be adopted, which is crucial because it gives the reader an understanding of how the researcher will accomplish the outlined objectives. Precisely, it provides a glimpse of what the researcher aims to research, validates the relevance and significance of their project and elucidates their plan to accomplish it.
Why Use a Research Proposal Generator?
In the world of research and academia, crafting a compelling research proposal can often be a complex and time-consuming process. Devised to encapsulate your research’s crucial elements succinctly, a well-structured proposal seeks approval and funding for the projects that researchers wish to undertake. Here’s where a research proposal generator comes in handy. This innovative tool is designed to streamline the process, enabling users to create a thorough, accurate, and persuasive proposal while significantly reducing the time and effort typically associated with this task.
- Saves Time : With a research proposal generator, researchers can bypass the long hours typically spent on outlining, structuring, and formatting proposals. The tool automatically generates a thorough and well-structured proposal, enabling the researcher to focus more on substantive content, thereby reducing time expenditure.
- Improves Accuracy : The research proposal generator is programmed based on standard formats and structures of proposals making it less likely for researchers to miss out on key elements or make errors in structure. This improved accuracy helps in enhancing the proposal’s overall quality and likelihood of approval.
- Enhances Efficiency : By automating repetitive and mundane tasks, proposal generators enhance research efficiency. This, in turn, leaves room for researchers to concentrate on creative and critical thinking processes, essential for any successful research effort.
- Easy to Use : Regardless of your experience or familiarity with proposal writing, a generator is intuitive and easy to use, thereby making it accessible to a broad range of researchers, including those just starting.
- Cost-Effective : In comparison to hiring professionals or buying expensive software, using a research proposal generator is a cost-effective alternative. For institutions or researchers operating on a tight budget, this might prove to be the ideal solution.
According to an old saying, “Work smarter, not harder.” This adage is the underlying principle behind the creation of a research proposal generator. It’s not about replacing human intelligence but maximizing it.
How To Use This AI Generator:
- Click “Use Generator” to create a project instantly in your workspace.
- Click “Save Generator” to create a reusable template for you and your team.
- Customize your project , make it your own, and get work done!
Global main menu
- School of Electronic Engineering and Computer Science
- PhD studentships
DeepMind PhD Studentship in AI or Machine Learning
About the Studentship
Queen Mary University of London is inviting applications for the DeepMind PhD Studentship for September 2023.
The DeepMind PhD Studentship programme is established at Queen Mary University of London in partnership with leading British AI company, DeepMind.
The PhD Studentship supports and encourages under-represented groups, namely female and Black researchers, to pursue postgraduate research in AI or Machine Learning.
The PhD DeepMind Studentship will cover tuition fees and offer a London weighted stipend of £19,668 per year minimum together with an annual £2,200 travel and conference allowance and a one-off equipment grant of £1,700.
- 3-year fully-funded PhD Studentship
- Access to cutting-edge facilities and expertise in AI
- Partnership and mentorship with DeepMind employees working at the cutting edge of AI research and technologies.
Who can apply Queen Mary is on the lookout for the best and brightest students in the fields of AI and Machine Learning.
Successful applicants will have the following profile:
- Identify as female and/or are of Black ethnicity, each being under-represented groups in the field of Artificial Intelligence and Computer Science
- Should hold, or is expected to obtain an MSc in Computer Science, Electronic Engineering, AI, Physics or Mathematics or a closely related discipline; or can demonstrate evidence of equivalent work experience
- Having obtained distinction or first-class level degree is highly desirable
- Programming skills are strongly desirable; however, we do not consider this to be an essential criterion if candidates have complementary strengths.
We actively encourage applications from candidates who are ordinarily resident in the UK. The studentship is also open to International applicants.
About the School of Electronic Engineering and Computer Science at Queen Mary
The PhD Studentship will be based in the School of Electronic Engineering and Computer Science (EECS) at Queen Mary University of London. As a multidisciplinary School, we are well known for our pioneering research and pride ourselves on our world-class projects. We are 8th in the UK for computer science research (REF 2021) and 7th in the UK for engineering research (REF 2021). The School is a dynamic community of approximately 350 PhD students and 80 research assistants working on research centred around a number of research groups in several areas, including Antennas and Electromagnetics, Computing and Data Science, Communication Systems, Computer Vision, Cognitive Science, Digital Music, Games and AI, Multimedia and Vision, Networks, Risk and Information Management, Robotics and Theory
For further information about research in the school of Electronic Engineering and Computer Science, please visit: http://eecs.qmul.ac.uk/research/ .
How to apply
Queen Mary is interested in developing the next generation of outstanding researchers - whether in academia, industry or government – therefore the project undertaken under this Studentship is expected to fit into the wider research programme of School. Applicants should select a supervisor (a first and second choice) from the School at application stage. Visit our website for information about our research groups and supervisors: eecs.qmul.ac.uk/phd/phd-opportunities/
Applicants should submit their interest by returning the following to [email protected] by 12pm (noon), 10 April 2023:
- Indicate first and second choice academic supervisor
- CV (max 2 pages)
- Cover letter (max 4,500 characters)
- Research proposal (max 500 words)
- 2 References
- Certificate of English Language (for students whose first language is not English)
- Other Certificates
Application deadline: 10 April 2023
Applications will be reviewed by a panel of academic staff: May 2023
Interviews: April/May 2023
Start date: September 2023
- Accreditations
- Facts and Figures
- Faculty Positions
- Maps and Directions
- Student Mentoring Program
- Current Students
- Engineering Honors
- Global Programs
- Admissions and Aid
- Prospective Students
- Entry to a Major Process
- Graduate Admissions
- Scholarships and Financial Aid
- Facilities and Equipment
- Faculty Research Specialty Areas
- Undergraduate Research
- Affiliated Faculty
- Emeritus Faculty
Doctor of Philosophy in Artificial Intelligence and Data Science
The Doctor of Philosophy (Ph.D.) degree is a research-oriented degree requiring a minimum of 64 semester credit hours of approved courses and research beyond the Master of Science (M.S.) degree [96 credit hours beyond the Bachelor of Science (B.S.) degree]. The university places limitations on these credit hours in addition to the requirements of the Department of Civil Engineering.
A complete discussion of all university requirements is found in the current Texas A&M University Graduate Catalog .
NOTE: All documents requiring departmental signatures must be submitted to the Civil Engineering Graduate Office in DLEB 101 at least one day prior to the Office of Graduate Studies deadline.
Artificial Intelligence and Data Science Faculty Members
- Dr. Mark Burris
- Dr. Nasir Gharaibeh
- Dr. Stefan Hurlebaus
- Dr. Dominique Lord
- Dr. Xingmao “Samuel” Ma
- Dr. Ali Mostafavi
- Dr. Arash Noshadravan
- Dr. Stephanie Paal
- Dr. Luca Quadrifoglio
- Dr. Scott Socolofsky
- Dr. Yunlong Zhang
Admission Admission to the AI/DS track is conditional upon meeting the general admission requirements. Also, students may only be admitted to the AI/DS track if a faculty member affiliated with the track is willing to supervise (and provide funding support via GAT or GAR or Fellowship) for the student. If a current student is approved to change from one track to another, they must complete the Track Change Request Form and send it to the CVEN Graduate Advising Office so notification can be sent to their original area coordinator. Please read the CVEN department policy on changing tracks.
Departmental Requirements In addition to fulfilling the University requirements for the Doctor of Philosophy (Ph.D.) degree, a student enrolled in the Civil Engineering graduate program in the area of Artificial Intelligence and Data Science area must satisfy the following department requirements.
- A minimum of 32 credit hours of graduate-level coursework taken through Texas A&M University [a minimum of 24 credit hours if the student already has taken at least another 24 credit hours of graduate course work for the Master of Science (M.S.) or Master of Engineering (MEng) degree].
- Remaining coursework requirement can be met by 32 hours of CVEN 691.
- Qualifying Exam
- Degree Plan
- Written Preliminary Exam
- Research Proposal
- Oral Preliminary Exam
- Completion of Dissertation
- Final Defense
Dissertation Topic Students pursuing the AI/DS track would work on dissertation topics with a great extent of interdisciplinary elements spanning across civil engineering and computer science/AI. Such interdisciplinary research would require a student to develop depth of knowledge and skills across both domains.
Committee The committee of Ph.D. students in the AI/DS track can be composed of faculty from different departments with backgrounds and skills related to the subject matter of the dissertation research.
Students in the AI/DS track are strongly encouraged to form their dissertation committee prior to the qualification exam. If the dissertation committee is formed prior to the qualification exam, the exam questions will be developed by the committee in coordination with the AI/DS Track Coordinator. If the student's dissertation committee is not formed at the time of the qualification examination, the Track Coordinator and the student advisor will handle the development of the qualification examination.
|
|
|
650 | STAT FND DATA SCIENCE | STAT |
647 | SPATIAL STATISTICS | STAT |
616 | STAT ASPECTS OF MACH LEARN I | STAT |
765 | MACH LEARN WITH NETWORKS | ECEN |
654 | STAT COMPUTING WITH R & PYTHON | STAT |
651 | STAT IN RESEARCH I | STAT |
633 | MACHINE LEARNING | CSCE |
639 | DATA MINING & ANALYSIS | STAT |
689 | SPTP: NETWORK SCI OF CITIES | URSC |
651 | STAT IN RESEARCH I | STAT |
689 | SPTP: NETWORK SCIENCE OF CITIES | URSC |
689 | SPTP: PROGRAMING IN URBAN ANALYTICS | URSC |
689 | Machine Intelligence and Applications in CE | CVEN |
An official website of the United States government
Here's how you know
Official websites use .gov A .gov website belongs to an official government organization in the United States.
Secure .gov websites use HTTPS. A lock ( Lock Locked padlock ) or https:// means you've safely connected to the .gov website. Share sensitive information only on official, secure websites.
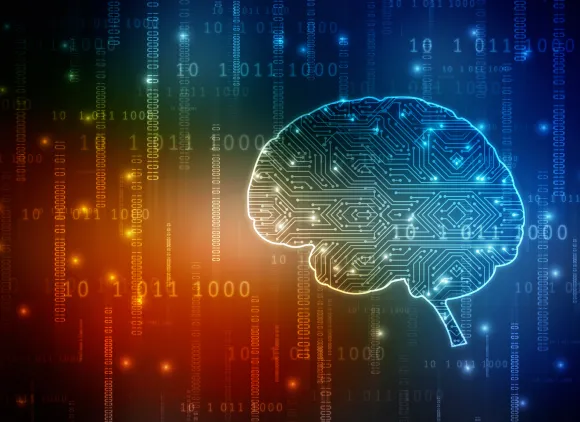
Artificial Intelligence
The U.S. National Science Foundation has invested in foundational artificial intelligence research since the early 1960s, setting the stage for today’s understanding and use of AI technologies.
AI-driven discoveries and technologies are transforming Americans' daily lives — promising practical solutions to global challenges, from food production and climate change to healthcare and education.
The growing adoption of AI also calls for a deeper understanding of its potential risks, like the amplification of bias, displacement of workers, or misuse by malicious actors to cause harm.
As a major federal funder of AI research, NSF advances AI breakthroughs that push the frontiers of knowledge, benefit people, and are aligned to the needs of society.
On this page
What is artificial intelligence?
How does AI affect our daily lives? How does it work in simple terms? Can we trust AI chatbots? In this 10-minute video, Michael Littman, NSF division director for Information and Intelligent Systems, looks at where the field of artificial intelligence has been and where it's going.
Brought to you by NSF
NSF's decades of sustained investments have ensured the continual advancement of AI research. Pioneering work supported by NSF includes:
Reinforcement learning
Which refines chatbots and trains self-driving cars, among other uses.
Neural networks
Which underlie breakthroughs in pattern recognition, image processing and natural language processing.
Large language models
Which power generative AI systems like ChatGPT.
Collaborative filtering
Which fuels content recommendation on the world's largest marketplaces and content platforms, from Amazon to Netflix.
AI-driven learning
Including virtual teachers (both digital and robotic) that incorporate speech, gesture, gaze and facial expression.
What we support
With investments of over $700 million each year, NSF supports:
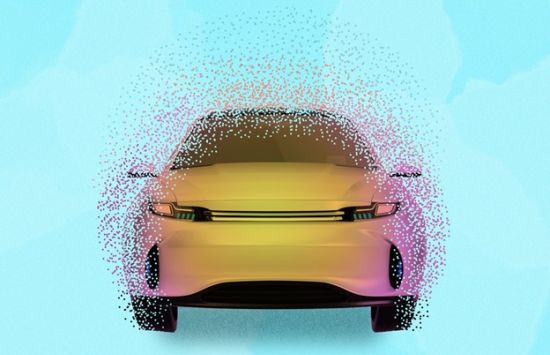
Innovation in AI methods
We invest in foundational research to understand and develop systems that can sense, learn, reason, communicate and act in the world.
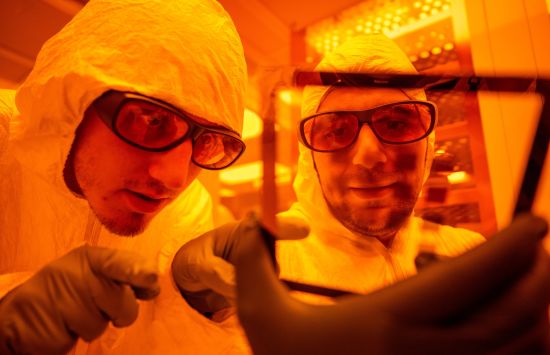
Application of AI techniques and tools
We invest in the application of AI across science and engineering to push the frontiers of knowledge and address pressing societal challenges.
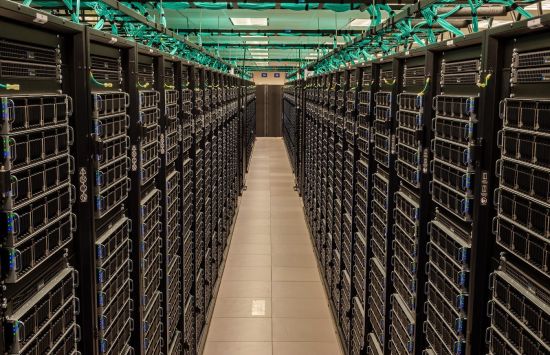
Democratizing AI research resources
We enable access to resources — like computational infrastructure, data, software, testbeds and training — to engage the full breadth of the nation's talent in AI innovation.
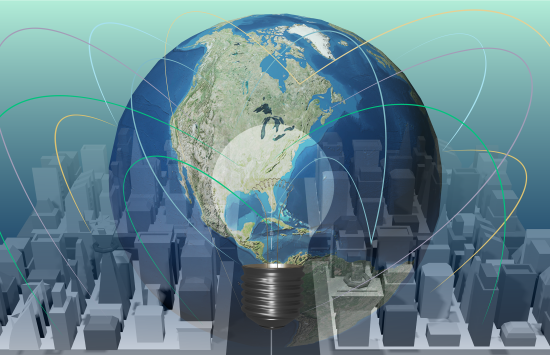
Trustworthy and ethical AI
We invest in the development of AI that is safe, secure, fair, transparent and accountable, while ensuring privacy, civil rights and civil liberties.
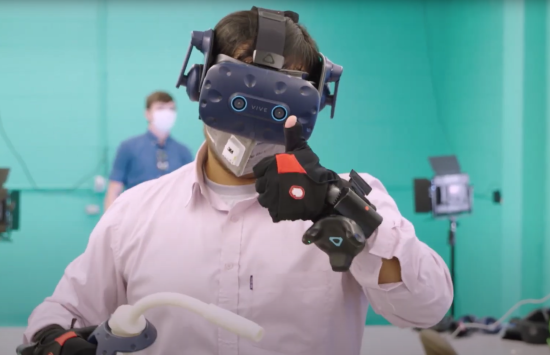
Education and workforce development
We invest in the creation of educational tools, materials, fellowships and curricula to enhance learning and foster an AI-ready workforce.
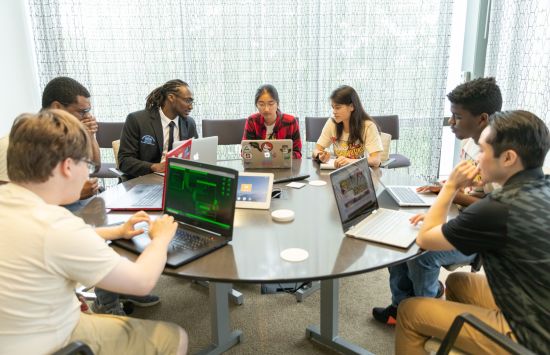
Partnerships to accelerate progress
We partner with other federal agencies, industry and nonprofits to leverage expertise; identify use cases; and improve access to data, tools and other resources.
National AI Research Institutes
Launched in 2020, the NSF-led National Artificial Intelligence Research Institutes program consists of 25 AI institutes that connect over 500 funded and collaborative institutions across the U.S. and around the world.
The AI institutes focus on different aspects of AI research, including but not limited to:
- Trustworthy and ethical AI.
- Foundations of machine learning.
- Agriculture and food systems.
- AI and advanced cybersecurity.
- Human-AI interaction and collaboration.
- AI-augmented learning.
Learn more by reading the 2020 , 2021 and 2023 AI Institutes announcements or visiting the AI Institutes Virtual Organization .
National AI Research Institutes: Interactive Map (PDF, 7.96 MB)
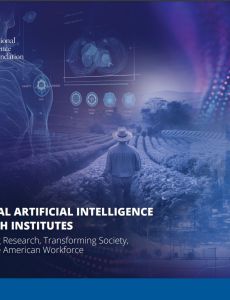
AI Institutes Booklet (PDF, 12.58 MB)
Hear from the newest ai research institutes.
- At the Edge of Artificial Intelligence This episode of NSF's Discovery Files podcast features three 2023 AI Research Institutes awardees discussing their work.
- The Frontier of Artificial Intelligence This Discovery Files episode features 2023 AI Research Institutes awardees applying AI to education, agriculture and weather forecasting.
National AI Research Resource Pilot
As part of the "National AI Initiative Act of 2020," the National AI Research Resource (NAIRR) Task Force was charged with creating a roadmap for a shared research infrastructure that would provide U.S.-based researchers, educators and students with significantly expanded access to computational resources, high-quality data, educational tools and user support.
The NSF-led interagency NAIRR Pilot will bring together government-supported, industry and other contributed resources to demonstrate the NAIRR concept and deliver early capabilities to the U.S. research and education community, including the full range of institutions of higher education and federally funded startups and small businesses.
The NAIRR Pilot is aimed to accelerate AI-dependent research such as:
- Societally relevant research on AI safety, reliability, security and privacy.
- Advances in cancer treatment and individual health outcomes.
- Supporting resilience and optimization of agricultural, water and grid infrastructure.
- Improving design, control and quality of advanced manufacturing systems.
- Addressing Earth and environmental challenges via the integration of diverse data and models.
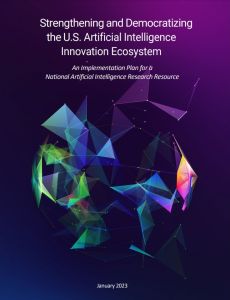
Implementation Plan for a National Artificial Intelligence Research Resource (PDF, 3.02 MB)
Featured funding.

Computer and Information Science and Engineering: Core Programs
Supports foundational and use-inspired research in AI, data science and human-computer interaction — including human language technologies, computer vision, human-AI interaction, and theory of machine learning.

America's Seed Fund (SBIR/STTR)
Supports startups and small businesses to translate research into products and services, including AI systems and AI-based hardware , for the public good.

Cyber-Physical Systems
Supports research on engineered systems with a seamless integration of cyber and physical components, such as computation, control, networking, learning, autonomy, security, privacy and verification, for a range of application domains.

Engineering Design and Systems Engineering
Supports fundamental research on the design of engineered artifacts — devices, products, processes, platforms, materials, organizations, systems and systems of systems.

Ethical and Responsible Research
Supports research on what promotes responsible and ethical conduct of research in AI and other areas as well as how to encourage researchers, practitioners and educators at all career stages to conduct research with integrity.

Expanding AI Innovation through Capacity Building and Partnerships
Supports capacity-development projects and partnerships within the National AI Research Institutes ecosystem that help broaden participation in artificial intelligence research, education and workforce development.

Experiential Learning for Emerging and Novel Technologies
Supports experiential learning opportunities that provide cohorts of diverse learners with the skills needed to succeed in artificial intelligence and other emerging technology fields.

Responsible Design, Development and Deployment of Technologies
Supports research, implementation and education projects involving multi-sector teams that focus on the responsible design, development or deployment of technologies.

Research on Innovative Technologies for Enhanced Learning
Supports early-stage research in emerging technologies such as AI, robotics and immersive or augmenting technologies for teaching and learning that respond to pressing needs in real-world educational environments.

Secure and Trustworthy Cyberspace
Supports research addressing cybersecurity and privacy, drawing on expertise in one or more of these areas: computing, communication and information sciences; engineering; economics; education; mathematics; statistics; and social and behavioral sciences.

Smart and Connected Communities
Supports use-inspired research that addresses communities' social, economic and environmental challenges by integrating intelligent technologies with the natural and built environments.

