Token Economy: A Systematic Review of Procedural Descriptions
Affiliations.
- 1 1 The Pennsylvania State University - Harrisburg, Middletown, USA.
- 2 2 The University of Memphis, TN, USA.
- 3 3 The Achievement Center, Erie, PA, USA.
- PMID: 28423911
- DOI: 10.1177/0145445517699559
The token economy is a well-established and widely used behavioral intervention. A token economy is comprised of six procedural components: the target response(s), a token that functions as a conditioned reinforcer, backup reinforcers, and three interconnected schedules of reinforcement. Despite decades of applied research, the extent to which the procedures of a token economy are described in complete and replicable detail has not been evaluated. Given the inherent complexity of a token economy, an analysis of the procedural descriptions may benefit future token economy research and practice. Articles published between 2000 and 2015 that included implementation of a token economy within an applied setting were identified and reviewed with a focus on evaluating the thoroughness of procedural descriptions. The results show that token economy components are regularly omitted or described in vague terms. Of the articles included in this analysis, only 19% (18 of 96 articles reviewed) included replicable and complete descriptions of all primary components. Missing or vague component descriptions could negatively affect future research or applied practice. Recommendations are provided to improve component descriptions.
Keywords: replication; systematic literature review; technological; token economy; token economy procedures.

Publication types
- Systematic Review
- Behavior Therapy / methods*
- Token Economy*
Token Economies
- First Online: 06 January 2023
Cite this chapter
- Tonya N. Davis 3 &
- Jessica S. Akers 3
371 Accesses
Token economies are made up of three interlocking contingencies including the token-production schedule, exchange-production schedule, and token-exchange schedule. Tokens must be paired with several unconditioned and conditioned reinforcers in order to serve as an effective generalized conditioned reinforcer. In this chapter, you will introduce your supervisees to generalized conditioned reinforcement and token economies. During the group supervision meeting, you will review the steps for developing a token economy and the importance of the interlocking schedules of reinforcement. In addition, your supervisees will practice developing a token economy and identifying the different interlocking contingencies within token economies. During the individual supervision meeting, you and your supervisee will review the plan that they developed for implementing a token economy with their client. The important procedures will be the identifying the behavior of interest and the backup reinforcers. During the final supervision meeting, you will instruct your supervisee to implement the token economy with their client.
This is a preview of subscription content, log in via an institution to check access.
Access this chapter
- Available as PDF
- Read on any device
- Instant download
- Own it forever
- Available as EPUB and PDF
- Compact, lightweight edition
- Dispatched in 3 to 5 business days
- Free shipping worldwide - see info
- Durable hardcover edition
Tax calculation will be finalised at checkout
Purchases are for personal use only
Institutional subscriptions
Hackenberg, T. D. (2009). Token reinforcement: A review and analysis. Journal of the Experimental Analysis of Behavior, 91 (2), 257–286. https://doi.org/10.1901/jeab.2009.91-257
Article PubMed PubMed Central Google Scholar
Hine, J. F., Ardoin, S. P., & Call, N. A. (2018). Token economies: Using basic experimental research to guide practical applications. Journal of Contemporary Psychotherapy, 48 , 145–154. https://doi.org/10.1007/s10879-017-9376-5
Article Google Scholar
Moher, C. A., Gould, D. D., Hegg, E., & Mahoney, A. M. (2008). Non-generalized and generalized conditioned reinforcers: Establishment and validation. Behavioral Interventions, 23 , 13–38. https://doi.org/10.1002/bin.253
Sleiman, A. A., Betz, A. M., Rey, C. N., & Blackman, A. L. (2020). Effects of token manipulation on responding within a token economy implemented with children with autism. Education and Treatment of Children, 43 , 323–333. https://doi.org/10.1007/s43494-020-00014-2
Download references
Author information
Authors and affiliations.
Baylor University, Waco, TX, USA
Tonya N. Davis & Jessica S. Akers
You can also search for this author in PubMed Google Scholar
Electronic Supplementary Material
(pptx 62 kb), appendix a: interlocking contingencies worksheet.
Kari receives a token after writing five spelling words. Once she earns 10 tokens, she can take a break with requested preferred items.
Token-Production Schedule
Exchange-Production Schedule
Token-Exchange Schedule
Chiney earns a token for every free throw she makes. Once she earns 20 tokens, she can exchange her tokens for a reinforcer. High-preference activities such as listening to music require 30 tokens and moderate-preference activities such as taking selfies require 10 tokens.
Damian earns one token after reading continuously for 1 minute. He can exchange tokens during recess and lunch break. Damian can purchase 10 extra minutes of recess or lunch break for 30 tokens.
Corey earns one token after hitting an average of 10 baseballs. He can exchange his tokens once he earns 50 tokens. His high-preference reinforcers require 60 tokens and his moderate-preference items require 20 tokens.
Shannon earns one token for each 15-minute period in which she remains on-task at work. She can exchange her tokens at the beginning and end of the workday. She can exchange 5 tokens for a piece of candy, 15 tokens for a soda, 50 tokens for lunch from a selected fast-food restaurant, and 300 tokens for permission to arrive at work at 10:00 am instead of 8:00 am.
Interlocking Contingencies Worksheet Answer Guide
High-preference activities 30 tokens; moderate-preference activities 10 tokens
1 min whole interval
Recess and lunch break
High-preference reinforcers 60 tokens; moderate-preference reinforcers 20 tokens
Whole interval 15 minutes
Beginning and end of the workday
5 tokens for candy; 15 tokens for soda; 50 tokens for lunch; 300 tokens for late arrival to work
Appendix B: Plan for Token Economy Development
Define the target behavior
□ Identify the conditions under which the behavior should occur (e.g., when instructed to clean up)
□ Topographical definition (e.g., picks up toy from the ground and places the toy in the designated container)
□ Required measurement dimensions that must be met (e.g., within 3 of the instruction, or cleans up for 1 minute continuously)
Select tokens and procedures for establishing them as conditioned reinforcers
□ Identify the stimulus that will serve as the token
□ Determine how the tokens will be conditioned as reinforcers
Develop a menu of back up reinforcers
□ Identify items that have in the past resulted in increased responding
□ Try to include an adequate number of back up reinforcers to reduce the impact of motivational variables. This number will vary based on the client’s history of reinforcement and the variety of reinforcers available.
Determine contingencies for earning and losing tokens
□ Identify the schedule of reinforcement for the behavior of interest. This could be the number of responses or the duration of engagement in the response.
Determine the prices of back up reinforcers
□ Identify how many tokens are required to purchase different reinforcers
□ High-preference items should “cost” more than moderate-preference items
Determine when and how tokens can be exchanged
□