Smart Health and Biomedical Research in the Era of Artificial Intelligence
Supports the development of new methods that intuitively and intelligently collect, sense, connect, analyze and interpret data from individuals, devices and systems.
NSF directorates supporting AI research
Computer and information science and engineering (cise), engineering (eng), technology, innovation and partnerships (tip), mathematical and physical sciences (mps), social, behavioral and economic sciences (sbe), stem education (edu), geosciences (geo), biological sciences (bio), international science and engineering (oise), integrative activities (oia), featured news.
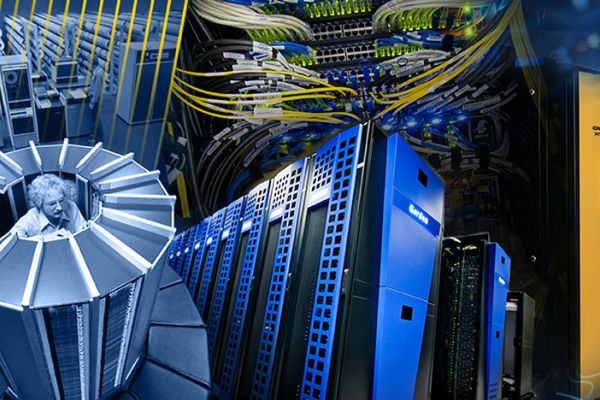
NSF-led National AI Research Resource Pilot awards first round access to 35 projects in partnership with DOE
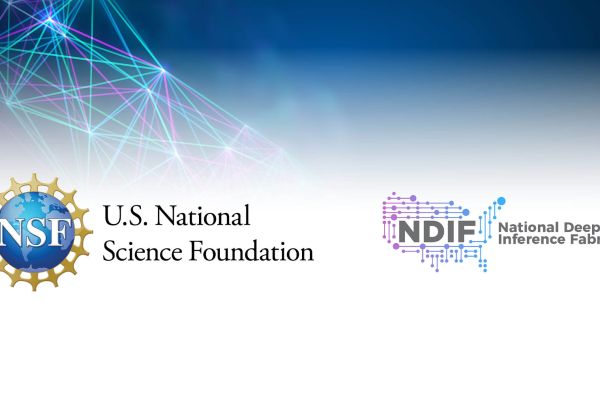
New NSF grant targets large language models and generative AI, exploring how they work and implications for societal impacts
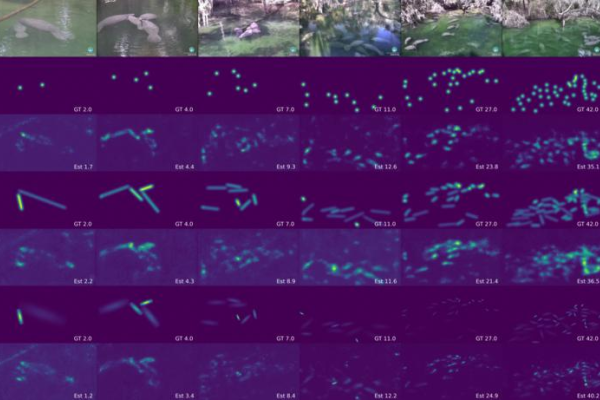

Saving an endangered species: New AI method counts manatee clusters in real time
Additional resources.
- NAIRR Pilot Explore opportunities for researchers, educators and students, including AI-ready datasets, pre-trained models and other NAIRR pilot resources.
- National Artificial Intelligence Initiative A coordinated federal approach to accelerate AI research and the integration of AI systems across all sectors of the economy and society.
- CloudBank Allows the research and education community to access cloud computing platforms.
- One Hundred Year Study on Artificial Intelligence A study focused on understanding and anticipating how AI will ripple through every aspect of how people work, live and play.
- Expanding the Frontiers of AI: Fact Sheet Learn how NSF is driving cutting-edge research on AI.
- "CHIPS and Science Act of 2022" The act authorizes historic investments in use-inspired, solutions-oriented research and innovation in key technology focus areas.
We have 81 Artificial Intelligence (fully funded) PhD Projects, Programmes & Scholarships
Computer Science
All locations
Institution
All Institutions
All PhD Types
All Funding
Artificial Intelligence (fully funded) PhD Projects, Programmes & Scholarships
Fully funded phd scholarship in multimodal user authentication and personalization at the center for computational, cognitive and connected imaging (c3i), phd research project.
PhD Research Projects are advertised opportunities to examine a pre-defined topic or answer a stated research question. Some projects may also provide scope for you to propose your own ideas and approaches.
Funded PhD Project (Students Worldwide)
This project has funding attached, subject to eligibility criteria. Applications for the project are welcome from all suitably qualified candidates, but its funding may be restricted to a limited set of nationalities. You should check the project and department details for more information.
Fully funded PhD position - Programming Group, Univ. of St.Gallen, Switzerland
Civil engineering: fully funded epsrc and twi phd scholarship: ai-driven investigation into predictive non-destructive evaluation, funded phd project (uk students only).
This research project has funding attached. It is only available to UK citizens or those who have been resident in the UK for a period of 3 years or more. Some projects, which are funded by charities or by the universities themselves may have more stringent restrictions.
Creative Technologies - Fully Funded PhD Studentship
The universities for nottingham and the centre for sustainable chemistry - phd scholarships available, funded phd programme (students worldwide).
Some or all of the PhD opportunities in this programme have funding attached. Applications for this programme are welcome from suitably qualified candidates worldwide. Funding may only be available to a limited set of nationalities and you should read the full programme details for further information.
4 Year PhD Programme
4 Year PhD Programmes are extended PhD opportunities that involve more training and preparation. You will usually complete taught courses in your first year (sometimes equivalent to a Masters in your subject) before choosing and proposing your research project. You will then research and submit your thesis in the normal way.
Use of Artificial Intelligence (A.I) and Machine Learning (ML) used for creating Navigational Situational awareness in Maritime Autonomous Surface Ships (MASS)
Smart acoustic control technologies, competition funded phd project (students worldwide).
This project is in competition for funding with other projects. Usually the project which receives the best applicant will be successful. Unsuccessful projects may still go ahead as self-funded opportunities. Applications for the project are welcome from all suitably qualified candidates, but potential funding may be restricted to a limited set of nationalities. You should check the project and department details for more information.
Large Language Models in Intelligent Robotic Systems for Environment Clean Up
Collaborative probabilistic machine learning, adaptive multi-access interoperable communication fabric for tinyedge, computational design of small molecules to prevent the early formation of multispecies biofilms, ai-optimised fermentation for sustainable protein production from food side streams, phd scholarship: “artificial intelligence for laser ultrasonic polycrystal imaging”, competition funded phd project (uk students only).
This research project is one of a number of projects at this institution. It is in competition for funding with one or more of these projects. Usually the project which receives the best applicant will be awarded the funding. The funding is only available to UK citizens or those who have been resident in the UK for a period of 3 years or more. Some projects, which are funded by charities or by the universities themselves may have more stringent restrictions.
Privacy-Aware and Trusted AI with Post Quantum Cryptography and Homomorphic Encryption
FindAPhD. Copyright 2005-2024 All rights reserved.
Unknown ( change )
Have you got time to answer some quick questions about PhD study?
Select your nearest city
You haven’t completed your profile yet. To get the most out of FindAPhD, finish your profile and receive these benefits:
- Monthly chance to win one of ten £10 Amazon vouchers ; winners will be notified every month.*
- The latest PhD projects delivered straight to your inbox
- Access to our £6,000 scholarship competition
- Weekly newsletter with funding opportunities, research proposal tips and much more
- Early access to our physical and virtual postgraduate study fairs
Or begin browsing FindAPhD.com
or begin browsing FindAPhD.com
*Offer only available for the duration of your active subscription, and subject to change. You MUST claim your prize within 72 hours, if not we will redraw.
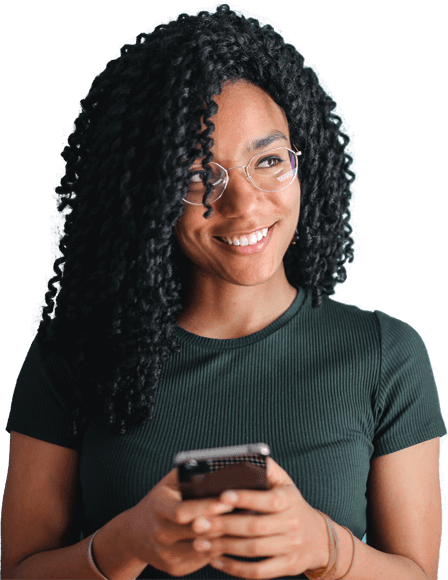
Do you want hassle-free information and advice?
Create your FindAPhD account and sign up to our newsletter:
- Find out about funding opportunities and application tips
- Receive weekly advice, student stories and the latest PhD news
- Hear about our upcoming study fairs
- Save your favourite projects, track enquiries and get personalised subject updates

Create your account
Looking to list your PhD opportunities? Log in here .
Filtering Results
- How it works
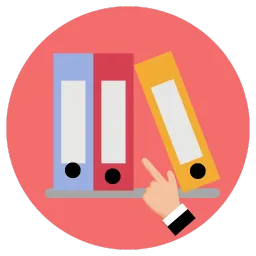
Useful Links
How much will your dissertation cost?
Have an expert academic write your dissertation paper!
Dissertation Services
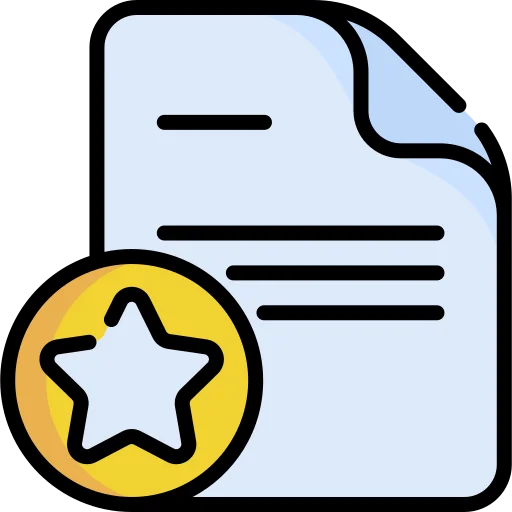
Get unlimited topic ideas and a dissertation plan for just £45.00
Order topics and plan
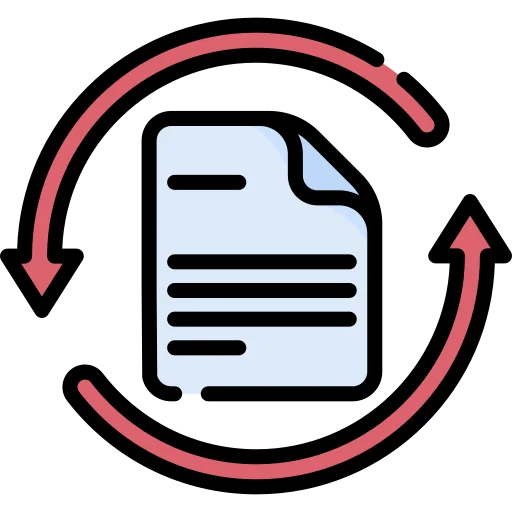
Get 1 free topic in your area of study with aim and justification
Yes I want the free topic
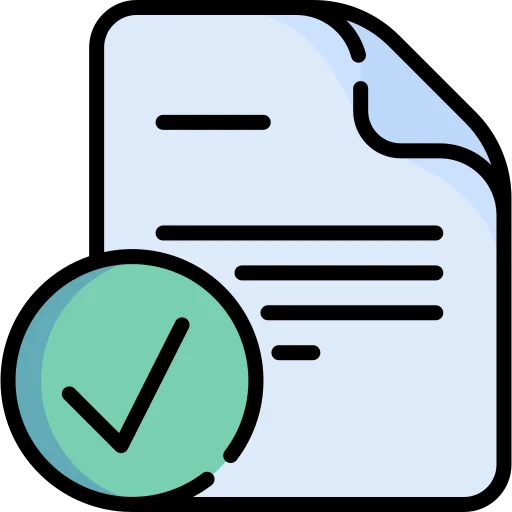
Artificial Intelligence Topics for Dissertations
Published by Carmen Troy at January 6th, 2023 , Revised On May 30, 2024
Artificial intelligence (AI) is the process of building machines, robots, and software that are intelligent enough to act like humans. With artificial intelligence, the world will move into a future where machines will be as knowledgeable as humans, and they will be able to work and act as humans.
When completely developed, AI-powered machines will replace a lot of humans in a lot of fields. But would that take away power from humans? Would it cause humans to suffer as these machines will be intelligent enough to carry out daily tasks and perform routine work? Will AI wreak havoc in the coming days? Well, these are questions that can only be answered after thorough research.
To understand how powerful AI machines will be in the future and what sort of world we will witness, here are the best AI topics you can choose for your dissertation.
You may also want to start your dissertation by requesting a brief research proposal from our writers on any of these topics, which includes an introduction to the topic, research question , aim and objectives , literature review , and the proposed methodology of research to be conducted. Let us know if you need any help in getting started.
Check our dissertation examples to get an idea of how to structure your dissertation .
Review the full list of dissertation topics for 2022 here.
You may also be interested in technology dissertation topics , computer engineering dissertation topics , networking dissertation topics , and data security dissertation topics .
List Of The Best Dissertation Topics & Ideas On AI
- How To Balance Transparency and Performance in Deep Learning Models
- The Ethical Implications of AI in Algorithmic Bias and Decision-Making
- How to Mitigate Threats and Secure Your Digital Presence Through AI
- Natural Language Processing for Real-world Applications
- AI in Substance Use Discovery and Development
- The Impact of AI on the Future of Transportation
- How to Enable Smart Cities and Connected Living
- The Use of AI in Combating Climate Change
- The Rise of Generative Adversarial Networks (GANs)
- The Impact of AI on Social Media: Content Moderation and the Challenge of Misinformation
- Can AI Achieve Artificial General Intelligence (AGI)? Exploring the Path to Human-Level Intelligence
- The Role of AI in Scientific Discovery
- AI for Personalised Finance
- How to Enhance Efficiency and Optimize Logistics through AI in Supply Chain Management
- Personalized Learning and Adaptive Teaching Systems
- AI for Fraud Detection and Prevention
- Automating Content Creation and the Future of News
- The Need for Human-Centered AI Design
- The Future of Work in the Age of AI: Automation, Upskilling, and the Evolving Job Market
- AI and the Creative Industries: Music Composition and Film Production
- How to Balance Innovation with Data Protection
- Can AI Achieve Sentience? Exploring the Philosophical and Scientific Implications
Topic 1: Artificial Intelligence (AI) and Supply Chain Management- An Assessment of the Present and Future Role Played by AI in Supply Chain Process: A Case of IBM Corporation in the US
Research Aim: This research aims to find the present, and future role AI plays in supply chain management. It will analyse how AI affects various components of the supply chain process, such as procurement, distribution, etc. It will use the case study of IBM Corporation, which uses AI in the US to make the supply chain process more efficient and reduce losses. Moreover, through various technological and business frameworks, it will recommend changes in the current AI-based supply chain models to improve their efficiency.
Topic 2: Artificial Intelligence (AI) and Blockchain Technology a Transition Towards Decentralised and Automated Finance- A Study to Find the Role of AI and Blockchains in Making Various Segments of Financial Sector Automated and Decentralised
This study will analyse the role of AI and blockchains in making various segments of financial markets (banking, insurance, investment, stock market, etc.) automated and decentralised. It will find how AI and blockchains can eliminate the part of intimidators and commission-charging players such as large banks and corporations to make the economy and financial system more efficient and cheaper. Therefore, it will study the applications of various AI and blockchain models to show how they can affect economic governance.
Topic 3: AI and Healthcare- A Comparative Analysis of the Machine Learning (ML) and Deep Learning Models for Cancer Diagnosis
Research Aim: This study aims to identify the role of AI in modern healthcare. It will analyse the efficacy of the contemporary ML and DL models for cancer diagnosis. It will find out how these models diagnose cancer, which technology, ML or DL, does it better, and how much more efficient. Moreover, it will also discuss criticism of these models and ways to improve them for better results.
Topic 4: Are AI and Big Data Analytics New Tools for Digital Innovation? An Assessment of Available Blockchain and Data Analytics Tools for Startup Development
Research Aim: This study aims to assess the role of present AI and data analytics tools for startup development. It will identify how modern startups use these technologies in their development stages to innovate and increase their effectiveness. Moreover, it will analyse its macroeconomic effects by examining its role in speeding up the startup culture, creating more employment, and raising incomes.
Topic 5: The Role of AI and Robotics in Economic Growth and Development- A Case of Emerging Economies
Research Aim: This study aims to find the impact of AI and Robotics on economic growth and development in emerging economies. It will identify how AI and Robotics speed up production and other business-related processes in emerging economies, create more employment, and raise aggregate income levels. Moreover, it will show how it leads to innovation and increasing attention towards learning modern skills such as web development, data analytics, data science, etc. Lastly, it will use two or three emerging countries as a case study to show the analysis.
Artificial Intelligence Research Topics
Topic 1: machine learning and artificial intelligence in the next generation wearable devices.
Research Aim: This study will aim to understand the role of machine learning and big data in the future of wearables. The research will focus on how an individual’s health and wellbeing can be improved with devices that are powered by AI. The study will first focus on the concept of ML and its implications in various fields. Then, it will be narrowed down to the role of machine learning in the future of wearable devices and how it can help individuals improve their daily routine and lifestyle and move towards a better and healthier life. The research will then conclude how ML will play a role in the future of wearables and help people improve their well-being.
Topic 2: Automation, machine learning and artificial intelligence in the field of medicine
Research Aim: Machine learning and artificial intelligence play a huge role in the field of medicine. From diagnosis to treatment, artificial intelligence is playing a crucial role in the healthcare industry today. This study will highlight how machine learning and automation can help doctors provide the right treatment to patients at the right time. With AI-powered machines, advanced diagnostic tests are being introduced to track diseases much before their occurrence. Moreover, AI is also helping in developing drugs at a faster pace and personalised treatment. All these aspects will be discussed in this study with relevant case studies.
Topic 3: Robotics and artificial intelligence – Assessing the Impact on business and economics
Research Aim: Businesses are changing the way they work due to technological advancements. Robotics and artificial intelligence have paved the way for new technologies and new methods of working. Many people argue that the introduction of robotics and AI will adversely impact humans, as most of them might be replaced by AI-powered machines. While this cannot be denied, this artificial intelligence research topic will aim to understand how much businesses will be impacted by these new technologies and assess the future of robotics and artificial intelligence in different businesses.
Topic 4: Artificial intelligence governance: Ethical, legal and social challenges
Research Aim: With artificial intelligence taking over the world, many people have reservations about the technology tracking people and their activities 24/7. They have called for strict governance of these intelligent systems and demanded that this technology be fair and transparent. This research will address these issues and present the ethical, legal, and social challenges governing AI-powered systems. The study will be qualitative in nature and will talk about the various ways through which artificial intelligence systems can be governed. It will also address the challenges that will hinder fair and transparent governance.
Topic 5: Will quantum computing improve artificial intelligence? An analysis
Research Aim: Quantum computing (QC) is set to revolutionise the field of artificial intelligence. According to experts, quantum computing combined with artificial intelligence will change medicine, business, and the economy. This research will first introduce the concept of quantum computing and will explain how powerful it is. The study will then talk about how quantum computing will change and help increase the efficiency of artificially intelligent systems. Examples of algorithms that quantum computing utilises will also be presented to help explain how this field of computer science will help improve artificial intelligence.
Topic 6: The role of deep learning in building intelligent systems
Research Aim: Deep learning, an essential branch of artificial intelligence, utilises neural networks to assess various factors similar to a human neural system. This research will introduce the concept of deep learning and discuss how it works in artificial intelligence. Deep learning algorithms will also be explored in this study to have a deeper understanding of this artificial intelligence topic. Using case examples and evidence, the research will explore how deep learning assists in creating machines that are intelligent and how they can process information like a human being. The various applications of deep learning will also be discussed in this study.
Topic 7: Evaluating the role of natural language processing in artificial intelligence
Research Aim: Natural language processing (NLP) is an essential element of artificial intelligence. It provides systems and machines with the ability to read, understand and interpret the human language. With the help of natural language processing, systems can even measure sentiments and predict which parts of human language are important. This research will aim to evaluate the role of this language in the field of artificial intelligence. It will further assist in understanding how natural language processing helps build intelligent systems that various organisations can use. Furthermore, the various applications of NLP will also be discussed.
Topic 8: Application of computer vision in building intelligent systems
Research Aim: Computer vision in the field of artificial intelligence makes systems so smart that they can analyse and understand images and pictures. These machines then derive some intelligence from the image that has been fed to the system. This research will first aim to understand computer vision and its role in artificial intelligence. A framework will be presented that will explain the working of computer vision in artificial intelligence. This study will present the applications of computer vision to clarify further how artificial intelligence uses computer vision to build smart systems.
Topic 9: Analysing the use of the IoT in artificial intelligence
Research Aim: The Internet of Things and artificial intelligence are two separate, powerful tools. IoT can connect devices wirelessly, which can perform a set of actions without human intervention. When this powerful tool is combined with artificial intelligence, systems become extremely powerful to simulate human behaviour and make decisions without human interference. This artificial intelligence topic will aim to analyse the use of the Internet of Things in artificial intelligence. Machines that use IoT and AI will be analysed, and the study will present how human behaviour is simulated so accurately.
Topic 10: Recommender systems – exploring its power in e-commerce
Research Aim: Recommender systems use algorithms to offer relevant suggestions to users. Be it a product, a service, a search result, or a movie/TV show/series. Users receive tons of recommendations after searching for a particular product or browsing their favourite TV show list. With the help of AI, recommender systems can offer relevant and accurate suggestions to users. The main aim of this research will be to explore the use of recommender systems in e-commerce. Industry giants use this tool to help customers find the product or service they are looking for and make the right decision. This research will discuss where recommender systems are used, how they are implemented, and their results for e-commerce businesses.
Free Dissertation Topic
Phone Number
Academic Level Select Academic Level Undergraduate Graduate PHD
Academic Subject
Area of Research
Frequently Asked Questions
How to find artificial intelligence dissertation topics.
To find artificial intelligence dissertation topics:
- Study recent AI advancements.
- Explore ethical concerns.
- Investigate AI in specific industries.
- Analyse AI’s societal impact.
- Consider human-AI interaction.
- Select a topic that aligns with your expertise and passion.
You May Also Like
In this article, we suggest some topics regarding Renewable Energy so you can kick start your dissertation without any delay.
If you have aimed to write your dissertation about leadership and direly looking for some exceptional leadership research topics, do not worry; we have got your back.
Need interesting computing engineering dissertation topics? Here are the trending Computing engineering dissertation titles so you can choose the most suitable one.
USEFUL LINKS
LEARNING RESOURCES
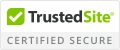
COMPANY DETAILS
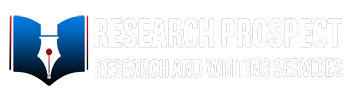
- How It Works

- Faculty of Medicine and Health
- Research and innovation
PhD opportunities to transform healthcare through AI
Research and innovation news Thursday 21 February 2019
T he University has announced 50 fully-funded PhD researcher places to unlock the potential of Artificial Intelligence (AI) in medical diagnosis and care.
A new centre for doctoral training (CDT) will be created, with a focus on the early detection, diagnosis, treatment and care of cancers.
It follows newly created government funding from UK Research and Innovation, to aid in training the next generation of AI healthcare pioneers.
Medical diagnosis and care
Professor David Hogg is leader of the new centre and professor of Artificial Intelligence at the University of Leeds.
“ Our PhD graduates will be equipped with broad skills in AI to innovate in diagnosis and care – in cancer and beyond. ” PROFESSOR DAVID HOGG, SCHOOL OF COMPUTING AT LEEDS
He said: “AI has the potential to make a real difference to patients with chronic diseases such as cancer."
"Early detection is critically important, identifying those at risk of cancer before symptoms appear, as well as identifying lifestyle changes that would reduce long-term risk.
“We can also ensure AI increases the reliability of diagnostic services, through faster and more accurate digital radiology and pathology, and it can provide clinicians with targeted and tailored care for each patient.
“ Leeds has an outstanding research and clinical reputation. ” PROFESSOR DAVID HOGG
“We are recruiting talented students from a range of background, from across science, engineering and health. While we will be focusing on cancer, where Leeds has an outstanding research and clinical reputation, our PhD graduates will be equipped with broad skills in AI to innovate in diagnosis and care – in cancer and beyond.”
Partnership with teaching hospital
Researchers will work alongside key national partners including Leeds Teaching Hospitals Trust (LTHT), the NHS, and wider industry.
LTHT is one of the largest teaching hospitals in all of Europe. It is known as a major centre of cancer research, with a world-renowned biomedical research facility and a leading clinical trials research unit.
Dr Christopher Herbert, Director of Operations: Research and Innovation at LTHT, said: “At the heart of the CDT is a close partnership with LTHT. This partnership will enable us to provide an outstanding and grounded training experience that maximizes the capacity of graduates to translate research into innovation within the NHS.
“We believe that AI will transform the way that medicine is practiced and how patients are managed over the next 20 years, and we want to be at the forefront of that revolution.”
The investment in this doctoral training centre further builds on the University’s well-established track record in transferring cross-disciplinary research ideas into world-leading clinical practice and products, leading to better outcomes for patients.
Researchers will collaborate with academic staff and work alongside clinicians and patients, positioning then as highly-skilled employees.
Data science and artificial intelligence
They will be hosted by the Leeds Institute for Data Analytics (LIDA) , established with University investment to support innovation in Medical Bioinformatics , funded by the Medical Research Council, and Consumer Data, funded by the Economic and Social Research Council.
The Institute has now grown to support a portfolio in excess of £45million of research across the University, bringing together over 150 researchers and data scientists, which includes a CDT in Data Analytics.
It supports the University’s partnership with the Alan Turing Institute , the UK’s national institute for data science and artificial intelligence.
“ Working with world class academic institutions and industry we will be able to train the next generation of top-tier AI talent and maintain the UK’s reputation as a trailblazer in emerging technologies. ” JEREMY WRIGHT, DIGITAL SECRETARY
Commenting on the UKRI investment, which will be supporting 16 new centres for doctoral training across the UK, Digital Secretary Jeremy Wright said: “The UK is not only the birthplace to the father of artificial intelligence, Alan Turing, but we are leading the way on work to ensure AI innovation has ethics at its core.
“We want to keep up this momentum and cement our reputation as pioneers in AI. Working with world class academic institutions and industry we will be able to train the next generation of top-tier AI talent and maintain the UK’s reputation as a trailblazer in emerging technologies.”
The Centre for Doctoral Training in Artificial Intelligence for Medical Diagnosis and Care will be welcoming the first cohort to join the University in September.
Further information
Read more about the government’s AI Sector Deal .
For interviews or further information please contact University of Leeds press office on [email protected] or +44(0)113 343 4031
Related News
See all Research and innovation news
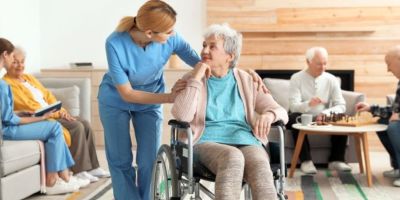
Care home staff and residents need ‘family’ bonds to thrive
Research and innovation - Tuesday 16 April 2024
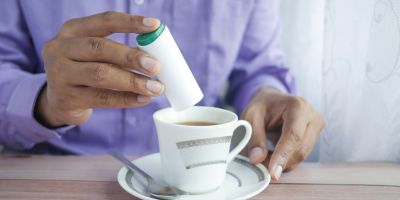
Sweeteners can improve weight loss maintenance, new research suggests
Research and innovation - Friday 22 March 2024
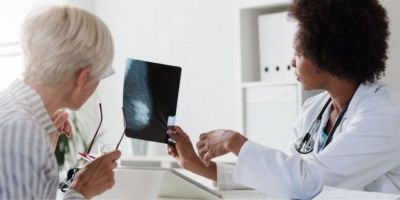
Cancer deaths plummet in middle aged people
Research and innovation - Friday 15 March 2024
Thank you for visiting nature.com. You are using a browser version with limited support for CSS. To obtain the best experience, we recommend you use a more up to date browser (or turn off compatibility mode in Internet Explorer). In the meantime, to ensure continued support, we are displaying the site without styles and JavaScript.
- View all journals
- Explore content
- About the journal
- Publish with us
- Sign up for alerts
- 26 June 2024
How I’m using AI tools to help universities maximize research impacts
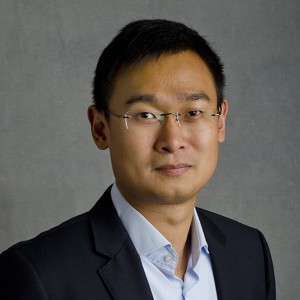
- Dashun Wang 0
Dashun Wang is a professor at the Kellogg School of Management and McCormick School of Engineering, and the founding director of the Center for Science of Science and Innovation at Northwestern University in Evanston, Illinois.
You can also search for this author in PubMed Google Scholar
You have full access to this article via your institution.
From the Internet to CRISPR–Cas9 gene editing, many seeds of progress were planted initially in the ivory tower of academia. Could research be doing even more for society? I argue that it could — if universities used artificial intelligence (AI) tools to maximize the impact of their scientists’ outputs.
Each year, millions of grant proposals, preprints and research papers are produced, along with patents, clinical trials and drug approvals. Massive data sets storing details of these outputs can be scoured by AI algorithms to better understand how science and technology progress and to identify gaps and bottlenecks that hinder breakthroughs. Over the past few years, my colleague and close collaborator Ben Jones, my team and I have been working with large US universities to maximize their research impacts. We’ve already learnt a lot.
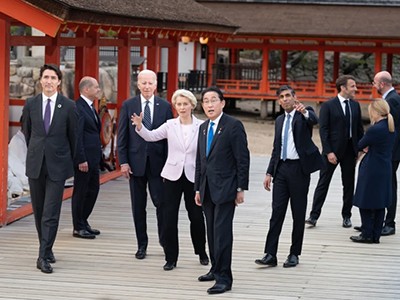
Revealed: the ten research papers that policy documents cite most
For example, during our pilot project at Northwestern University in Evanston, Illinois, we worked with one of its researchers in biology. She has published hundreds of papers and acquired tens of millions of dollars in research funding. By tracing her papers and grants and how her research has been used, we discovered an intriguing fact.
The researcher had never engaged with the university’s technology transfer office (TTO), yet her research had been used extensively by private companies worldwide — many of their patents cited her work. My collaborator Alicia Löffler, then head of the TTO, talked to the researcher. It turned out that she was unaware of those market impacts. Within one week of that conversation, the researcher filed her first invention disclosure with the university.
This episode raised several questions. How many scientists are in similar positions? Can researchers with untapped innovation potential be identified? And can the obstacles that hinder technological progress be addressed? To find out, Ben, Alicia and I, and our team, have expanded studies to other universities. Our preliminary work suggests that people in such positions are common.
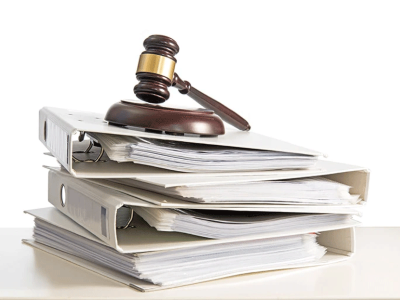
Has your research influenced policy? Use this free tool to check
For one, the researcher is a woman. When we compared how often male and female faculty members patented their work, we found a disparity. Male faculty members typically patented their research two to ten times more often than did their female counterparts, although this rate varied by university and discipline. But when we measured the extent to which the two groups’ scientific publications were cited by patents, we found no statistically significant difference. In other words, female scientists’ work is just as close to the technological frontier.
Numerous factors can contribute to this gender gap , such as unequal access to education and mentorship, funding disparities, prevailing norms and stereotypes and structural barriers in patenting and commercialization processes. A better understanding of these challenges would help to broaden the pool of innovators.
Similarly, we see a large difference between tenure-track and tenured faculty members: tenured researchers patent their work at a higher rate. But one doesn’t magically become more innovative the moment tenure is granted. The causes of this gap are probably distinct from those of the gender one, and might include promotion incentives and what counts towards tenure. But both discrepancies point to untapped opportunities for innovation.
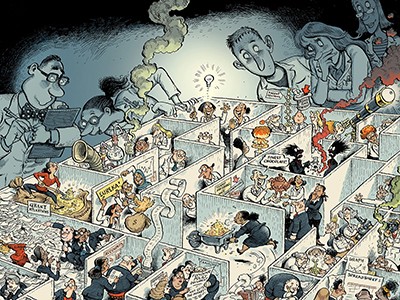
Want to speed up scientific progress? First understand how science policy works
Thus, data and AI tools can help institutions to identify people and ideas that are overlooked, both in a research institution and globally. But universities must take care. They have many roles and responsibilities — from educating future leaders to advancing fundamental knowledge — that must not be eclipsed by efforts to promote practical applications. Some people might argue that scientists don’t need to commercialize their ideas themselves, because industry can pick up the ball. Or there might be unintended consequences. Emphasizing what is useful could come at the expense of curiosity-driven research or result in flocking to what seem to be the hottest and most fruitful ideas today rather than to those that will help the world most in future.
But the role of science is changing. Many of today’s issues, from pandemics to climate change, are closely linked with scientific progress. The dichotomy of basic versus applied research is becoming inadequate. For example, advances along the science–society interface, such as discoveries that aid marketable applications ( M. Ahmadpoor and B. F. Jones Science 357 , 583–587; 2017 ) or social-science insights that guide policymaking ( Y. Yin et al. Nature Hum. Behav. 6 , 1344–1350; 2022 ), are highly impactful, as evidenced by high citation rates. By engaging more with use-inspired research, scientists can produce insights that both advance basic understanding and address societal needs.
Encouraging developments are under way. In 2022, the US National Science Foundation created the Directorate for Technology, Innovation and Partnerships to support use-inspired research and translate discoveries into real-world applications. Its Assessing and Predicting Technology Outcomes programme will fund innovative projects — including our work, which we plan to expand to more than 20 universities — to understand how investments in science and technology can best accelerate progress. Other nations, university leaders and policymakers must seize this opportunity, too. I think of science as ‘the little engine that could’. If research and development could be made even 5% more efficient, the returns could be immense.
Nature 630 , 794 (2024)
doi: https://doi.org/10.1038/d41586-024-02081-6
Reprints and permissions
Competing Interests
D.W. receives consulting fees from one of the universities he works with.
Related Articles
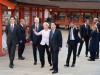
- Research management
- Computer science
To regain lost public trust, incorporate research ethics into graduate training
Correspondence 02 JUL 24
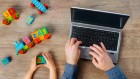
What it means to be a successful male academic
Career Column 26 JUN 24
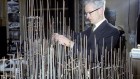
The strategy behind one of the most successful labs in the world
Comment 26 JUN 24
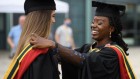
UK election: three research priorities for the next government
Editorial 02 JUL 24
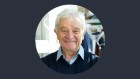
We can make the UK a science superpower — with a radical political manifesto
World View 18 JUN 24
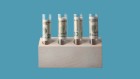
Securing your science: the researcher’s guide to financial management
Career Feature 14 JUN 24
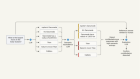
‘Fighting fire with fire’ — using LLMs to combat LLM hallucinations
News & Views 19 JUN 24
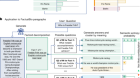
Detecting hallucinations in large language models using semantic entropy
Article 19 JUN 24
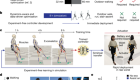
Experiment-free exoskeleton assistance via learning in simulation
Article 12 JUN 24
Postdoctoral / Research Scientist / Research Assistant positions in Molecular Immunology
Postdoctoral / Research Scientist / Research Assistant positions in Molecular Immunology / Cancer Immunology
Dallas, Texas (US)
The University of Texas Southwestern Medical Center (UT Southwestern Medical Center)
Alzheimer's Disease (AD) Researcher/Associate Researcher
Xiaoliang Sunney XIE’s Group is recruiting researchers specializing in Alzheimer's disease (AD).
Beijing, China
Changping Laboratory
Osaka University Immunology Frontier Research Center Postdoctoral Researcher
IFReC, Osaka University in Japan offers Advanced Postdoc Positions for Immunology, Cell Biology, Bioinformatics and Bioimaging.
Suita Campus, Osaka University in Osaka, Japan
Immunology Frontier Research Center, Osaka University
PostDoc Researcher, Magnetic Recording Materials Group, National Institute for Materials Science
Starting date would be after January 2025, but it is negotiable.
Tsukuba, Japan (JP)
National Institute for Materials Science
Tenure-Track/Tenured Faculty Positions
Tenure-Track/Tenured Faculty Positions in the fields of energy and resources.
Suzhou, Jiangsu, China
School of Sustainable Energy and Resources at Nanjing University
Sign up for the Nature Briefing newsletter — what matters in science, free to your inbox daily.
Quick links
- Explore articles by subject
- Guide to authors
- Editorial policies
Exploring the Impact of Artificial Intelligence in Teaching and Learning of Science: A Systematic Review of Empirical Research
- Open access
- Published: 27 June 2024
Cite this article
You have full access to this open access article
- Firas Almasri 1 , 2 , 3 , 4
383 Accesses
Explore all metrics
The use of Artificial Intelligence (AI) in education is transforming various dimensions of the education system, such as instructional practices, assessment strategies, and administrative processes. It also plays an active role in the progression of science education. This systematic review attempts to render an inherent understanding of the evidence-based interaction between AI and science education. Specifically, this study offers a consolidated analysis of AI’s impact on students’ learning outcomes, contexts of its adoption, students’ and teachers’ perceptions about its use, and the challenges of its use within science education. The present study followed the PRISMA guidelines to review empirical papers published from 2014 to 2023. In total, 74 records met the eligibility for this systematic study. Previous research provides evidence of AI integration into a variety of fields in physical and natural sciences in many countries across the globe. The results revealed that AI-powered tools are integrated into science education to achieve various pedagogical benefits, including enhancing the learning environment, creating quizzes, assessing students’ work, and predicting their academic performance. The findings from this paper have implications for teachers, educational administrators, and policymakers.
Similar content being viewed by others
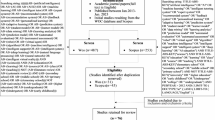
Artificial Intelligence in Science Education (2013–2023): Research Trends in Ten Years
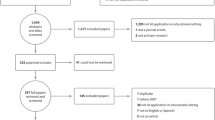
Systematic review of research on artificial intelligence applications in higher education – where are the educators?
Towards a Tripartite Research Agenda: A Scoping Review of Artificial Intelligence in Education Research
Avoid common mistakes on your manuscript.
Introduction
Artificial Intelligence (AI) is a broad field encompassing various technologies that have been developed over the past 50 years to enable machines to perform tasks traditionally requiring human intelligence, such as perceiving, reasoning, learning, and interacting (Ergen, 2019). However, recent advancements in generative AI (GenAI), particularly models like ChatGPT, have brought unprecedented attention to AI’s transformative potential across multiple industries (Hong et al., 2022 ; Lucci et al., 2022 ). Unlike predictive (pre-generative) AI which focuses predictions and decision making through a variety of machine learning and modelling techniques, Generative AI specializes in creating new content, such as text, images, and codes by using models of deep learning (Dai, 2023 ; Tang & Nichols, 2024 ). This distinction is essential to understand the breadth of AI applications in education.
Artificial intelligence in education (AIEd) is an evolving interdisciplinary arena incorporating AI technologies to renovate and enhance teaching and learning environments. Particularly, the application of AI in science teaching and learning is becoming more popular, even as interest in AI’s effects on general education is growing (Chiu et al., 2023 ; Gonzalez et al., 2017 ). More specifically, machine learning, a specific artificial intelligence technology, has been applied to automatically evaluate scientific models used in the education sector. Zhai et al. ( 2022 ) employed machine learning techniques to assess the quality of these models after gathering student responses to activities. Their research demonstrates how artificial intelligence can be used to automate assessment procedures and provide students with timely and detailed feedback on their work in the area of science education (Zhai, C Haudek, Zhai et al., 2020a , b , 2022 ). Similarly, Popenici and Kerr ( 2017 ) conducted a study to investigate the impact of AI on the teaching-learning process in higher education settings. Their study focused on how intelligent technologies are affecting student learning and traditional teaching approaches in education. Their research presents valuable insights into the incorporation of AI within science education contexts.
Zawacki-Richter et al. ( 2019 ), in their systematic assessment of AI applications in higher education, focused on the vital role that teachers can play in this domain. Their results suggest how important it is to explore and understand the needs and perceptions of teachers when integrating these technologies into teaching-learning settings. Likewise, Xu and Ouyang (2022) employed a systematic literature review method to identify and summarize research studies and classify the roles of AI in the educational system. Their findings advocate the use of AI is within the education environment to support its role in three ways: (1) AI as a new subject, (2) AI as an immediate mediator, and AI as a complementary aid to impact the teacher-learner, learner-self, and learner-learner relationships.
Though artificial intelligence has flourished in numerous domains within the education system, a comprehensive analysis of its role, advantages, and challenges in science education must be further explored through empirical investigations. This knowledge gap might prompt teachers, policymakers, and educational administrators to base decisions on patchy as well as limited information, lacking potential opportunities to enhance science teaching and learning with the help of AI. To fill this gap, the present paper provides a systematic review that comprehensively examines and consolidates AI’s impact on science education, evidenced by the empirical publications published from 2014 to 2023. While GenAI represents a significant leap in AI capabilities, this review considers the full spectrum of AI technologies, including both pre-GenAI and GenAI developments. In this way, we attempt to provide a holistic perspective on the current landscape, aiding stakeholders in leveraging AI’s potential while also considering its challenges and ethical implications for educational domains. The overarching objective of this review is to provide insights that could guide future research endeavors and advocate for evidence-based practices to enrich science education through the effective utilization of artificial intelligence.
Research Background
Overview of science education.
The goal of science education is not only to teach scientific knowledge but also to develop a scientifically literate populace capable of engaging in scientific reasoning and decision-making (Almasri, 2021 ; Grinnell, 2021 ). This aligns with the “Science for All” movement, emphasizing the importance of science education for all students, not just those pursuing careers in science (Almasri et al., 2022 ; Mansour, 2009 ). Students’ scientific literacy and critical thinking abilities are developed through the teaching and learning of scientific theories, procedures, and experiments in science education (Alharbi et al., 2022 ; Liu & Pásztor, 2022 ; Mogea, 2022 ; Zulyusri et al., 2023 ).
The nature of science education extends beyond content-based instruction to include student-centered activities and the development of scientific literacy for citizenship (Almasri et al., 2021 ; Irez, 2006a , b ; Kolstø, 2001 ). National development hinges significantly on robust contributions from the scientific community, driving economic growth and propelling the overall advancement of a nation (Hewapathirana & Almasri, 2022 ; Kola, 2013 ). The “Call to Action” for science education highlights an compelling necessity to improve educational approaches and make them consistent with the demands of the 21st century (Holme, 2021 ; Ibáñez & Delgado-Kloos, 2018 ). It is essential for developing students’ foundational knowledge, intriguing their curiosity, and getting them ready for STEM careers as per the contemporary world’s needs. Through AI incorporation, science education can be made more interesting, approachable, and pertinent for students of all ages and backgrounds by emphasizing experiential learning.
Prospects of Incorporating AI in Science Learning
With AI technology’s continuous evolution and popularity, the possibilities for its application in science education are promising but not without challenges. AI has the capability to transform the way science is taught and learned. One of the most compelling applications of AI in science education is its ability to simulate scientific experiments and provide virtual laboratory experiences to science learners. This ensures that students can practice and develop their scientific skills in a safe and controlled environment, potentially saving expenses and offering new opportunities for exploring scientific concepts that may not be feasible in traditional laboratory settings (Wahyono et al., 2019 ). However, these virtual experiences may lack the tactile and hands-on aspects of interaction with the physical world (Tang & Cooper, 2024 ), which are crucial for certain types of learning.
By leveraging AI, educators can also move away from traditional, one-size-fits-all approaches to education and instead provide personalized and interactive learning experiences for students. AI-powered algorithms can go beyond simply providing recommendations and assessments to conducting deep analyses of students’ learning patterns, allowing for highly personalized learning experiences (Zhai et al., 2021 ; Zhai et al., 2020a , b ). However, the effectiveness of these personalized learning systems depends heavily on the quality and representativeness of the data they are trained on, which can sometimes introduce biases and perpetuate existing inequities.
In addition, students can also benefit from immediate feedback and adaptive learning pathways, ensuring that they are able to address any misconceptions or gaps in their understanding of scientific phenomena (Mavroudi et al., 2018 ). AI can also help science educators track and monitor students’ progress more effectively, allowing for targeted interventions and support where necessary. Moreover, the use of AI can enable the development of interactive and immersive learning environments, making science education more engaging and accessible to students with diverse learning styles and needs. As AI continues to advance, the potential for its integration into science learning is likely to grow, presenting exciting opportunities to transform and elevate the science education experiences for students at all levels.
Anticipated Benefits of AI Implementation in Science Teaching
Artificial intelligence (AI) has numerous benefits in science education, profoundly affecting teaching and learning for science subjects. AI programs can study how students learn and change the material to fit each student’s needs, skills, and the way they learn. This way of creating educational material helps students learn better and faster. It lets them go at their own speed and in a way that matches how they like to learn (Zawacki-Richter et al., 2019 ). Also, AI-powered data analysis can help science teachers understand how well their students are doing in specific scientific subjects and where they might need extra help.
Another significant advantage is the improvement of exploratory learning through virtual labs and reenactments. AI-powered instruments have the potential to recreate complex logical tests, which may be illogical or hazardous to conduct in a conventional classroom setting. These virtual situations offer hands-on learning encounters and permit understudies to try distinctive scenarios, improving their understanding of scientific concepts (Ibáñez et al., 2018). This approach was not as supportive of extending understudy engagement but too valuable to democratize access to high-quality science instruction. AI devices can interface understudies and teachers over diverse geographies, empowering the trade of logical thoughts and cultivating a worldwide point of view on logical issues. This interconnecting also permits integrating differing datasets into the educational modules, uncovering understudies to real-world logical challenges and datasets (Holmes et al., 2023 ).
Ethical Considerations of AI Integration in Education
Even though artificial intelligence (AI) in education has bright futures, important ethical issues need to be resolved when integrating AI in the classroom. Many researchers have stressed considering the ethical implications and the need for character education in the era of AI (Burton et al., 2017 ; Cathrin & Wikandaru, 2023 ). The lack of critical reflection on the pedagogical and ethical implications and the risks of implementing AI applications in higher education underscores the need for a comprehensive ethical framework (Bozkurt et al., 2021 ).
Additionally, the integration of AI into educational settings presents new ethical obligations for teachers, necessitating a revaluation of ethical frameworks and responsibilities (Adams et al., 2022 ). Moreover, the introduction of ethics courses in academic training and capacity building of AI development actors can facilitate the integration of ethical values and the development of responsible AI (Kiemde & Kora, 2022 ). The ethical implications of AI in education extend beyond technical considerations to encompass broader societal impacts, such as privacy protection and social justice (Hermansyah et al., 2023 ).
Educators and students must understand, evaluate, and familiarize themselves with the uses of generative AI tools and consider their potential impacts on academic integrity. This involves recognizing when and how AI is used, assessing the reliability and validity of AI-generated outputs, and understanding the ethical and social implications of AI applications (Akgun & Greenhow, 2021 ). Moreover, the application of AI in education brings questions about educational equity and access to the fore. Systemic biases in AI algorithms and data can perpetuate inequities, making it crucial to address these biases effectively (Adams et al., 2022 ).
Purpose of the Study
The primary goal of the present study is to highlight the potential benefits as well as any disparities that might result from the widespread use of AI in science subjects. These discrepancies could be related to gaps in infrastructure, preparedness in the region, or accessibility. Eventually, the present investigation aims to furnish all relevant stakeholders, i.e., educators, learners, policymakers, and curriculum designers—with a deeper understanding of the current interaction between AI and science education.
The following research questions are addressed in this systematic review:
Impact on Learning Outcomes: How do AI tools impact student learning outcomes and engagement in science education?
Contexts of AI Adoption: What are the potential disparities in the uptake of AI tools within science education, considering differences among countries, educational levels, and subject areas?
Student and Teacher Perceptions: What are the perceptions and attitudes of students and educators towards the use of AI tools in science education?
Pedagogical Challenges: What are the identified challenges associated with using AI in science education?
Methodology
The authors worked diligently to explore how artificial intelligence contributes to science education thoroughly. We followed a structured process suggested by the widely used review methodology called Preferred Reporting Items for Systematic Reviews and Meta-Analyses (Page et al., 2021 ). The review approach of the current study comprised various stages – defining the study’s purpose along with specific research questions, formulating a protocol, an extensive literature search, a systematic screening process, extracting pertinent data, and synthesizing the findings. The sections below specifically mention how each of these steps was carried out for this study.
Search Strategy
We accessed a range of prominent digital repositories and databases to search the relevant literature. Particularly, IEEE Xplore, Springer, Tylor and Francis, ERIC (U.S. Dept. of Education), Science Direct, and Wiley were targeted to search the relevant literature. We also used Google Scholar and Google to make sure that we didn’t miss any important information. We used advanced search features to limit our search results to papers published between 2014 and 2023, ensuring that our search was focused and up to date (Piasecki et al., 2018 ).
We utilized a smart search strategy along with a range of search terms and operators to accomplish this. Our search strategy used a combination of key terms such as “artificial intelligence”, “AI”, “generative AI”, “ChatGPT,” “machine learning”, “robotics”, “intelligent system,” and “expert system” paired with descriptors like “science education,” “science learning,” or simply “science”. These combinations, along with their possible variations, were systematically applied to search within the papers’ titles, keywords, and abstracts. This search strategy was created with the aim to identify and consider a broad range of empirical work relating to the use of artificial intelligence in the teaching and learning of science.
Eligibility Criteria
Describing clear eligibility (inclusion and exclusion) criteria allows for setting boundaries for a systematic literature review. These criteria were aimed at creating a structured framework that facilitates the inclusion of studies meeting essential prerequisites while excluding those that don’t align with our research objectives. The inclusion criteria are as follows:
The paper must have employed empirical methods, such as quantitative, qualitative, or/and mixed methods, warranting a rigorous data collection and analysis approach.
The paper should have conducted research in an educational setting, encircling primary, middle, secondary, or higher education, emphasizing the applicability of the findings in educational environments.
A pivotal criterion necessitates the use of artificial intelligence in the study. This AI practice should have been applied to the teaching-learning process, and empirical data collected and integrated into the study.
Studies should be related to a science-related content area, spanning courses like chemistry, physics, biology, engineering, health sciences, or other related disciplines, ensuring applicability to the research topic.
The timeframe specified for publication years, from 2014 to 2023, targets to capture relevant studies within the past decade, ensuring the examination of recent developments in AI-based learning.
Our exclusion criteria were as follows:
Excluding studies that are not empirical in nature, such as theoretical papers, reviews, editorials, or opinion pieces, to maintain the focus on empirical research.
Studies written in languages other than English.
Studies that did not explicitly mention the AI use within a learning context.
Excluding studies that are solely available in abstract form and lack full-length publications.
The Screening Process
In the months of November and December 2023, we went on a thorough hunt for the required information. We started by searching through loads of databases and found 5,121 articles. After getting rid of duplicates, checking publication dates and titles, and looking at abstracts to see if they met the eligibility criteria for the present study, we ended up with 128 articles. From there, we excluded 41 studies because they didn’t really dive into science education. That left us with 87 articles that we pored over super carefully. We made sure they fit our criteria and answered our research questions before diving into them. From the pool of these 61 articles, ten (13) studies were identified as lacking clear empirical evidence regarding the use of artificial intelligence and were subsequently excluded. This process resulted in a final dataset of 74 articles that were included in the systematic review. See Table 1 for the list of studies included in our review. Figure 1 demonstrates a quick preview of the search strategy and the screening process.
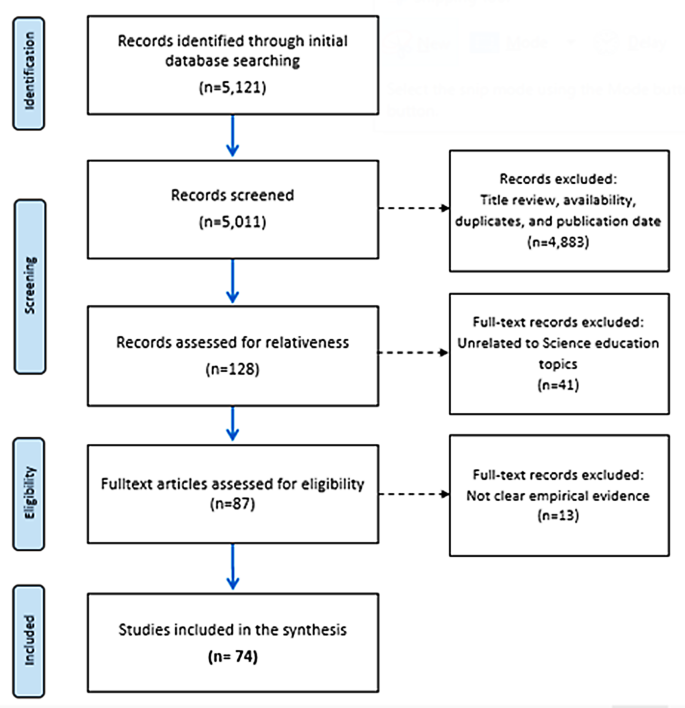
PRISMA review process
Coding and Analysis
We used a mix of qualitative and quantitative content analysis techniques to synthesize the findings of the empirical papers. To ensure inter-rater reliability in relation to the quality of article coding procedures, a small random sample consisting of 20 selected articles was independently coded by multiple raters. The calculated reliability level exceeded 92%, signifying a high degree of agreement across coding categories. We conducted a comprehensive examination of the studies from various perspectives. Firstly, we analyzed the characteristics of the data set, including the country where the studies were conducted, the journal name, the content area, and the educational level.
In this comprehensive review of the literature, we carefully evaluated seventy-four (74) empirical studies that deal with the incorporation of AI into science education. Numerous research approaches, such as mixed, qualitative, and quantitative approaches, were used in these studies. Examining the publication dates of the included papers revealed that they were dispersed over the review study’s 10-year focal period (2014 to 2023). The year 2023, with twenty-seven (27) papers, led the way, demonstrating researchers’ strong interest in the most recent research on the application of artificial intelligence in science education. This was followed by ten (10) studies in 2022, eight (08) studies in 2021, and nine (09) studies in year 2020. For more information on the year-wise publication, see Fig. 2 .
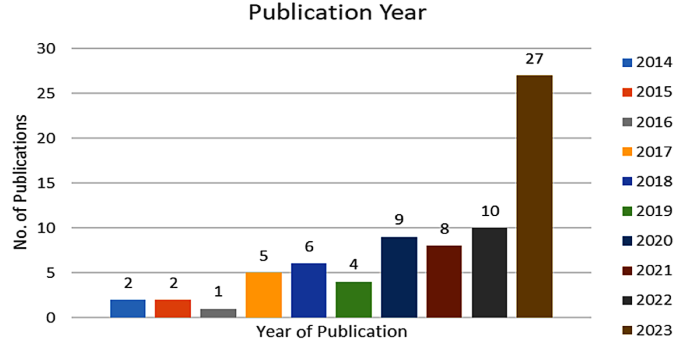
Year-wise publications
The review process of the present study involved the consolidation of findings pertaining to four distinct research questions, each of which is presented separately in the following sections.
RQ1: Impact on Outcomes Comparison
The first research question of the current study specifically addressed the primary intention of this systematic research i.e., analyzing the reported impact of AI-enhanced learning on students’ learning outcomes in science education. The empirical papers reviewed showed that artificial intelligence has been used within science education for a variety of purposes, such as engaging students in the learning process with a strong sense of motivation and interest (Balakrishnan, 2018 ), generating tests of science subjects (Aldabe & Maritxalar, 2014 ; Nasution & Education, 2023 ), scoring and providing personalized feedback on students’ assignments (Azcona et al., 2019 ; Maestrales et al., 2021 ; Mirchi et al., 2020 ), and predicting student performance (Blikstein et al., 2014 ; Buenaño-Fernández et al., 2019 ; Jiao et al., 2022a , b ).
AI-based tools were found to have a positive influence on student’ learning outcomes in science-related courses. The experimental group that was exposed to AI integration in their learning environments exhibited significantly higher scores in their academic tests compared to the control group who experienced traditional learning environments (Alneyadi & Wardat, 2023 ; Koć-Januchta et al., 2020 ). Ledesma and García ( 2017 ) and Lamb et al. ( 2021 ) highlighted AI’s capacity to identify complex concepts and enhance problem-solving skills significantly in subjects (Lamb et al., 2021 ; Ledesma & García, 2017 ). Ferrarelli and Iocchi ( 2021 ), Cochran et al. ( 2023 ), and Figueiredo and Paixão ( 2015 ) showcased how AI is helpful in fostering improved subject understanding and heightened motivation among students, particularly in physics and chemistry (Ferrarelli & Iocchi, 2021 ; Figueiredo et al., 2016 ).
Lee et al. ( 2022 ) argue that AI-based tools such as chatbots can help students become cognitively more active in the learning process(Lee et al., 2022 ). Likewise, Azcona ( 2019 ) suggests that personalized learning facilitated by AI can help reduce the gap between lower- and higher-performing students. Moreover, AI-powered education can empower students to predict their learning outcomes and strategically regulate their learning behavior (Buenaño-Fernández et al., 2019 ).
The effectiveness of different AI models varied across studies. Nguyen et al. ( 2023 ) highlighted the performance disparities among AI models like Google Bard, ChatGPT, and Bing Chat in addressing biology problems for Vietnamese students (Nguyen et al., 2023 ). While chatbots positively influenced online learning experiences, their impact on academic achievement remained variable (Almasri, 2022a ; Deveci Topal et al., 2021 ). In essence, these findings underscore the potential of AI to augment science education by enhancing student understanding, motivation, and engagement. However, they also underscore the importance of addressing challenges related to AI’s adaptability to subject matter and context and the need for continued exploration into AI’s comparative impact on academic achievement vis-à-vis traditional teaching methods in science education. Daher et al. ( 2023 ) pointed to AI’s limitations in comprehending specific subject matter, which could impact its effectiveness in aiding student learning. Cooper ( 2023 ) emphasized the need for educators to critically evaluate and adapt AI-generated resources to suit diverse teaching contexts.
RQ2: Contexts of AI Adoption
In our second research question, we aimed to explore the potential disparities in the uptake of AI tools within science education, considering differences among countries, educational levels, and subject areas. The results disclosed that artificial intelligence has been incorporated in a variety of subject areas within science education, including physical and natural sciences. The studies reviewed were highly dominated by investigations that did not specify any particular domain of science ( n = 15, 20.30%), but they preferred to use “Science” as the subject area in their papers. Next in line, was the subject of Physics with the second-highest number of papers ( n = 10, 13.50%). The list was continued by Biology and Programming with nine ( n = 9, 12.16%) and eight ( n = 8, 10.81%) papers, respectively. The subjects of Mathematics and Engineering occupied about 16% (with 06 papers each) of the total papers. Out of 74 studies, only five (05) studies were conducted to investigate the use of AI for AI education. The subjects of Computers/technology were focused on in four papers. Lastly, only one paper was centered around the use of artificial intelligence in Statistics and Earth Science. Figure 3 provides a summary of the content areas that were the focus of the papers included in our review.
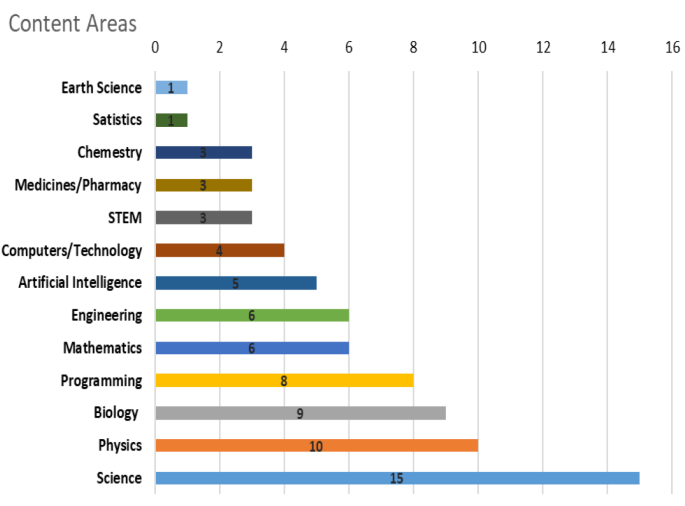
Studies distributed around subject areas within science education
While examining the various educational levels that benefited from the integration of artificial intelligence in some manner, we found that nearly half of the studies ( n = 35, 47%) belonged to undergraduate level, followed by high schools ( n = 15, 20%) and middle schools ( n = 7, 10%) respectively. Out of the total 74 papers, about 8% of the studies ( n = 6) were conducted in secondary school contexts. Likewise, 8% of the studies involved multiple levels of educational settings. In contrast, three of the studies (about 4%) were conducted in elementary school. Only 2% of the papers belonged to the college level, and only one study was conducted at the postgraduate and college levels. Figure 4 provides a quick distribution of the students in various educational contexts.
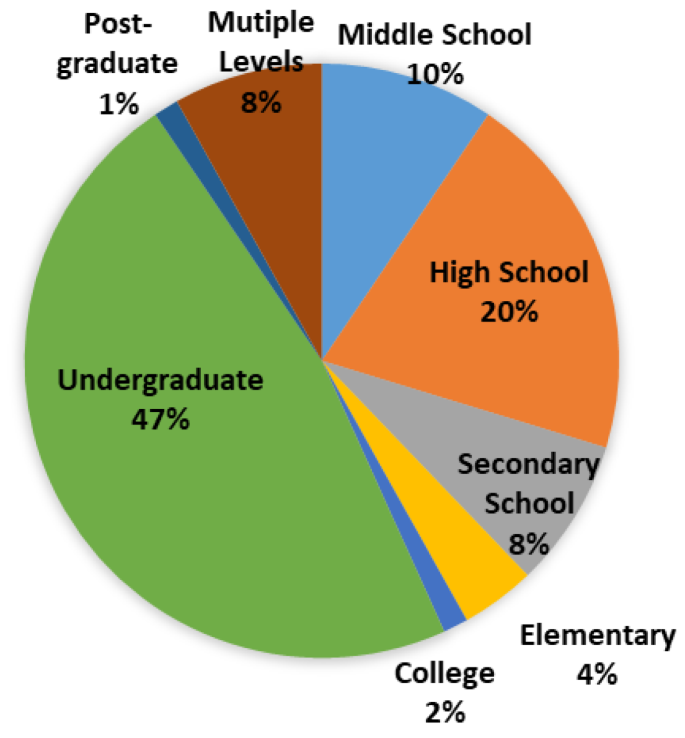
Studies distributed across various educational levels
Similarly, country-wise categorization of the papers exposed that about 38% of the studies ( n = 25) were conducted in the context of the United States. Germany ranked second in the list with six studies (8%). This was followed by four studies (5.4%) carried out in Turkey and Australia. UAE and Malaysia followed in the race, each with three papers. Eight countries, including Sweden, China, Mexico, Saudi Arabia, Spain, the Netherlands, Israel, and Taiwan, contributed about 21.6% of the total papers, each with two studies. The rest of the papers ( n = 10, 13.51%) were written in the context of 10 different countries across the globe (see Fig. 5 for details).
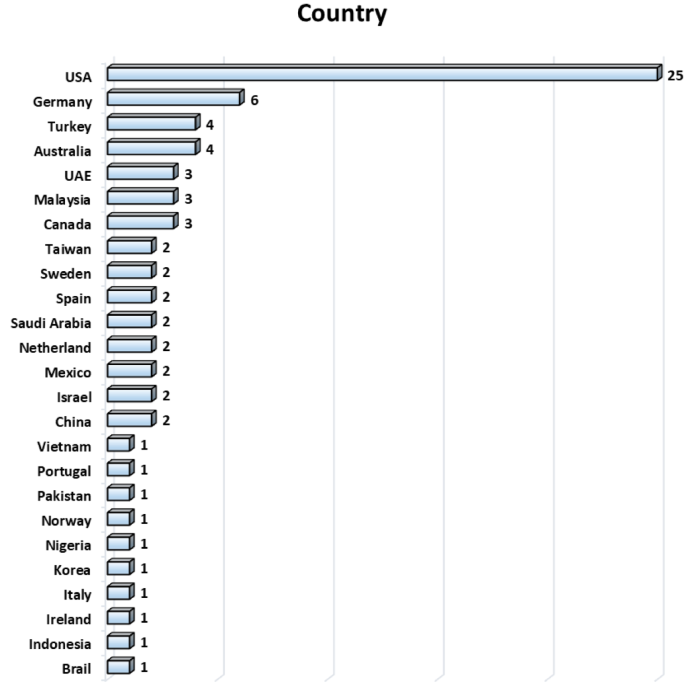
Country of research context
RQ3: Student and Teacher Perceptions
With our third research question, we attempted to explore science teachers’ and students’ perceptions regarding the integration of AI. The studies revealed multifaceted perspectives on the integration of AI in science education among both students and teachers. The effectiveness of AI tools in augmenting learning experiences garnered students’ attention. Students showcased increased engagement and improved subject understanding through AI-based interventions, indicating positive perceptions of AI’s efficacy in enhancing learning outcomes (Ferrarelli & Iocchi, 2021 ; Ledesma & García, 2017 ). For example, Bitzenbauer (2023) found that ChatGPT’s use in Physics classrooms favorably influenced students’ perceptions in Germany. Avelino et al. ( 2017 ) echoed this sentiment for undergraduate students in the United States.
Students reported their increased interest in science courses when AI was integrated into the learning environments. Students particularly admired the AI’s power to provide prediction and personalized feedback (Azcona et al., 2019 ). According to Elkhodr et al. ( 2023 ), science students perceive AI-based tools as useful and enjoyable learning resources, while most students showed a willingness to use them in the future.
Our analysis suggests that science teachers hold a high level of acceptance and positive attitudes toward AI’s utilization in the classroom. Teachers welcome its use with positive correlations to self-efficacy, ease of use, and behavioral intentions (Al Darayseh, 2023 ). They perceive this technology as the need of the hour to boost student engagement (Almasri, 2022b ; Nersa, 2020 ). Empirical papers included in the current study exposed fluctuating degrees of comfort and adaptability among educators and students in incorporating AI into their teaching and learning processes. Al Darayseh ( 2023 ) noted that science teachers exhibited favorable attitudes toward AI’s integration, possibly due to the perceived reduced effort in its utilization and their confidence in their essential skills to incorporate AI effectively.
There are several factors that influence teachers’ intentions and behavior regarding the use of AI, including self-esteem, expected benefits, ease of utilization, and their overall attitude toward AI applications. Teachers’ favorable disposition towards AI use is also due to their perception of reduced effort in its utilization.(Nja et al., 2023 ). Overall, teachers consider AI tools like ChatGPT to be helpful in designing science units, rubrics, and quizzes (Cooper, 2023 ). Yet, challenges associated with AI integration could influence students’ and teachers’ perceptions of AI’s reliability and accuracy in supporting educational goals, posing potential barriers to widespread acceptance and utilization.
RQ4: Pedagogical Challenges
Our analysis uncovered several challenges associated with the integration of AI in terms of complexities and limitations of its use within this particular domain of the education system. One prevalent challenge revolved around AI’s capability to comprehend and effectively address specific subject matter. Daher et al. ( 2023 ) highlighted instances where AI, like ChatGPT, encountered difficulties in understanding complex concepts in chemistry. They argue that the information provided by AI tools such as ChatGPT is limited because it depends on the data it was taught with. It might not have access to the latest or most complete knowledge in a particular domain.
Adaptability and contextual relevance emerged as significant concerns regarding the use of AI within science teaching. Cooper ( 2023 ) stressed that teachers critically evaluate AI-based resources and adapt them to their teaching contexts. He suggested that a one-size-fits-all approach might not suffice in accommodating the intricacies of varied educational environments. Another challenge pertained to the effectiveness and performance variability of different AI models. Nguyen et al. ( 2023 ) showcased the varying performance levels of different AI models, indicating disparities in their ability to address specific subject-related challenges. This variability in performance, as seen in different studies, implies the need for thorough evaluation and selection of appropriate AI tools tailored to the needs of specific subject areas. Furthermore, ethical considerations and limitations in AI’s current capabilities were notable concerns. Kieser et al. ( 2023 ) raised ethical issues regarding students using AI to fabricate data for class assignments. Addressing these challenges requires a nuanced approach that acknowledges the potential and constraints of AI while striving to optimize its role in enhancing science education effectively.
Discussions
The primary objective of this review was to investigate the interaction between artificial intelligence and science education. Our study uncovered a diverse landscape of AI usage within science education. Our results suggested that integrating AI tools in science education consistently improves students’ academic performance. This was evident in higher test scores and a better understanding of complex concepts compared to those in traditional learning environments (Alneyadi & Wardat, 2023 ; Koć-Januchta et al., 2020 ; Siddaway et al., 2019 ).
Literature suggests that integrating artificial intelligence into the teaching-learning process facilitates understanding complex scientific topics (Lamb et al., 2021 ; Ledesma & García, 2017 ). It also helps develop problem-solving skills considerably, leading to a better understanding of subjects, particularly in fields like physics and chemistry. Furthermore, it was revealed that science teachers use AI-driven tools to engage students effectively and foster their motivation and interest in science-related subjects (Balakrishnan, 2018 ). Personalized learning through AI tools helps bridge performance gaps between lower and higher-performing students (Azcona et al., 2019 ), contributing to a more equitable learning environment. AI-generated personalized feedback also contributed to students’ increased engagement in the learning process (Azcona et al., 2019 ; Maestrales et al., 2021 ; Mirchi et al., 2020 ).
The current systematic review suggests that the distribution of studies within various subject areas in science education showcases a dominant focus on science in general, followed by physics, biology, programming, and other specific science subjects. Some specific domains, like earth science and statistics, received comparatively the least attention in the reviewed literature.
The distribution of research papers across countries demonstrates certain disparities. The United States had a significantly higher number of studies compared to other nations. Germany ranked second on the list. Turkey and Australia followed, while UAE, Malaysia, and Canada contributed with a moderate number of studies. Several countries had minimal representation, with a diverse spread across multiple nations. Concentration of studies in certain countries like the United States and Germany might suggest varying levels of research infrastructure or prioritization of AI in education compared to other nations with fewer studies. This could potentially lead to disparities in the implementation and impact of AI tools in science education among different regions globally.
Our analysis found that students exhibit increased engagement and interest in science courses when AI tools are integrated into learning environments. This heightened interest is attributed to AI’s ability to provide predictions and personalized feedback (Jiao et al., 2022b ), making learning more engaging and enjoyable (Hewapathirana & Almasri, 2022 ). Students perceive AI-based tools as useful and beneficial for their learning experiences. They acknowledge AI’s effectiveness in improving subject understanding and express a willingness to continue using such tools in the future (Elkhodr et al., 2023 ).
Similar to students, science teachers also demonstrate positive attitudes and acceptance of AI tools in the classroom, correlating with perceived benefits in student engagement and their own teaching efficacy. Teachers view AI integration as a means to enhance student engagement, with some perceiving it as a way to reduce effort while teaching, leading to increased confidence in utilizing AI effectively (Al Darayseh, 2023 ). Specifically, teachers perceive ChatGPT a valuable resource for designing science units, rubrics, quizzes, and teaching aids, offering convenience and potential enhancement to their teaching methodologies.
While AI showed promise in improving learning outcomes, there are challenges related to its adaptability to subject matter and context. Some studies pointed out limitations in comprehending specific subjects, potentially impacting the effectiveness of AI in aiding student learning. Previous research suggests that AI tools like ChatGPT face difficulties in comprehending and addressing complex concepts in specific subject areas, as seen in instances within chemistry (Daher et al., 2023 ). The dependency on the data it was trained with limits its access to the latest or most comprehensive knowledge in particular domains. A uniform approach might not adequately cater to the complexities and nuances of varied educational environments, emphasizing the need for adaptable solutions (Cooper, 2023 ). Addressing these challenges requires a balanced approach that acknowledges AI’s potential and constraints in science education. Thus, teachers are advised to critically evaluate AI-generated resources and tailor them to diverse teaching contexts.
Our research provides important implications for teacher preparation and in-service professional development regarding AI in our society and implementing AI tools and processes in K-12 education (Antonenko & Abramowitz, 2023 ). As a whole, integrating artificial intelligence positively enhances the process and outcome of science education. However, there are certain limitations and challenges associated with its use. Providing training and support to educators to effectively utilize AI tools can enhance their confidence and capabilities in integrating these technologies into teaching practices. Moreover, establishing clear ethical guidelines and frameworks for the responsible use of AI in education can mitigate the risk of misuse and ensure ethical practices among students and educators.
Limitations
Some of the inherent limitations of this research review are discussed in this section. First, just like with other reviews, the search terms and strategies determine which research papers are included. Although a thorough and methodologically rigorous search was the goal, using different search terms might have turned up more articles that could have been included in the review. Furthermore, a few particular research databases were searched in order to find pertinent empirical literature for inclusion in this research review. An alternative methodological strategy would have involved restricting the search for research to a predetermined list of scholarly, peer-reviewed journals. A smaller sample of literature for inclusion may have occurred due to this strategy. However, greater control over validity, reliability, and credibility during the search and inclusion processes was sought to the best level. Lastly, we may have missed some grey literature, such as dissertations and conference proceedings, that was not indexed in the databases/repositories that we used.
This systematic review examined the impact, perceptions, and challenges associated with the integration of Artificial Intelligence (AI) in the teaching and learning of science. Our analysis uncovered a landscape rich in prospective benefits and challenges. The usage of AI in science education steadily established positive impacts on student learning outcomes. It encourages participation in the educational process, enhances comprehension of the subject, and boosts motivation in the students. Both students and teachers showed positive views of AI’s effectiveness and ease of use. Both acknowledged its potential to boost learning experiences. Nevertheless, issues arose from AI’s limited ability to understand particular subject matter, its inability to adjust to various educational contexts, and the variation in performance between various AI models. Ethical considerations regarding responsible use also appeared to be an important concern. Addressing these challenges demands a careful approach that considers thorough evaluation and adaptation to diverse contexts. Educators and policymakers should navigate these complexities to join the potential of AI in science education while ensuring ethical practices and maximizing its impact on students’ learning journey worldwide.
Adams, C., Pente, P., Lemermeyer, G., Turville, J., & Rockwell, G. (2022). Artificial intelligence and teachers’ new ethical obligations. The International Review of Information Ethics , 31 (1). https://doi.org/10.29173/irie483 .
Akgun, S., & Greenhow, C. (2021). Artificial intelligence in education: Addressing ethical challenges in K-12 settings. AI and Ethics , 2 , 431–440. https://doi.org/10.1007/s43681-021-00096-7 .
Article Google Scholar
Aldabe, I., & Maritxalar, M. (2014). Semantic similarity measures for the generation of science tests in basque. IEEE Transactions on Learning Technologies , 7 (4), 375–387. https://doi.org/10.1109/TLT.2014.2355831 .
Al Darayseh, A. (2023). Acceptance of artificial intelligence in teaching science: Science teachers’ perspective. Artificial Intelligence , 4 , 100132. https://doi.org/10.1016/j.caeai.2023.100132 .
Alharbi, S. M., Elfeky, A. I., & Ahmed, E. S. (2022). The effect of e-collaborative learning environment on development of critical thinking and higher order thinking skills. Journal of Positive School Psychology , 6 (6), 6848–6854.
Google Scholar
Almasri, F. (2021). Collaborative learning in science education: effects of student’s gender attitudes and achievement in science education University of Warwick, Retrieved from http://webcat.warwick.ac.uk/record=b3853208 .
Almasri, F. (2022a). The impact of e-learning, gender-groupings and learning pedagogies in biology undergraduate female and male students’ attitudes and achievement. Education and Information Technologies , 27 , 8329–8380. https://doi.org/10.1007/s10639-022-10967-z .
Almasri, F. (2022b). Simulations to teach science subjects: Connections among students’ engagement, self-confidence, satisfaction, and learning styles. Education and Information Technologies , 27 (5), 7161–7181. https://doi.org/10.1007/s10639-022-10940-w .
Almasri, F., Hewapathirana, G. I., Alhashem, F., Daniel, C. E., & Lee, N. (2022). The effect of gender composition and pedagogical approach on major and non-major undergraduates biology students’ achievement. Interactive Learning Environments , 1–33. https://doi.org/10.1080/10494820.2022.2066138 .
Almasri, F., Hewapathirana, G. I., Ghaddar, F., Lee, N., & Ibrahim, B. (2021). Measuring attitudes towards biology major and non-major: Effect of students’ gender, group composition, and learning environment. Plos One , 16 (5), e0251453. https://doi.org/10.1371/journal.pone.0251453 .
Alneyadi, S., & Wardat, Y. J. C. E. T. (2023). ChatGPT: Revolutionizing student achievement in the electronic magnetism unit for eleventh-grade students in Emirates schools. Contemporary Educational Technology , 15 (4), ep448. https://doi.org/10.30935/cedtech/13417 .
Antonenko, P., & Abramowitz, B. (2023). In-service teachers’(mis) conceptions of artificial intelligence in K-12 science education. Journal of Research on Technology in Education , 55 (1), 64–78. https://doi.org/10.1080/15391523.2022.2119450 .
Avelino, F., Wittmayer, J. M., Kemp, R., & Haxeltine, A. (2017). Game-changers and transformative social innovation. Ecology and Society, 22 (4). https://www.jstor.org/stable/26798984
Azcona, D., Hsiao, I. H., & Smeaton, A. F. (2019). Detecting students-at-risk in computer programming classes with learning analytics from students’ digital footprints. User Modeling and User-Adapted Interaction , 29 , 759–788. https://doi.org/10.1007/s11257-019-09234-7 .
Balakrishnan, B. (2018). Motivating engineering students learning via monitoring in personalized learning environment with tagging system. Computer Applications in Engineering Education , 26 (3), 700–710. https://doi.org/10.1002/cae.21924 .
Blikstein, P., Worsley, M., Piech, C., Sahami, M., Cooper, S., & Koller, D. (2014). Programming pluralism: Using learning analytics to detect patterns in the learning of computer programming. Journal of the Learning Sciences , 23 (4), 561–599. https://doi.org/10.1080/10508406.2014.954750 .
Bozkurt, A., Karadeniz, A., Baneres, D., Rodríguez, M. E., & Rodríguez, M. E. (2021). Artificial intelligence and reflections from educational landscape: A review of AI studies in half a century. Sustainability , 13 (2), 800. https://doi.org/10.3390/su13020800 .
Buenaño-Fernández, D., Gil, D., & Luján-Mora, S. (2019). Application of machine learning in predicting performance for computer engineering students: A case study. Sustainability , 11 (10), 2833. https://doi.org/10.3390/su11102833 .
Burton, E., Goldsmith, J., Koenig, S., Kuipers, B., Mattei, N., & Walsh, T. (2017). Ethical considerations in artificial intelligence courses. AI Magazine , 38 (2), 22–34. https://doi.org/10.1609/aimag.v38i2.2731 .
Cathrin, S., & Wikandaru, R. (2023). The future of character education in the era of artificial intelligence. Humanika Kajian Ilmiah Mata Kuliah Umum , 23 (1). https://doi.org/10.21831/hum.v23i1.59741 .
Chiu, T. K., Xia, Q., Zhou, X., Chai, C. S., & Cheng, M. (2023). Systematic literature review on opportunities, challenges, and future research recommendations of artificial intelligence in education. Computers and Education: Artificial Intelligence , 4 , 100118. https://doi.org/10.1016/j.caeai.2022.100118 .
Cochran, K., Cohn, C., Rouet, J. F., & Hastings, P. (2023, June). Improving automated evaluation of student text responses using gpt-3.5 for text data augmentation. In International conference on artificial intelligence in education (pp. 217–228). Springer Nature Switzerland. https://doi.org/10.1007/978-3-031-36272-9_18
Cooper, G. (2023). Examining science education in chatgpt: An exploratory study of generative artificial intelligence. Journal of Science Education and Technology , 32 (3), 444–452. https://doi.org/10.1007/s10956-023-10039-y .
Daher, W., Diab, H., & Rayan, A. (2023). Artificial intelligence generative tools and conceptual knowledge in problem solving in Chemistry. Information , 14 (7), 409. https://doi.org/10.3390/info14070409 .
Dai, Y. (2023). Negotiation of epistemological understandings and teaching practices between primary teachers and scientists about artificial intelligence in professional development. Research in Science Education , 53 (3), 577–591. https://doi.org/10.1007/s11165-022-10072-8 .
Deveci Topal, A., Eren, D., C., & Kolburan Geçer, A. (2021). Chatbot application in a 5th grade science course. Education and Information Technologies , 26 (5), 6241–6265. https://doi.org/10.1007/s10639-021-10627-8 .
Elkhodr, M., Gide, E., Wu, R., & Darwish, O. (2023). ICT students’ perceptions towards ChatGPT: An experimental reflective lab analysis. STEM Education , 3 (2), 70–88. https://doi.org/10.3934/steme.2023006 .
Ferrarelli, P., & Iocchi, L. (2021). Learning newtonian physics through programming robot experiments. Technology Knowledge and Learning , 26 (4), 789–824. https://doi.org/10.1007/s10758-021-09508-3
Figueiredo, M., Esteves, L., Neves, J., & Vicente, H. (2016). A data mining approach to study the impact of the methodology followed in chemistry lab classes on the weight attributed by the students to the lab work on learning and motivation. Chemistry Education Research and Practice , 17 (1), 156–171. https://doi.org/10.1039/C5RP00144G .
Figueiredo, M., & Paixão, F. (2015). O Papel da Observação na Compreensão da Natureza do Conhecimento Científico: Uma Proposta Didática Baseada nas Reações de Combustão. In Proceedings do Encontro Nacional de Educação em Ciências – ENEC 2015 - Ciência com Cultura (pp. 205–209). Lisboa.
Gonzalez, A. J., Hollister, J. R., DeMara, R. F., Leigh, J., Lanman, B., Lee, S. Y., & Wilder, B. (2017). AI in informal science education: Bringing turing back to life to perform the turing test. International Journal of Artificial Intelligence in Education , 27 , 353–384. https://doi.org/10.1007/s40593-017-0144-1 .
Grinnell, F. (2021). Scientific Inquiry, pluralism and complementarity. Scientific Inquiry Pluralism and Complementarity . https://doi.org/10.31235/osf.io/gejwv .
Hermansyah, M., Najib, A., Farida, A., Sacipto, R., & Rintyarna, B. S. (2023). Artificial intelligence and ethics: Building an artificial intelligence system that ensures privacy and social justice. International Journal of Science and Society , 5 (1), 154–168. https://doi.org/10.54783/ijsoc.v5i1.644 .
Hewapathirana, G., & Almasri, F. (2022). Active learning compared with lecture-based pedagogies in gender and socio-cultural context-specific major and non-major biology classes. Handbook of research on active Learning and Student Engagement in Higher Education (pp. 293–319). IGI Global.
Holmes, W., Bialik, M., & Fadel, C. (2023). Artificial intelligence in education . Globethics.
Holme, T. A. (2021). Considering the call to action for science education (Vol. 98, pp. 2739–2740). ACS.
Hong, F., Dou, W., & Chen, S. (2022). Research on the impact of artificial intelligence on government public service quality . Paper presented at the 2022 2nd International Conference on Public Management and Intelligent Society (PMIS 2022).
Ibáñez, M. B., & Delgado-Kloos, C. (2018). Augmented reality for STEM learning: A systematic review. Computers & Education , 123 , 109–123. https://doi.org/10.1016/j.compedu.2018.05.002 .
Irez, S. (2006a). Are we prepared? An assessment of preservice science teacher educators’ beliefs about nature of science. Science Education , 90 (6), 1113–1143. https://doi.org/10.1002/sce.20156 .
Irez, S. J. S. E. (2006b). Are we prepared? An assessment of preservice science teacher educators’ beliefs about nature of science. 90 (6), 1113–1143.
Jiao, P., Ouyang, F., Zhang, Q., & Alavi, A. H. (2022a). Artificial intelligence-enabled prediction model of student academic performance in online engineering education. Artificial Intelligence Review , 55 (8), 6321–6344. https://doi.org/10.1007/s10462-022-10155-y .
Jiao, P., Ouyang, F., Zhang, Q., & Alavi, A. H. (2022b). Artificial intelligence-enabled prediction model of student academic performance in online engineering education. Artificial Intelligence Review , 55 (8), 6321–6344.
Kiemde, S. M. A., & Kora, A. D. (2022). Towards an ethics of AI in Africa: Rule of education. AI and Ethics , 2 (1), 35–40. https://doi.org/10.1007/s43681-021-00106-8 .
Kieser, F., Wulff, P., Kuhn, J., & Küchemann, S. (2023). Educational data augmentation in physics education research using ChatGPT. Physical Review Physics Education Research, 19 (2), 020150.
Koć-Januchta, M. M., Schönborn, K. J., Tibell, L. A., Chaudhri, V. K., & Heller, H. C. (2020). Engaging with biology by asking questions: Investigating students’ interaction and learning with an artificial intelligence-enriched textbook. Journal of Educational Computing Research , 58 (6), 1190–1224.
Kok-Sing, T., & Nichols, K. (Eds.). (2024). Collection: Artificial intelligence in science education. Research in Science Education . https://link.springer.com/collections/dbihehajcd .
Kola, A. J. (2013). Importance of science education to national development and problems militating against its development. American Journal of Educational Research , 1 (7), 225–229. https://doi.org/10.12691/education-1-7-2 .
Kolstø, S. D. (2001). Scientific literacy for citizenship: Tools for dealing with the science dimension of controversial socioscientific issues. Science Education , 85 (3), 291–310. https://doi.org/10.1002/sce.1011 .
Lamb, R., Hand, B., & Kavner, A. (2021). Computational modeling of the effects of the science writing heuristic on student critical thinking in science using machine learning. Journal of Science Education and Technology , 30 , 283–297. https://doi.org/10.1007/s10956-020-09871-3 .
Ledesma, E. F. R., & García, J. J. G. (2017). Selection of mathematical problems in accordance with student’s learning style. International Journal of Advanced Computer Science Applications , 8 (3). https://doi.org/10.14569/IJACSA.2017.080316 .
Lee, H. S., Pallant, A., Pryputniewicz, S., Lord, T., Mulholland, M., & Liu, O. L. (2019). Automated text scoring and real-time adjustable feedback: Supporting revision of scientific arguments involving uncertainty. Science Education , 103 (3), 590–622.
Lee, Y. F., Hwang, G. J., & Chen, P. Y. (2022). Impacts of an AI-based cha bot on college students’ after-class review, academic performance, self-efficacy, learning attitude, and motivation. Educational Technology Research and Development , 70 (5), 1843–1865. https://doi.org/10.1007/s11423-022-10142-8 .
Liu, Y., & Pásztor, A. (2022). Effects of problem-based learning instructional intervention on critical thinking in higher education: A meta-analysis. Thinking Skills and Creativity , 45 , 101069. https://doi.org/10.1016/j.tsc.2022.101069 .
Lucci, S., Kopec, D., & Musa, S. M. (2022). Artificial intelligence in the 21st century: Mercury learning and information .
Maestrales, S., Zhai, X., Touitou, I., Baker, Q., Schneider, B., & Krajcik, J. (2021). Using machine learning to score multi-dimensional assessments of chemistry and physics. Journal of Science Education and Technology , 30 , 239–254. https://doi.org/10.1007/s10956-020-09895-9 .
Mansour, N. (2009). Science-technology-society (STS) a new paradigm in science education. Bulletin of Science Technology & Society , 29 (4), 287–297. https://doi.org/10.1177/0270467609336307 .
Mavroudi, A., Giannakos, M., & Krogstie, J. (2018). Supporting adaptive learning pathways through the use of learning analytics: Developments, challenges and future opportunities. Interactive Learning Environments, 26 (2), 206–220. https://doi.org/10.1080/10494820.2017.1292531
Mirchi, N., Bissonnette, V., Yilmaz, R., Ledwos, N., Winkler-Schwartz, A., & Del Maestro, R. F. J. P. (2020). The virtual operative assistant: An explainable artificial intelligence tool for simulation-based training in surgery and medicine. Plos One , 15 (2), e0229596. https://doi.org/10.1371/journal.pone.0229596 .
Mogea, T. (2022). Students’ critical thinking ability in English teaching and learning. Jurnal Pendidikan Dan Sastra Inggris , 2 (3), 157–171.
Nasution & Education. (2023). Using artificial intelligence to create biology multiple choice questions for higher education. Agricultural and Environmental Education , 2 (1).
Nersa, A. (2020). The coverage of children Rights in Amhara television children’s program
Nguyen, P., Trương, H., Nguyen, P., Bruneau, P., Cao, L., & Wang, J. (2023). Evaluation of Google Bard on Vietnamese High School Biology Examination.
Nja, C. O., Idiege, K. J., Uwe, U. E., Meremikwu, A. N., Ekon, E. E., Erim, C. M., & Umalili, B. (2023). Adoption of artificial intelligence in science teaching: From the vantage point of the African science teachers. Smart Learning Environments , 10 (1), 42. https://doi.org/10.1186/s40561-023-00261-x .
Page, M. J., McKenzie, J. E., Bossuyt, P. M., Boutron, I., Hoffmann, T. C., Mulrow, C. D., & Brennan, S. E. (2021). The PRISMA 2020 statement: An updated guideline for reporting systematic reviews. International Journal of Surgery , 88 , 105906. https://doi.org/10.1136/bmj.n71 .
Piasecki, J., Waligora, M., & Dranseika, V. (2018). Google search as an additional source in systematic reviews. Science and Engineering Ethics , 24 , 809–810.
Popenici, S. A., & Kerr, S. (2017). Exploring the impact of artificial intelligence on teaching and learning in higher education. Research and Practice in Technology Enhanced Learning , 12 (1), 1–13. https://doi.org/10.1186/s41039-017-0062-8 .
Siddaway, A. P., Wood, A. M., & Hedges, L. V. (2019). How to do a systematic review: A best practice guide for conducting and reporting narrative reviews, meta-analyses, and meta-syntheses. Annual Review of Psychology , 70 , 747–770. https://doi.org/10.1146/annurev-psych-010418-102803 .
Tang, K. S., & Cooper, G. (2024). The role of materiality in an era of generative artificial intelligence. Science & Education , 1–16. https://doi.org/10.1007/s11191-024-00508-0 .
Wahyono, I. D., Fadlika, I., Asfani, K., Putranto, H., Hammad, J., & Sunarti. (2019). New adaptive intelligence method for personalized adaptive laboratories. In 2019 International conference on electrical, electronics and information engineering (ICEEIE) (pp. 196–200). https://doi.org/10.1109/ICEEIE47180.2019.8981477
Zawacki-Richter, O., Marín, V. I., Bond, M., & Gouverneur, F. (2019). Systematic review of research on artificial intelligence applications in higher education–where are the educators? Journal of Educational Technology in Higher Education , 16 (1), 1–27. https://doi.org/10.1186/s41239-019-0171-0 .
Zhai, X., Haudek, C., Shi, K., Nehm, L. H., R., & Urban-Lurain, M. (2020a). From substitution to redefinition: A framework of machine learning‐based science assessment. Journal of Research in Science Teaching , 57 (9), 1430–1459. https://doi.org/10.1002/tea.21658 .
Zhai, X., He, P., & Krajcik, J. (2022). Applying machine learning to automatically assess scientific models. Journal of Research in Science Teaching , 59 (10), 1765–1794. https://doi.org/10.1002/tea.21773 .
Zhai, X., Shi, L., & Nehm, R. H. (2021). A Meta-analysis of machine learning-based Science assessments: Factors impacting machine-human score agreements. Journal of Science Education and Technology , 30 (3), 361–379. https://doi.org/10.1007/s10956-020-09875-z .
Zhai, X., Yin, Y., Pellegrino, J. W., Haudek, K. C., & Shi, L. (2020b). Applying machine learning in science assessment: A systematic review. Studies in Science Education , 56 (1), 111–151. https://doi.org/10.1080/03057267.2020.1735757 .
Zulyusri, Z., Elfira, I., Lufri, L., & Santosa, T. A. (2023). Literature study: Utilization of the PjBL model in science education to improve creativity and critical thinking skills. Jurnal Penelitian Pendidikan IPA , 9 (1), 133–143. https://doi.org/10.29303/jppipa.v9i1.2555 .
Download references
Acknowledgements
Author information, authors and affiliations.
College of Engineering and Sciences, International University of Science and Technology in Kuwait, Ardiya, Kuwait
Firas Almasri
Department of Physics, Toronto Metropolitan University, Toronto, ON, M5B 2K3, Canada
Centre for Education Studies, University of Warwick, Coventry, United Kingdom
Institute for Biomedical Engineering, Science and Technology (iBEST), A Partnership Between Toronto Metropolitan University and St. Michael’s Hospital, Toronto, ON, M5B 1T8, Canada
You can also search for this author in PubMed Google Scholar
Corresponding author
Correspondence to Firas Almasri .
Ethics declarations
Consent to publish.
I consent to publish identifiable details within the text in the Research in Science Education Journal.
Conflict of Interest
The authors reported no potential conflict of interest.
Additional information
Publisher’s note.
Springer Nature remains neutral with regard to jurisdictional claims in published maps and institutional affiliations.
Rights and permissions
Open Access This article is licensed under a Creative Commons Attribution 4.0 International License, which permits use, sharing, adaptation, distribution and reproduction in any medium or format, as long as you give appropriate credit to the original author(s) and the source, provide a link to the Creative Commons licence, and indicate if changes were made. The images or other third party material in this article are included in the article’s Creative Commons licence, unless indicated otherwise in a credit line to the material. If material is not included in the article’s Creative Commons licence and your intended use is not permitted by statutory regulation or exceeds the permitted use, you will need to obtain permission directly from the copyright holder. To view a copy of this licence, visit http://creativecommons.org/licenses/by/4.0/ .
Reprints and permissions
About this article
Almasri, F. Exploring the Impact of Artificial Intelligence in Teaching and Learning of Science: A Systematic Review of Empirical Research. Res Sci Educ (2024). https://doi.org/10.1007/s11165-024-10176-3
Download citation
Accepted : 07 June 2024
Published : 27 June 2024
DOI : https://doi.org/10.1007/s11165-024-10176-3
Share this article
Anyone you share the following link with will be able to read this content:
Sorry, a shareable link is not currently available for this article.
Provided by the Springer Nature SharedIt content-sharing initiative
- Artificial intelligence
- Science education
- Engineering students
- STEM learning
- Science learning
- Find a journal
- Publish with us
- Track your research
- Comment Comments
- Save Article Read Later Read Later
How AI Revolutionized Protein Science, but Didn’t End It
June 26, 2024

How does a one-dimensional string of molecules fold correctly into its innate three-dimensional shape? This question, known as the protein folding problem, was recently solved by artificial intelligence.
Fran Pulido for Quanta Magazine
Introduction
In December 2020, when pandemic lockdowns made in-person meetings impossible, hundreds of computational scientists gathered in front of their screens to watch a new era of science unfold.
They were assembled for a conference, a friendly competition some of them had attended in person for almost three decades where they could all get together and obsess over the same question. Known as the protein folding problem, it was simple to state: Could they accurately predict the three-dimensional shape of a protein molecule from the barest of information — its one-dimensional molecular code? Proteins keep our cells and bodies alive and running. Because the shape of a protein determines its behavior, successfully solving this problem would have profound implications for our understanding of diseases, production of new medicines and insight into how life works.
At the conference, held every other year, the scientists put their latest protein-folding tools to the test. But a solution always loomed beyond reach. Some of them had spent their entire careers trying to get just incrementally better at such predictions. These competitions were marked by baby steps, and the researchers had little reason to think that 2020 would be any different.
They were wrong about that.
That week, a relative newcomer to the protein science community named John Jumper had presented a new artificial intelligence tool, AlphaFold2, which had emerged from the offices of Google DeepMind, the tech company’s artificial intelligence arm in London. Over Zoom, he presented data showing that AlphaFold2’s predictive models of 3D protein structures were over 90% accurate — five times better than those of its closest competitor.
In an instant, the protein folding problem had gone from impossible to painless. The success of artificial intelligence where the human mind had floundered rocked the community of biologists. “I was in shock,” said Mohammed AlQuraishi , a systems biologist at Columbia University’s Program for Mathematical Genomics, who attended the meeting. “A lot of people were in denial.”
But in the conference’s concluding remarks, its organizer John Moult left little room for doubt: AlphaFold2 had “largely solved” the protein folding problem — and shifted protein science forever. Sitting in front of a bookshelf in his home office in a black turtleneck, clicking through his slides on Zoom, Moult spoke in tones that were excited but also ominous. “This is not an end but a beginning,” he said.

Quanta Magazine ; source: RCSB PDB
Proteins are molecules that come in hundreds of millions of different shapes. Each one serves a particular biological function, from carrying oxygen through the blood to sparking chemical reactions. The function is typically defined by its shape or structure.
When Google’s public relations machine churned the news out to the world, the media went wild. Headlines claimed that AlphaFold2 “ will change everything .” Protein biologists who had spent their entire careers investigating the structures of single proteins feared that they would lose their jobs. Some claimed that AlphaFold2 would revolutionize drug development; now that biologists could quickly learn proteins’ shapes, they could create new medicines that could target them. Others pushed back, arguing that the results were mostly hype and little would change.
Moult could barely comprehend the news himself. He ended the conference with the question on everyone’s mind: “What now?”
That was three and a half years ago. It’s finally possible to start answering his question.
AlphaFold2 has undeniably shifted the way biologists study proteins. However, while AlphaFold2 is a powerful prediction tool, it’s not an omniscient machine. It has solved one part of the protein folding problem very cleverly, but not the way a scientist would. It has not replaced biological experiments but rather emphasized the need for them.
Perhaps AlphaFold2’s biggest impact has been drawing biologists’ attention to the power of artificial intelligence. It has already inspired new algorithms, including ones that design new proteins not found in nature; new biotech companies; and new ways to practice science. And its successor, AlphaFold3, which was announced in May 2024 , has moved to the next phase of biological prediction by modeling the structures of proteins in combination with other molecules like DNA or RNA.
“It’s the biggest ‘machine learning in science’ story that there has been,” AlQuraishi said.
However, there are still massive gaps that artificial intelligence hasn’t filled. These tools can’t simulate how proteins change through time or model them in the context in which they exist: within cells. “AlphaFold changed everything and nothing,” said Paul Adams , a structural biologist who develops algorithms to model the structures of biomolecules at Lawrence Berkeley National Laboratory.
This is the story of how Jumper’s team at Google DeepMind pulled off their coup in protein science, and what it means for the future of artificial intelligence in biology.
Formulating the Problem
A piece of origami paper is little more than pressed wood pulp until it’s folded in specific ways; then it becomes something new. A few precise crimps and flips, and it’s a fortune teller, a paper device that can predict your future. Take the same piece of paper, change a few of the folding steps, and now it’s a winged crane, granting good fortune to its recipient.
Similarly, a long string of amino acid molecules has no function until it spontaneously folds into its innate shape, which biologists call its structure. A protein’s structure determines how it binds to or otherwise interacts with other molecules, and therefore defines its role in a cell.
Mark Belan for Quanta Magazine
There are a couple hundred million known proteins on the planet and many more unknown ones. They do it all: Hemoglobin and myoglobin ferry oxygen around the muscles and body. Keratin gives structure to hair, nails and skin. Insulin enables glucose to move into cells to be converted into energy. Proteins can take on a seemingly infinite number of shapes to match the seemingly infinite number of jobs they do in life.
“Right from the atom all the way to ecosystems, [protein structure] is kind of a lingua franca,” AlQuraishi said. “It’s where everything happens.”
A cell makes proteins by daisy-chaining small molecules called amino acids into long polypeptide strings. The amino acids it chooses depends on the underlying set of instructions provided to it by DNA. Within a fraction of a second of its creation, a polypeptide string bends, buckles and folds precisely into the protein’s final three-dimensional shape. Once off the molecular assembly line, it scurries along to do its biological work.
If proteins didn’t perform this folding process exceedingly well, cascades of disasters would tumble through the body. An incorrectly folded or unraveled protein can lead to toxicity and cell death. Many diseases and disorders, such as sickle cell anemia, are caused by misfolded proteins. And misfolded proteins can aggregate into clumps that are hallmarks of neurodegenerative diseases like Alzheimer’s and Parkinson’s.
Yet no one really knows specifically how protein folding happens. How does the sequence information in these simple molecular chains encode a protein’s complex shape? This is the “most profound question that we can ask,” said George Rose , a biophysics professor emeritus at Johns Hopkins University.

In the 1950s, the biochemist Christian Anfinsen conducted experiments which suggested that a string of amino acids contains an internal code telling it how to fold into a protein — and that there should be a way to predict the protein’s shape from that code. This hypothesis is known as Anfinsen’s dogma.
PBH Images/Alamy
Scientists were probing this question as far back as the 1930s. But efforts really took off in the mid-1950s when the biochemist Christian Anfinsen added proteins to chemical solutions that either unfolded them by breaking their bonds or caused them to fold incorrectly. Then he observed what they did next. Anfinsen saw that the unfolded or misfolded proteins could spontaneously refold into their correct structures. His findings, which later won him a Nobel Prize , demonstrated that proteins form their 3D shapes from an internal code — the one written by their string of amino acids.
Anfinsen hypothesized that there should, therefore, be a way to predict a protein’s shape from the sequence of its amino acids. This became generally known as the protein folding problem.
Once its polypeptide chain is assembled, a protein can fold into its structure within a thousandth of a second — a timescale that perplexed the molecular biologist Cyrus Levinthal. In his 1969 paper “ How to Fold Graciously ,” Levinthal calculated that if a protein were to try out every possible folding option, it would take an impossibly long time to assemble. Clearly, he mused, something must send the protein down the right folding pathway more directly.
Over time, the protein folding problem has forked into new kinds of problems. Now three main questions are posed: Can a protein’s structure be predicted from its amino acid sequence? What is the folding code? And what is the folding mechanism?
These questions started to burrow into scientists’ brains in the early 1960s when the first experimentally determined protein structures became available. Max Perutz and John Kendrew, two biologists at the University of Cambridge, grew proteins into crystals, bombarded them with X-rays and measured how the rays bent — a technique known as X-ray crystallography. By doing so, they determined the 3D structures of hemoglobin and myoglobin. It took them more than two decades. They shared a Nobel Prize for their discoveries.

John Kendrew (left) and Max Perutz (right) meticulously uncovered the structures of hemoglobin and myoglobin using X-ray crystallography. Then they built physical models using balls (atoms) and sticks (chemical bonds).
MRC Laboratory of Molecular Biology
Since then, innumerable researchers have strived to understand not just what different proteins look like, but how they come to look that way. “It’s a very human thing to want to know what things look like, because then you can understand how they function,” said Helen Walden , a structural biologist at the University of Glasgow. Some looked into the chemistry of the problem, others into the physics. Experimentalists reconstructed protein shapes through painstaking lab work. Computational biologists hunted for clues with models and simulations, which they programmed and reprogrammed with different combinations of algorithmic rules.
As more structures came along, the protein science community needed a way to organize and share them. In 1971, the Protein Data Bank was founded as an archive for protein structures. Freely available, the data bank became a dependable tool for anyone who needed to know the structure of a protein to probe a biological question.
When the Protein Data Bank opened, it held the structures of seven proteins. By the time Google DeepMind used it to train AlphaFold2 nearly 50 years later, it held more than 140,000 — each laboriously decoded by the scientists known as structural biologists.
The Experimentalists’ Agony
Starting in the mid-1970s, every few months Janet Thornton reliably received a package in the mail. Inside was a 12-inch magnetic tape containing data about new protein structures deposited in the Protein Data Bank. A biophysicist at the University of Oxford, Thornton would eagerly rip open the package so she could analyze the new structures nearly as soon as they were discovered. The first tape Thornton received had only 20 structures on it.

“I had many students who said, ‘I want to come and solve the protein folding problem,’” said Janet Thornton, a structural biologist who retired from the European Molecular Biology Laboratory last year. “But I didn’t have any new ideas, frankly, about how to do that.”
Jeff Dowling, EMBL-EBI
Every one of those proteins represented years of work. Often a doctoral candidate would spend their four or more years in graduate school crystallizing a single protein, collecting data from it or interpreting that data to figure out the folded structure.
Oxford’s department of biophysics was, at the time, one of the world’s centers for X-ray crystallography. There, in 1965, David Phillips, one of the pioneers of protein crystallography, first determined the structure of an enzyme: lysozyme, which the immune system uses to attack bacteria. Using X-ray crystallography, Oxford biophysicists created maps of proteins’ electron density; the areas in which electrons congregated were likely to contain an atom. Thornton and her colleagues printed these electron density maps onto plastic sheets and stacked them one on top of another to create a “contour map” of the protein’s geography, she said.

X-ray crystallography helps scientists build electron density maps, which visualize where electrons congregate and therefore where atoms likely sit in a molecule. By stacking the maps on top of one another (left), scientists can deduce the structure of a protein or another molecule like penicillin (right).
Science Museum Group
Then they converted the maps into physical models. They placed their plastic maps into a Richards box, named for the Oxford biophysicist Frederic Richards, who invented the device in 1968. Inside a Richards box, an angled mirror reflected the maps into a workspace, allowing the scientists to see exactly where each atom was located relative to others. Then they physically built their model out of balls and sticks.
This method was cumbersome and restrictive. In 1971, Louise Johnson, who would go on to become an eminent crystallographer, was modeling phosphorylase, which at 842 amino acids was at the time the largest protein anyone had worked on. To model it, Johnson had to climb a ladder into a two-story Richards box, which Oxford constructed especially for her project.
Once a model was complete, scientists used a ruler to measure the distances between atoms to come up with coordinates for the protein structure. “It was archaic,” Thornton said. Then they fed the coordinates into a computer. The computerized version looked like a dense forest, she said, with atoms clumped together in a jumble. Only when Thornton looked at the structure through 3D glasses could she start to see the protein’s topology.
“It was a very torturous process,” Thornton said. “It’s amazing that it got done.”
Year by agonizing year, they did it. Once researchers were confident in their protein structure, they submitted it to the Protein Data Bank. By 1984, 152 proteins had been deposited. In 1992, that number climbed to 747.
While the experimentalists toiled on with their physical models, another faction of protein biologists — the computational scientists — took a different approach. But as they pondered Anfinsen’s insight that a protein’s structure should be predictable from its amino acid sequence, they got a bit overconfident.
Writing Their Own Rules
As an undergraduate in the early 1960s, John Moult planned to become a physicist. Then he learned about the protein folding problem. “Somebody came and gave a lecture about biology being too important to leave to the biologists,” he said, “which I arrogantly took seriously.” Captivated, he took his career in a different direction.
After he graduated, Moult went into protein crystallography. He decoded the structures of several proteins, including beta-lactamase, a bacterial enzyme that destroys penicillin, and received his doctorate in molecular biophysics at Oxford in 1970. But as he started his postdoc, he tired of the experimentalist approach and began to drift toward the growing field of protein computation. Computational biologists, as opposed to experimentalists, wrote computer algorithms to try and prove that Anfinsen was right: that they could feed a program a string of amino acids to generate a correct protein structure.

John Moult co-founded the Critical Assessment of Structure Prediction (CASP) experiment to force himself and other computational biologists to test their computer models of proteins against experimentally determined protein structures.
Umit Gulsen for Quanta Magazine
The transition from biological experiments to computation was an uneasy one. Moult was used to the slow, careful work of solving a single protein structure. In his new field, computational papers regularly claimed to have solved the protein folding problem and related sub-problems.
Moult was dubious. “The things that were being published in that area were not as rigorous as I was used to,” he said. “This is not because we’re all a load of crooks in this field. It’s because if you’re doing this sort of computational work, you’re doing it in a virtual world.”
In a virtual world, computationalists wrote their own rules when the rules of the natural world didn’t work. They designed their algorithms so that atoms stuck together in a certain way or the protein always folded to the right or the left. Over time, the models drifted farther away from reality. It’s hard to maintain rigor in a world where you have complete control, Moult said.
Still, he could see the value of both sides. Experimentalists worked precisely but slowly; computationalists worked quickly but were so removed from biophysical realities that they were often wrong.
There must be a way, he thought, to bring the best of both approaches together.
The Stamping Begins
In the early 1990s, Moult and his colleague Krzysztof Fidelis had an idea for bringing discipline to the field’s chaos. They set up a community science experiment that they called the Critical Assessment of Structure Prediction, or CASP.
The idea was simple. As CASP’s organizers, Moult and Fidelis would publish a list of amino acid sequences for proteins whose structures had been recently solved and supplied to them by experimentalists, but for which the results hadn’t yet been published. Then computational groups around the world would try their best to predict the protein’s structure using whatever method they wanted. An independent group of scientists would assess the models by comparing their answers to the experimentally confirmed structures.
The idea took off. CASP soon became a proving ground for computational approaches to the protein folding problem. These were the days before artificial intelligence, when computational approaches involved simulating molecular physics. It was a chance for scientists to put their thinking to the test in a public trial against their peers. “It wasn’t supposed to be a competition,” Thornton said. “But it actually has turned out to be a competition.”
Every two years, scientists gathered at the Asilomar conference center, an old chapel near Monterey, California, that used to be a Methodist retreat. During these conferences, the organizers announced the competition’s results and the computationalists gave talks about their methods and approaches. Moult encouraged attendees to stamp their feet on the wooden floors if they didn’t like what they were hearing.
“There was, at the beginning, quite a lot of stamping,” he said.
It was “almost like a drum,” recalled David Jones , a professor of bioinformatics at University College London who studied under Thornton. The biologists stamped if the talks got bogged down in details. They stamped if claims were overblown. They stamped if speakers were repetitive or too much in the weeds. But it was friendly stamping, Jones said: “It wasn’t nasty.”

The early CASP conferences were held at the Asilomar conference center in Monterey, California. When attendees stamped on the wooden floors, it sounded like a drum.
Aramark Destinations
Whatever the reason, when the echoes of stamping started ringing in a speaker’s ears, it was embarrassing. “Thank God I never got stamped on,” Jones said. One year, he and his colleagues presented a computational method called threading, in which amino acid sequences were woven through known protein structures to search for a fit. They didn’t do too badly. “We were quite pleased. … It was all downhill after that,” Jones recalled, laughing. “No, it was fun.”
There was a lot of excitement back then, said Silvio Tosatto , a professor of bioinformatics at the University of Padua. “People thought that they could become millionaires because they had the right algorithm, and some other people thought that they would immediately win the Nobel Prize.”
Neither of those things happened during the early years. When asked what the CASP submissions were like during that time, Moult paused. “‘Random’ is a good word,” he said.
Some methods performed better than expected, such as “homology modeling,” which compared the structures of known proteins to deduce the structures of unknown ones. Others were a dead loss. Most structure predictions were “tortured-looking objects,” Moult said.
“I was loving seeing them fail,” joked Anastassis Perrakis , a structural biologist at the Netherlands Cancer Institute and Utrecht University who gave experimentally determined structures to CASP organizers for use in the competition. “It’s not rivalry, but we like to tease each other in science.”
Through this process, clear leaders emerged. In 1996, after the second CASP wrapped up, a young man named David Baker asked Jones to share a taxi to the airport. Baker had seen Jones’ talk and was working on his own computational model. He didn’t have it ready for this CASP, but he wanted to chat about it. Jones listened to his ideas in the cab and never expected to see him again.
At the next competition in 1998, Baker blew the doors wide open with his algorithm Rosetta. He became “the man to beat,” Jones said.

David Baker, who is now one of the world’s leading protein design experts, was the man to beat at CASP with his high-performing algorithm named Rosetta.
BBVA Foundation
Algorithms like Rosetta modeled interactions between the atoms of amino acid molecules to predict how they would fold. They “showed that you actually could predict protein structure,” Baker said. “But it wasn’t good enough or accurate enough to be useful.”
In 2008, humans were still beating the computers. Baker , who by then was running his own lab at the University of Washington, created a free online computer game called Foldit , in which players folded strings of amino acids into protein structures. In a paper published in Nature , his team reported that human Foldit players outperformed Rosetta in modeling proteins.
But the human lead wouldn’t last long. In the early 2010s, important breakthroughs in a concept known as co-evolution propelled the field forward and would later turn out to be critical for artificial intelligence. The idea, which had been around for decades, was straightforward: By comparing closely related sequences of amino acids in hundreds to thousands of proteins, scientists could identify the amino acids that had mutated — and, importantly, determine whether they’d mutated in step with others. If two amino acids changed together, they were likely linked in some way. “You start to be able to say, ‘Well, these two things are probably close together in space,’” said Adams, the structural biologist at Berkeley Lab.
But until the early 2010s, such predictions of which amino acids were in contact were dismal. Their accuracy hovered between 20% and 24%. Then scientists noticed that their statistical methods were introducing errors, suggesting that some amino acids were in contact when they weren’t. Later, Moult learned that statisticians had been keenly aware of this kind of error for decades. When you looked back, he said, you’d think, “How could I be so stupid?”
Computational biologists cleaned up the statistical tools . By 2016, the accuracy of contact prediction had shot up to 47%. Two years later, it reached 70%. Baker’s algorithm built on this success: In 2014, Rosetta produced two protein structures so accurate that a CASP assessor thought Baker might have solved the protein folding problem.
The co-evolution insights were “fantastic,” Adams said. Without using machine learning, co-evolution was “one of the big things that came that really pushed that field forward.”
However, it got the field only so far. Co-evolution required an abundance of similar proteins to compare, and experimentalists weren’t solving protein structures fast enough to supply computationalists’ needs.
The years flowed by in a punctuated equilibrium, Moult said, using a term from evolutionary biology. Sometimes it felt as though no good ideas had evolved for a billion years — and then something exciting would happen.
Off the Deep End
In 2016, David Jones caught a glimpse of the future in a new paper in Nature . Researchers from Google DeepMind, an artificial intelligence team based in London, detailed how their algorithm, which used a method known as deep learning, had beaten a human champion at an ancient board game called Go.
Jones was amazed. “Things are happening,” he recalled thinking at the time. “I’m really going to have to get into this deep learning.”
Deep learning is a flavor of artificial intelligence loosely inspired by the human brain. In your brain, molecular information is sent across an interconnected web of brain cells called neurons. Neurons have little arms called dendrites that grab molecules dispatched by neighboring neurons which tell the receiving neuron either to fire and propagate a signal or not to fire.
“If enough activity comes at that neuron, then that neuron is going to fire,” said Michael Littman , a computer science professor at Brown University. That results in another wave of molecules being released to the next neuron.
In the 1950s, computer scientists realized that they could wire electronic bits together to create “neural networks.” Every unit in the neural network is a node, which researchers likened to a neuron: A neuron receives information from other neurons, then calculates whether to fire toward the next ones. In neural networks, information propagates across multiple layers of neurons to produce a particular outcome, like recognizing a dog in an image.
The more layers of neurons you have, the more intricate calculations you can perform. But early neural networks were made up of only two layers. In the 1990s, that number increased to three, and it stayed there for two decades. “We could not figure out how to reliably create networks that were deeper than that,” Littman said.
Structural biologists, including Jones and Moult, had tried using neural networks in protein science since the 1990s, but the limitations of shallow networks and sparse data held them back. Then, in the early 2010s, computer scientists learned how to better structure neural networks to allow reliable training of more layers. Networks deepened to 20, 50, 100 and then thousands of layers. “To distinguish that from the way we were doing it in the ’90s, people started to call it ‘deep learning,’” Littman said. “Because if machine learning people are good at one thing, it’s making up sexy names.”
Deep learning transformed artificial intelligence, leading to algorithms that excelled at recognizing features in photos or voices — and, it turns out, at beating humans in games.
In March 2016, when DeepMind co-founder Demis Hassabis was in Seoul watching his AI system AlphaGo beat a human world champion in the ancient game of Go, he flashed back to playing Foldit as an undergraduate. He wondered: If DeepMind researchers could write an algorithm to mimic the intuition of Go masters , couldn’t they write one to mimic the intuition of Foldit gamers, who knew nothing about biology but could fold proteins?

In 2016, AlphaGo, an AI system by Google DeepMind, defeated Lee Sedol (right), the world champion in the ancient game of Go. Its ability to mimic human intuition drew biologists’ attention to the potential power of deep learning in protein science.
Google DeepMind
Jinbo Xu , a professor at the Toyota Technological Institute at Chicago, also recognized the potential of using deep learning to attack the protein folding problem. He was inspired by what these networks were doing in image recognition. By then, computer scientists had had great success with convolutional networks, which program deep learning algorithms to break images into pieces and identify patterns between them. Xu brought this technique to protein folding. He used a mathematical object called a matrix to represent which amino acids were close together in space, then fed the data into a convolutional network as an image. The algorithm looked for patterns among these images to predict the 3D coordinates of the atoms that make up a protein.
In 2016, Xu posted a preprint of this work on arxiv.org (it was later published in PLOS Computational Biology ) that “was quite influential” for the field, Moult said. It showed people “the sort of things you might do with deep learning.”
Before long, protein structure groups started dabbling in deep learning. AlQuraishi and his team were the first to develop an approach that could directly predict protein structure exclusively with neural networks, in what’s called an “end-to-end” method — it just didn’t work very well. Others wondered how they could dip their toes into a new approach that felt so momentous.
“I didn’t know exactly what I wanted to do with deep learning, but I realized I needed to be doing deep learning,” Jones said.
He had started to write grant applications to find his way in when he received an email from Google DeepMind. They asked Jones about the CASP competition and offered help. “I just assumed they meant: We’ve got lots of computer power,” Jones said.
After he met them, it became obvious that Google had larger ambitions. But to pull them off, the tech giant would need more scientific brainpower.
A New Player on the Field
In 2016, when Jones started working as a consultant for Google DeepMind on a project that would later be known as AlphaFold, John Jumper was completing his doctorate in theoretical chemistry at the University of Chicago.
As a teenager, Jumper had taught himself how to program computers. He also had a knack for physics. So when it came time to go to college, he decided to study math and physics even though his parents, both engineers, were worried he’d never be able to find a job.
“I thought I was going to be a ‘laws of the universe’ physicist all the way through,” Jumper said. “I’ve always loved this notion of discovering something true of the universe.”
As an undergraduate at Vanderbilt University, he joined a collaboration with researchers at the Fermi National Accelerator Laboratory to study the strange properties of subatomic particles called quarks . One day, when he was sitting at a lunch table with the researchers, he got some sour news. “So, this experiment that we’re working on — when’s it going to turn on?” Jumper recalled asking them. One of the professors said that he’d probably retire first. The other, a bit older, said he might not live to see it.
“I wanted to do science in a little shorter timeframe than that,” Jumper said. After finishing undergrad, he started a doctoral program in theoretical condensed matter physics — and quickly dropped out. He had gotten a job at D.E. Shaw Research, a New York company that, at the time, was creating basic simulations of proteins. By understanding how proteins move and change, they hoped to better understand the mechanisms of various ailments like lung cancer.
It was the first time Jumper grasped the potential significance of his work. “It’s about health and extending people’s lives,” he said. For the next three years, Jumper modeled protein movements on the company’s supercomputers, which they had built specifically to simulate molecules faster. “I was doing more simulation on a Tuesday of some weeks than I was going to do in my entire Ph.D.,” he said.
In 2011, he gave graduate school another shot, this time studying theoretical chemistry at the University of Chicago. He was still interested in protein structure and movement. But he was frustrated by the slow pace of academia. “I no longer had this access to this custom computer hardware” he had used at D.E. Shaw, Jumper said. He wondered if he could use artificial intelligence — “at the time we called it statistical physics” — to reach a level of quick simulation that otherwise required advanced machines. He began dabbling in machine learning and neural networks.
During this time, he also started thinking about the protein folding problem. He suspected that the problem should be solvable with the training data available in the Protein Data Bank — by 2012, it contained more than 76,000 protein structures.
“I believed that the data were sufficient,” Jumper said. But “the ideas weren’t.”
In 2017, Jumper heard a rumor that Google DeepMind was getting into protein structure prediction. He had just completed his doctorate, using machine learning to simulate protein folding and dynamics. He applied for a job as a research scientist.
“The project was still secret,” he said. If he raised the subject of protein folding in an interview, the DeepMind team changed the subject. “You can only do that so many times before I’m pretty sure what you’re doing,” Jumper said.
In October 2017, he arrived at DeepMind’s London office. With Jones’ help as a consultant, the team was already deep into the development of AlphaFold. “It was a great fun time where we were just throwing ideas at the wall,” Jones said. “Eventually, a good core idea emerged, and they ran with it.”
To train their algorithm, the DeepMind team used more than 140,000 structures from the Protein Data Bank. They fed this information into a convolutional network, but didn’t change much about the AI architecture itself. It was “standard machine learning,” Jumper said.
By the spring of 2018, AlphaFold was ready to join CASP and compete against bona fide protein scientists. “It’s a bit like Formula One racing,” Jones reflected. “You think you’ve built the best car, but you just don’t know what the other teams have built.” The stakes felt high. The DeepMind team debated whether they should compete anonymously; they didn’t want to risk humiliation.
“Nobody wants to fail,” Jones said. In academia, it’s part of the job; you fail, and you move on because you don’t have a choice. “But obviously if you’re a multibillion-dollar tech company, it’s not a good look if you tried to do something and failed.”
They ultimately decided to submit their results under the Google DeepMind name. A few months before the December meeting, Jones heard from CASP’s organizers. They suggested that the DeepMind team come along to the meeting because AlphaFold had performed really well.
Their victory wasn’t massive — they were about 2.5 times better at predicting protein structures compared to the next-best team — but their win made an impression. “It was clear something interesting had happened,” Moult said.
Rebooting the Algorithm
The win should have energized the DeepMind team. But they knew they weren’t close to solving the protein folding problem. Hassabis had gathered them a few months earlier. “Are we going to go after solving this or not?” Jumper recalled him saying. “If not, let’s find problems that we can have this really, really big impact on.”
“We had this moment where we really decided: We are going to go after solving it,” Jumper said. They went back to the drawing board.

John Jumper suspected that biologists had studied enough protein structures to solve the protein folding problem. “I believed that the data were sufficient,” said Jumper, who started working at Google DeepMind in 2017. But “the ideas weren’t.”
With his diverse background in physics, chemistry, biology and computation, Jumper brought original insights to brainstorming sessions. Soon, he was leading the team, which had grown from six to 15 people. “There was something very unique going on,” said Raphael Townshend, who interned at Google DeepMind in 2019 and later founded Atomic AI, an AI-driven biotech company.
In academia, experts are often siloed from one another, each pursuing independent projects with little collaboration. At DeepMind, experts in statistics, structural biology, computational chemistry, software engineering and more worked together on the protein folding problem. They also had the massive financial and computational resources of Google behind them. “Things that would have taken me months to do as a Ph.D. student, I was doing in a single day,” Townshend said.
The London DeepMind office was high-energy, he said, and much of that energy was generated by Jumper. “He’s a true genius, I would say, and also a very humble person,” said the computer scientist Ellen Zhong , who interned at DeepMind in 2021 and is now an assistant professor at Princeton University. “He was beloved by the team.”
Under Jumper’s leadership, AlphaFold was reconstructed. DeepMind designed a new type of transformer architecture — a type of deep learning that has “powered basically every single machine learning breakthrough that’s happened in the last five years,” Townshend said. The neural network tweaked the strength of its connections to create more accurate representations of the data, in this case protein evolutionary and structure data. It ran that data through a second transformer to predict the 3D structure of a protein. The algorithm then honed the structure further by running it, together with some of the revised data, back through its transformers a few more times.
When they first started working on AlphaFold2, their algorithm was “terrible, but not as terrible as we expected,” Jumper said. “[It] made helices that kind of vaguely looked like a protein.” But as they honed it further, they noticed enormous increases in the efficiency and accuracy of their predictions.
“It was actually terrifying,” Jumper said. If it’s working too well, that usually means “you’re doing the wrong thing.” They checked, but there wasn’t a problem. It was simply working.
The team decided to run an internal experiment to see whether their system would be helpful to biologists. They identified roughly 50 papers published in high-end journals like Science , Nature and Cell that not only described a new protein structure but also generated insights about the protein’s function from the structure. They wanted to see if AlphaFold2 would stand up to the experimentalists’ laborious approach.
They entered the amino acid sequences. AlphaFold2 ran its prediction engine. For each sequence, it spat out a prediction close to the experimental structure presented in the papers. However, in the team’s view, it wasn’t accurate enough. The structures were missing key details that the experimentalists learned about their proteins. “You feel like you’ve finished the race and it’s like finding out that you’ve got the second half,” Jumper said.
The team further honed the system over the next six months, minor improvement by minor improvement. A few weeks before the protein candidates were released for the 2020 CASP competition, they performed another usefulness test. Jumper was satisfied. Google DeepMind submitted their predictions to CASP in the spring of 2020. And then they waited.
The Earthquake
In early summer, Moult received an email from a CASP assessor: “Look at this, it’s pretty impressive.” Attached to the email was a protein structure solved by Google DeepMind. Moult was indeed impressed, but he thought it was a one-off.
Then he got another email, and another. “That’s strange,” he recalled thinking. There were three, four, a whole slew of near-perfect protein predictions — and all from DeepMind. By the end of the summer, “we rapidly realized … something very, very extraordinary had happened,” Moult said.
CASP assessors score each submission by comparing the predicted protein structure to its proven experimental structure. A perfect score, in which the model and reality match atom by atom, is 100. Moult had always believed that anything above 90 would indicate that an algorithm had effectively solved a protein’s structure. Most of AlphaFold’s structures hit or surpassed the 90 mark.
A few months before the meeting, Moult called Jumper with the news. “I cursed out loud,” Jumper recalled. “My wife asked if I was OK.”
In December 2020, less than a year into the Covid-19 pandemic, Jumper presented AlphaFold2 over Zoom at the virtual CASP meeting.
Like the rest of the attendees, Jones watched from home. “I was just stuck … watching this unfold,” he said. “There’s no outlet because your colleagues aren’t nearby. … We’re all under lockdown so we can’t go anywhere.”
For anyone who wasn’t a neural network expert, the ideas were complex. Even so, the conclusions were clear. DeepMind had solved the structure prediction part of the protein folding problem. AlphaFold2 could accurately predict the structure of a protein from its amino acid sequence.
“Ugh, my favorite subject is dead,” Jones recalled thinking. “DeepMind shot it, and it’s the end.”
For years, Anastassis Perrakis had contributed unpublished experimental results to CASP for the competition. When he saw AlphaFold2’s results for a protein his team had sweated over, he thought, “Uh-oh.” AlphaFold2 had gotten it perfectly right.
Alone at home in lockdown, the scientists were united in thinking that the world of protein science had changed forever. As its inhabitants looked out across the new landscape, they had one question in mind: What now?
Shock and Awe
Structural biology suddenly became unstructured.
At first there was “a lot of soul searching,” said Silvio Tosatto, who had competed in CASP since its earliest days. Some structural biologists feared their jobs would become obsolete. Others grew defensive and claimed that AlphaFold2 wasn’t accurate.
The computational biologists who had been trying to solve this problem, some for decades, found the moment bittersweet. In a blog post he wrote after CASP, AlQuraishi cited an attendee who described feeling like someone whose child had left home for the first time.

Mohammed AlQuraishi, a systems biologist at Columbia University’s Program for Mathematical Genomics, hopes that deep learning will be able to simulate an entire cell and all the structures and dynamics within it by 2040.
Nicole Pereira
But even amid their trepidation around this shiny new tool, many scientists were ecstatic. Those who didn’t do structural work used to have to collaborate with structural biologists to determine protein structures for their broader experiments. Now, they could just press a few buttons and get the structure on their own.
In the media, AlphaFold2 became the shiny new artificial intelligence breakthrough that would “ change everything .” But it took months and years for scientists to tease apart what AlphaFold2 could and couldn’t do. Around six months after Jumper’s talk, Google DeepMind published their results and shared AlphaFold2’s underlying code. “When AlphaFold2 was out, the next day we were trying to install it in our GPU servers,” Perrakis said. Biologists began to play.
“I expected [AlphaFold2] to fall down,” Thornton said. “But actually my impression is that it’s been remarkably successful.”
It started to become clear that rather than being a threat, AlphaFold2 might be a catalyst for accelerating research. Rather than put structural biologists out of a job, it gave them a new tool to do their jobs better. “If you view a structural biologist simply as a technical specialist who works out the structure of proteins, then yes, of course structural biologists are out of the job,” Walden said. But that would be like saying that the Human Genome Project made genomicists obsolete because they could no longer publish a paper describing the sequence of a single gene.
In many cases, a structural biologist’s goal is to discover the function of a protein. With AlphaFold2, they could create a hypothesis within minutes rather than wait for months or years to work out a structure through experiments.
“This changes structural biology in many good ways, and not bad ways,” Adams said. “This only makes this a more exciting field to work in.”
However, it didn’t immediately result in all kinds of new drugs as some people had predicted — and researchers soon learned that the tool has its limitations. AlphaFold2 predictions aren’t perfect. They require experimental validation, Perrakis said. But “you can move much quicker to the actual study of the structures.” Now when his students start a new project, they first use AlphaFold2 to predict the structure of a particular protein. Then they conduct experiments to validate it.
Perrakis suspects that he and other researchers will continue to use X-ray crystallography to a degree. But to develop initial protein structures, many are starting to combine deep learning predictions with advanced electron microscope techniques such as cryo-EM, which involves flash-freezing biological samples and bombarding them with electrons. Then they can get to the interesting questions about what their proteins do. AlphaFold2 has “turbo-boosted” cryo-EM, AlQuraishi said.
That shift has already begun. In June 2022, a special issue of Science revealed the near-atomic structure of a human nuclear pore complex. This massive, complicated structure — built of 30 different proteins — had been a biological quandary for decades. The scientists used AlphaFold2 predictions to fill in gaps in the proteins’ structures left unsolved by cryo-EM.
Seeing that paper, in which other scientists used AlphaFold2 to make a biological breakthrough, was the “moment that I knew that [AlphaFold] really, really mattered,” Jumper said.
Discoveries like the nuclear pore complex dot the timeline of the last three years of protein science. Already, AlphaFold2 has predicted protein structures that have been used to study diseases and create new tools for drug delivery. “It’s been hugely helpful for us,” said Feng Zhang , a molecular biologist at the Broad Institute who used AlphaFold2 to engineer a molecular syringe to deliver drugs into human cells. Knowing a protein’s structure can also help develop drugs if researchers can identify molecules to latch onto a protein’s shape, for example, and change its behavior. While some studies have suggested that AlphaFold2 predictions aren’t as useful as experimental structures in this realm, others have shown that they work just as well . The full impact of AI tools on drug discovery is still unfolding.
Some biologists, however, are already looking beyond AlphaFold2’s use in discerning the structures and functions of known proteins and toward designing ones that don’t exist in nature — a technique pivotal for designing novel medicines.
The Next Frontier
Almost immediately after seeing Jumper’s talk at the 2020 CASP conference, Baker got back to work on his Rosetta algorithm. Google hadn’t yet shared AlphaFold2’s underlying source code. Still, “we started playing with some of the ideas that they introduced,” Baker said. On the same day that Google DeepMind published AlphaFold2 in Nature , he and his team announced RoseTTAFold , a highly accurate rival to AlphaFold. RoseTTAFold also uses deep learning to predict protein structures but has a very different underlying architecture than AlphaFold2.
“Once the scientific idea is out there, it’s possible for people, at least the ones who have enough resources, to reverse engineer it and try to build on top of that,” Tosatto said.
RoseTTAFold wasn’t alone. Other AlphaFold competitors, including Meta, crafted their own algorithms to address protein structure prediction or related problems. Some, including Townshend’s biotech startup Atomic AI, have expanded beyond proteins to use deep learning to understand RNA structures. However, in the realm of single-structure predictions, no one has been able to match AlphaFold’s accuracy so far, Thornton said. “I’m sure they will, but I think getting another … AlphaFold moment like that will be very difficult.”

Last year, David Baker (pictured here), with John Jumper and Demis Hassabis, received the Frontiers of Knowledge Award in Biology and Biomedicine for their work revolutionizing the study and design of proteins with artificial intelligence.
At least in public, Baker and Jumper have continued the tradition of productive competition established by CASP. “They might feel that I compete with them, but I feel like they’ve just been inspirational for us,” Baker said.
Jumper welcomes it. “It’s really important that people build on this science,” he said. “It would be sad to me if there was no intellectual lineage of AlphaFold.”
Baker is already evolving his program’s lineage to focus on a new frontier in protein science: protein design. Right now, biologists are confined to studying the proteins already invented by nature. Baker envisions a science in which they could design novel proteins — ones crafted specifically to harness sunlight, break down plastic, or form the basis of drugs or vaccines.
“The number of different types of protein structures or shapes in nature at the moment is quite limited,” said Danny Sahtoe , a structural biologist at the Hubrecht Institute in the Netherlands who did his postdoc under Baker. “In theory, more should be possible, and if you can have more shapes, that also means that you can have more functions.”
Protein design is essentially the “inverse protein folding problem,” said Baker, who directs the Institute for Protein Design at the University of Washington. Rather than feed an amino acid sequence to a deep learning algorithm and ask it to spit out a protein structure, a protein designer feeds a structure into an algorithm and asks it to spit out a sequence. Then, using that amino acid sequence, the designer builds the protein in the lab.
AlphaFold and RoseTTAFold by themselves can’t spit out these sequences; they are programmed to do the opposite. But Baker created a design-specific iteration of RoseTTAFold, known as RoseTTAFold diffusion or RF diffusion , based on its neural architecture.
The field of protein design has existed for a long time, but deep learning has accelerated it, Sahtoe said. It makes the process of designing realistic computer models of proteins “incredibly fast.” It used to take weeks or months for trained protein designers to create the backbone of a new protein. Now they can make one in days, sometimes even overnight.

Foldit , an online game developed by Baker’s lab, has players predict protein structures.
Baker also updated Foldit to incorporate his obsession: Instead of building protein structures, players design proteins. It’s been productive. Baker’s lab has written papers on several of the player-designed proteins. One of the world’s top Foldit players is now a graduate student working with one of Baker’s colleagues at the University of Washington.
“Do we understand protein folding? Well, if we can design new sequences that fold up to new structures, then that shows we understand quite a lot about protein folding,” Baker said. “That, in a sense, you could view as a solution to the protein folding problem too.”
Trust Exercise
AlphaFold2’s success has undeniably shifted biologists’ attitudes toward artificial intelligence. For a long time, many experimental biologists distrusted computation. They understood that some machine learning approaches can make data appear better than it is. Then Google DeepMind demonstrated “unequivocally that you could do serious work with this,” AlQuraishi said. Any skepticism is now matched with: “Well, what about AlphaFold?”
“Biologists now believe our prediction results,” said Xu, the computational biologist who advanced convolutional networks. “Before, biologists always suspected if our prediction is reliable.”
Playing into this trust is a feature of the AlphaFold2 platform: It not only generates a 3D model of a protein, but also self-assesses the accuracy of its prediction by grading different parts of the structure on a confidence scale from zero to 100.
In July 2022, after Google DeepMind released the structure predictions of 218 million proteins — nearly all those known in the world — Adams decided to analyze AlphaFold2’s self-reports. He compared the predictions to the proteins’ solved structures and independently assessed their accuracy.
The “good news is that when AlphaFold thinks that it’s right, it often is very right,” Adams said. “When it thinks it’s not right, it generally isn’t.” However, in about 10% of the instances in which AlphaFold2 was “very confident” about its prediction (a score of at least 90 out of 100 on the confidence scale), it shouldn’t have been, he reported: The predictions didn’t match what was seen experimentally.
That the AI system seems to have some self-skepticism may inspire an overreliance on its conclusions. Most biologists see AlphaFold2 for what it is: a prediction tool. But others are taking it too far. Some cell biologists and biochemists who used to work with structural biologists have replaced them with AlphaFold2 — and take its predictions as truth. Sometimes scientists publish papers featuring protein structures that, to any structural biologist, are obviously incorrect, Perrakis said. “And they say: ‘Well, that’s the AlphaFold structure.’”
“Some people are overconfident — like, way overconfident — in what these deep learning models can do,” said Lauren Porter , an investigator at the National Institutes of Health. “We should use these deep learning models for as much as we can, but we also need to approach them with caution and humility.”
Jones has heard of scientists struggling to get funding to determine structures computationally. “The general perception is that DeepMind did it, you know, and why are you still doing it?” Jones said. But that work is still necessary, he argues, because AlphaFold2 is fallible.
“There are very large gaps,” Jones said. “There are things that it can’t do quite clearly.”
While AlphaFold2 is excellent at predicting the structures of small, simple proteins, it’s less accurate at predicting those containing multiple parts. It also can’t account for the protein’s environment or bonds with other molecules, which alter a protein’s shape in the wild. Sometimes a protein needs to be surrounded by certain ions, salts or metals to fold properly.
“At the moment, AlphaFold is a little bit of a ways away from being able to determine context,” Walden said. Her group has determined several structures experimentally that AlphaFold2 couldn’t predict.
There are also several types of dynamic proteins that AlphaFold2 predicts poorly but that are no less important in function. Shape-shifting proteins, also known as fold-switching proteins, are not static: Their shapes change as they interact with other molecules. Some fold into dramatically different shapes, despite having the same amino acid sequence. Fold-switching proteins “challenge the paradigm that sequences encode one structure,” Porter said, “because clearly they don’t.”
Fold-switching proteins like RfaH, shown here, can change conformations to perform different tasks. When in its alpha helix form, the RfAH protein can’t bind to its target — but when it switches its form to a beta sheet, it can.
Courtesy of Lauren Porter
Compared to the hundreds of thousands of static, single-structure proteins that the DeepMind algorithm trained on, there are only about 100 examples of fold-switching proteins — although more surely exist. It’s perhaps no surprise, Porter said, that “generally speaking, these algorithms were made to predict a single fold.”
And then there are the proteins that flail about like an air dancer outside a car dealership. Intrinsically disordered proteins or protein regions lack a stable structure . They wiggle and re-form constantly. “They’ve been in many ways ignored simply because they were a little bit annoying,” said Kresten Lindorff-Larsen , a professor of computational protein biophysics at the University of Copenhagen. Around 44% of human proteins have a disordered region made up of at least 30 amino acids. “It’s a relatively large fraction of them,” Lindorff-Larsen said.
AlphaFold2 can predict when a region is likely to be intrinsically disordered — but it can’t tell you what that disorder looks like.
For his part, Jumper’s biggest frustration is that AlphaFold2 doesn’t register the difference between two proteins that vary by a single amino acid, known as a point mutation. Point mutations can “have quite dramatic effects, sometimes on structure and often on function of proteins,” he said. “AlphaFold is relatively blind” to them, in that it will produce the same structure for both sequences.
In September 2023 DeepMind released AlphaMissense , a deep learning algorithm that predicts the effects of such mutations. It can’t show the change to the structure, but it informs the user if the mutation might turn the protein pathogenic or introduce dysfunction based on similar mutations in known pathogenic proteins.
However, even if AlphaFold2 could predict all proteins perfectly, it would be far from modeling biological reality. That’s because in a cell, proteins never act alone.
Cellular Complexity
The insides of cells are complicated and chaotic. A cell’s external membrane envelops a biochemical environment densely crowded with molecular parts — proteins, signaling molecules, messenger RNA, organelles and more. Proteins bind to each other and to other molecules, which alters their forms and functions.

Proteins don’t work alone: They interact constantly with other molecules. This rendering of a cellular landscape is made of models of real proteins and other molecules in “an attempt to visualize the great complexity and beauty of the cell’s molecular choreography,” wrote its creator, Gael McGill .
Evan Ingersoll and Gaël McGill, PhD/Digizyme Inc
AlphaFold2’s ability to predict the structure of a single protein doesn’t get biologists close to understanding proteins in this intricate native environment. But that’s the direction the field is now heading. Protein science’s artificial intelligence giants, Google DeepMind and David Baker’s Institute for Protein Design, are now evolving their deep learning algorithms to predict the structures that proteins assume while interacting with other molecules.
In spring 2024, they both published papers describing similar developments in this area. Updates to their algorithms — launched with the new names AlphaFold3 and RoseTTAFold All-Atom — enable them to predict the structures of proteins bound to each other, DNA, RNA and other small molecules.
Biologists are just starting to test out these updates. So far, AlphaFold3 is much more accurate than RoseTTAFold All-Atom, AlQuraishi said — but it’s not as big of a leap as an “AlphaFold2 moment.” For some macromolecules, such as RNA structures, its accuracy remains below that of other physics-based systems and experiments.
AlphaFold3 predicts the structures of molecular complexes, such as this enzyme found in a plant-damaging fungus. In this model structure, the protein (blue) is linked to simple sugars (yellow) and an ion (yellow sphere).
Even so, the new algorithms are a step in the right direction. The interactions between proteins and other molecules are critical to their functioning in cells. To develop drugs that can dock onto proteins and alter their activity as desired, researchers need to understand what those complexes look like. It’s unlikely, though, that either algorithm will lead to new medicines anytime soon, Adams said. “Both methods are still limited in their accuracy, [but] both are dramatic improvements on what was possible.”
There is one other major change in DeepMind’s new product. AlphaFold2’s underlying code was open-source so that other researchers could study the algorithm and remake it for their own projects. However, rather than share AlphaFold3’s source code, Google has so far opted to protect it as a trade secret. “For the time being, at least, no one can run and use it like they did with [AlphaFold2],” AlQuraishi said.
Even before the release of AlphaFold3, researchers had been testing AlphaFold2 to see if it could provide useful information on proteins in different conformations. Brenda Rubenstein , an associate professor of chemistry and physics at Brown University, was interested in kinases, a type of protein that activates other proteins. Specifically, she wanted to understand the mechanism of a kinase that causes cancer so that she could develop more precise drugs against it. Her lab modeled the kinase’s structure using a physics-based approach, which maps the 3D coordinates of atoms using Newton’s laws. It took two and a half years.
“About a year ago, we said: Can we do this faster?” Rubenstein said. They tried using AlphaFold2 in a novel way. By feeding data about related proteins to the algorithm, she found that it could predict her kinase in different conformations with more than 80% accuracy.
Rubenstein’s is one of several labs finding that “if you poke AlphaFold in the right way, you get it to kind of spit out alternate conformations,” AlQuraishi said. “That’s been encouraging.”
AlQuraishi hopes that by 2040, deep learning will be able to simulate an entire cell and all the structures and dynamics within it. Getting there, however, will require leaps on both the experimental and computational sides.
An Outsider’s Take
For many biologists, AlphaFold2 was the breakthrough they had been waiting for. The goal of CASP had been to create computing tools that predict protein structure from sequence. Still, many can’t help but ask: Why was a relative newcomer able to crack the protein code when so many experts had struggled for decades?
The insights that Google DeepMind’s team of computer and protein scientists brought to the problem are undeniable. At the same time, the ground of protein science was fertile and ready to yield a deep learning revolution, AlQuraishi said. “These things don’t appear out of nowhere.”
By the time CASP 2020 came around, many researchers expected a breakthrough in structure prediction to come through artificial intelligence. “It was all heading in that direction,” Townshend said. But they didn’t expect it to come from a multibillion-dollar technology company, and they didn’t expect it so soon. Some said AlphaFold2 wasn’t a feat of new science but rather clever engineering. Some were surprised that David Baker’s algorithms didn’t take the trophy. Others were less surprised because of Google DeepMind’s unmatched resources.
Around 100 labs participate in CASP every year, and though they had begun to adopt AI technologies, they “probably didn’t have the expertise that DeepMind had, nor the computing power,” Thornton said. DeepMind “had access to basically unlimited computing power.”
She also speculated that Google’s lack of expertise in protein science may have freed them creatively. “They were single-minded,” Thornton said, and focused on building a great neural network. Protein biologists had baggage. As they worked on their AI tools, they wanted to capture the atomic-level molecular physics and chemistry involved in protein folding. DeepMind had a different approach: We will transform sequence data into a 3D structure, and it doesn’t matter how we get there.
“Rather than trying to solve the protein folding problem, which I think a lot of previous predictions tried to do, they actually just went with the brute force” of mapping out the atoms’ final positions in space, Walden said. “Rather interestingly, they have therefore probably solved the problem.”

The Princeton University computer scientist Ellen Zhong, who was an intern on Google DeepMind’s AlphaFold2 team in 2021, pioneered the use of deep learning in combination with cryo-EM to study protein dynamics.
Tori Repp/Fotobuddy for Princeton University
To some biologists, that approach leaves the protein folding problem incomplete. From the earliest days of structural biology, researchers hoped to learn the rules of how an amino acid string folds into a protein. With AlphaFold2, most biologists agree that the structure prediction problem is solved. However, the protein folding problem is not. “Right now, you just have this black box that can somehow tell you the folded states, but not actually how you get there,” Zhong said.
“It’s not solved the way a scientist would solve it,” said Littman, the Brown University computer scientist.
This might sound like “semantic quibbling,” said George Rose, the biophysics professor emeritus at Johns Hopkins. “But of course it isn’t.” AlphaFold2 can recognize patterns in how a given amino acid sequence might fold up based on its analysis of hundreds of thousands of protein structures. But it can’t tell scientists anything about the protein folding process.
“For many people, you don’t need to know. They don’t care,” Rose said. “But science, at least for the past 500 years or so … has been involved with trying to understand the process by which things occur.” To understand the dynamics, mechanisms, functions and nature of protein-based life, Rose argued, you need the full story — one that deep learning algorithms can’t tell us.
To Moult, it doesn’t matter that the machine does something he doesn’t understand. “We’re all used to machines doing things we can’t. You know, I can’t run as fast as my car,” he said. To molecular biologists who are trying to study a protein and just need to know roughly what it looks like, how they get there doesn’t really matter.
But “until we know really how it works, we’re never going to have a 100% reliable predictor,” Porter said. “We have to understand the fundamental physics to be able to make the most informed predictions we can.”
“We keep moving the goalpost,” AlQuraishi said. “I do think that core problem has been solved, so now it’s very much about what comes next.”
Even as biologists continue to debate these topics, others are looking forward to a field that’s undeniably changed — and backward toward its recent past.
Sometimes Perrakis is hit by a wave of nostalgia for the old ways of doing things. In 2022, his team described an enzyme involved in modifying microtubules (giant, rod-shaped molecules that provide structures to cells) that they had determined using X-ray crystallography. “I realized that I’m never going to do that [again],” he said. “Having the first structure appearing after months of work was a very particular satisfaction.”
AlphaFold2 hasn’t made those experiments obsolete. On the contrary, it’s illuminated just how necessary they are. It has stitched together two historically disparate disciplines, launching a new and stimulating conversation.
The New World
Seventy years ago, proteins were thought to be a gelatinous substance, Porter said. “Now look at what we can see”: structure after structure of a vast world of proteins, whether they exist in nature or were designed.
The field of protein biology is “more exciting right now than it was before AlphaFold,” Perrakis said. The excitement comes from the promise of reviving structure-based drug discovery, the acceleration in creating hypotheses and the hope of understanding complex interactions happening within cells.
“It [feels] like the genomics revolution,” AlQuraishi said. There is so much data, and biologists, whether in their wet labs or in front of their computers, are just starting to figure out what to do with it all.
But like other artificial intelligence breakthroughs sparking across the world, this one might have a ceiling.
AlphaFold2’s success was founded on the availability of training data — hundreds of thousands of protein structures meticulously determined by the hands of patient experimentalists. While AlphaFold3 and related algorithms have shown some success in determining the structures of molecular compounds, their accuracy lags behind that of their single-protein predecessors. That’s in part because there is significantly less training data available.
The protein folding problem was “almost a perfect example for an AI solution,” Thornton said, because the algorithm could train on hundreds of thousands of protein structures collected in a uniform way. However, the Protein Data Bank may be an unusual example of organized data sharing in biology. Without high-quality data to train algorithms, they won’t make accurate predictions.
“We got lucky,” Jumper said. “We met the problem at the time it was ready to be solved.”
No one knows if deep learning’s success at addressing the protein folding problem will carry over to other fields of science, or even other areas of biology. But some, like AlQuraishi, are optimistic. “Protein folding is really just the tip of the iceberg,” he said. Chemists, for example, need to perform computationally expensive calculations. With deep learning, these calculations are already being computed up to a million times faster than before, AlQuraishi said.
Artificial intelligence can clearly advance specific kinds of scientific questions. But it may get scientists only so far in advancing knowledge. “Historically, science has been about understanding nature,” AlQuraishi said — the processes that underlie life and the universe. If science moves forward with deep learning tools that reveal solutions and no process, is it really science?
“If you can cure cancer, do you care about how it really works?” AlQuraishi said. “It is a question that we’re going to wrestle with for years to come.”
If many researchers decide to give up on understanding nature’s processes, then artificial intelligence will not just have changed science — it will have changed the scientists too.
Meanwhile, the CASP organizers are wrestling with a different question: how to continue their competition and conference. AlphaFold2 is a product of CASP, and it solved the main problem the conference was organized to address. “It was a big shock for us in terms of: Just what is CASP anymore?” Moult said.
In 2022, the CASP meeting was held in Antalya, Turkey. Google DeepMind didn’t enter, but the team’s presence was felt. “It was more or less just people using AlphaFold,” Jones said. In that sense, he said, Google won anyway.
Some researchers are now less keen on attending. “Once I saw that result, I switched my research,” Xu said. Others continue to hone their algorithms. Jones still dabbles in structure prediction, but it’s more of a hobby for him now. Others, like AlQuraishi and Baker, continue on by developing new algorithms for structure prediction and design, undaunted by the prospect of competing against a multibillion-dollar company.
Moult and the conference organizers are trying to evolve. The next round of CASP opened for entries in May. He is hoping that deep learning will conquer more areas of structural biology, like RNA or biomolecular complexes. “This method worked on this one problem,” Moult said. “There are lots of other related problems in structural biology.”
The next meeting will be held in December 2024 by the aqua waters of the Caribbean Sea. The winds are cordial, as the conversation will probably be. The stamping has long since died down — at least out loud. What this year’s competition will look like is anyone’s guess. But if the past few CASPs are any indication, Moult knows to expect only one thing: “surprises.”

Get highlights of the most important news delivered to your email inbox
Comment on this article
Quanta Magazine moderates comments to facilitate an informed, substantive, civil conversation. Abusive, profane, self-promotional, misleading, incoherent or off-topic comments will be rejected. Moderators are staffed during regular business hours (New York time) and can only accept comments written in English.

Next article

IMAGES
VIDEO
COMMENTS
RESEARCH TOPIC. Generative Adversarial Networks (GANs) are a class of unsupervised machine learning techniques to estimate a distribution from high-dimensional data and to sample elements that mimic the observations (Goodfellow et al., 2014). They use a zero-sum dynamic game be- tween two neural networks: a generator, which generates new ...
PhD Proposal in Artificial Intelligence and Machine Learning Artificial Intelligence for Ecosystem Monitoring using Remote Sensing and Digital Agriculture Data Advisors: ... Learning Research, pages 1481-1490, Lille, France, 07-09 Jul 2015. PMLR. [3]Julien Mairal. Large-Scale Machine Learning and Applications.
ANITI - ARTIFICIAL & NATURAL INTELLIGENCE TOULOUSE INSTITUTE https://aniti.univ-toulouse.fr/. PHD PROPOSAL IN ARTIFICIAL INTELLIGENCE AND MACHINE LEARNING. DESCRIPTION. In combinatorial optimization, the objective is to find an assignment of a set of decision variables that satisfy a set of constraints and that optimizes a given objective function.
Costs and Funding. Annual tuition fees for PhDs in Artificial Intelligence are typically around £4,000 to £5,000 for UK/EU students. Tuition fees for international students are usually much higher, typically around £25,000 per academic year. A variety of scholarships and funding support options are available for postgraduate study.
CSCI/PHIL 6550 Artificial Intelligence (3 hours) ARTI 6950 Faculty Research Seminar (1 hour) ARTI/PHIL 6340 Ethics and Artificial Intelligence (3 hours) Elective Courses. In addition to the required courses above, at least 6 additional courses must be taken from Groups A and Group B below, subject to the following requirements.
Thesis Proposal; University Oral Examination; Dissertation; Progress Guidelines. ... Faculty by Research. Architecture; Artificial Intelligence; Biomedicine and Health; Computational Biology; ... The Computer Science Department PhD program is a top-ranked research-oriented program, typically completed in 5-6 years. ...
Search Funded PhD Research Projects in Computer Science, Artificial Intelligence. Search for PhD funding, scholarships & studentships in the UK, Europe and around the world. PhDs ; ... research proposal tips and much more; Early access to our physical and virtual postgraduate study fairs; Complete your profile.
A Ph.D. in AI is the highest awarded degree in the field of artificial intelligence. It involves several years of study and research, culminating in a project, thesis, or dissertation of original academic research that expands our knowledge of AI. The main focus of a Ph.D. in AI is research. Students spend about six years researching one topic.
This PhD project addresses the challenge of early-stage cancer detection. Artificial intelligence (AI) and machine learning (ML) are transforming medical diagnosis, such as cancer detection. Read more. Supervisors: Dr F Langbein, Dr J Wu, Dr K Sidorov, Dr A Abdelmoty.
Need to be related with your degree and present research areas of artificial intelligence. In general, the PhD research proposal has a standard format to write. As well, it is composed of different components such as title, abstract, introduction, literature study, methodologies, conclusion, and references. In fact, we have a native writer team ...
The PhD in Artificial Intelligence is centered upon how computers operate to match the human decision making process in the brain. Your research will be led by AI experts with both research and industrial expertise. This emerging subject is starting to attract attention on the wider issues as the IOT and other advanced computer systems work in ...
PhD proposal: An Artificial Intelligence framework to improve the learning of dynamic models from time series data Supervisors : Pr. Morgan MAGNIN and Pr. Olivier ROUX (École centrale de Nantes, LS2N) ... The research domain of this PhD thesis is the formal modelling and analysis of complex dynamical systems (specifically in biological systems ...
Best Masters and PhD Research Proposal in Artificial Intelligence (AI) Nowadays, people live their life with more advancements and ease of access to the developed technologies. Artificial intelligence is the process of mimicking behaviors, or functions of the human brain by the computes or machines in problem-solving and learning information.
PHD PROPOSAL IN ARTIFICIAL INTELLIGENCE AND MACHINE LEARNING. Two PhD positions are available in the framework of the Horizon EU Project TUPLES : Trustworthy Planning and Scheduling with Learning and Explanations, aiming to develop scalable, yet transparent, robust and safe algorithmic solutions for planning and scheduling. Expand.
A research proposal is a systematic and concise synopsis of proposed research. Encompassing the key information, it serves as a roadmap to the principal components of the study. The main aim of a research proposal is to establish the necessity of the research, elucidate its aims and objectives, and illustrate the originality or significance of ...
The PhD Studentship supports and encourages under-represented groups, namely female and Black researchers, to pursue postgraduate research in AI or Machine Learning. The PhD DeepMind Studentship will cover tuition fees and offer a London weighted stipend of £19,668 per year minimum together with an annual £2,200 travel and conference ...
Doctor of Philosophy in Artificial Intelligence and Data Science. The Doctor of Philosophy (Ph.D.) degree is a research-oriented degree requiring a minimum of 64 semester credit hours of approved courses and research beyond the Master of Science (M.S.) degree [96 credit hours beyond the Bachelor of Science (B.S.) degree].
PhD Project Proposals ... recent progress in advanced artificial intelligence algorithms such as deep learning has created ... More specifically, the PhD project will research current methods and limitations of developing . empirical models of value-chain systems using sensor data. It will then design methods for developing
Artificial Intelligence. The U.S. National Science Foundation has invested in foundational artificial intelligence research since the early 1960s, setting the stage for today's understanding and use of AI technologies. AI-driven discoveries and technologies are transforming Americans' daily lives — promising practical solutions to global ...
Use of Artificial Intelligence (A.I) and Machine Learning (ML) used for creating Navigational Situational awareness in Maritime Autonomous Surface Ships (MASS) Please contact. Dr Philippa Ryan. Applications accepted all year, but ideally looking for September 2024 start date. Funded PhD Project (UK Students Only).
Artificial Intelligence Research Topics. Topic 1: Machine Learning and Artificial Intelligence in the Next Generation Wearable Devices. Topic 2: Automation, machine learning and artificial intelligence in the field of medicine. Topic 3: Robotics and artificial intelligence - Assessing the Impact on business and economics.
Lastly, the research paper (Muthmainnah et al., 2022), looked at how artificial intelligence (AI) can help people learning English as a second language strengthen their critical thinking skills.
T he University has announced 50 fully-funded PhD researcher places to unlock the potential of Artificial Intelligence (AI) in medical diagnosis and care.. A new centre for doctoral training (CDT) will be created, with a focus on the early detection, diagnosis, treatment and care of cancers. It follows newly created government funding from UK Research and Innovation, to aid in training the ...
Artificial-intelligence algorithms could identify scientists who need support with translating their work into real-world applications and more. Leaders must step up.
The use of Artificial Intelligence (AI) in education is transforming various dimensions of the education system, such as instructional practices, assessment strategies, and administrative processes. It also plays an active role in the progression of science education. This systematic review attempts to render an inherent understanding of the evidence-based interaction between AI and science ...
This paper presents a strategy of state-of-the-art review of artificial intelligence (AI) in education and a case study about preparing students to have the competencies and skills necessary for ...
Evan Calabrese, MD, PhD, an assistant professor of radiology at Duke University Medical Center and a physician-scientist researcher at the Duke Center for Artificial Intelligence in Radiology (DAIR), provides a detailed analysis of his research on artificial intelligence (AI) applications in pediatric neuroradiology, focusing on neonatal hypoxic-ischemic encephalopathy (HIE).
Artificial intelligence (AI) is a highly interdisciplinary field, characterized by a range of different approaches, theories, and methods. Deception is as central to AI's functioning as the circuits, software, and data that make it run. This book argues that, since the beginning of the computer age, researchers and developers have explored the ways users are led to believe that computers are ...
Artificial intelligence can clearly advance specific kinds of scientific questions. But it may get scientists only so far in advancing knowledge. "Historically, science has been about understanding nature," AlQuraishi said — the processes that underlie life and the universe.
Mathematical Foundations of Artificial Intelligence Webinar June 12, 2024. Agenda • MFAI Introduction and Motivation ... PI, co-PI, senior/key personnel, postdoctoral scholars, graduate or undergraduate students, or other trainees from the full spectrum of diverse talent in STEM, including ... and major research institutions. • Proposals ...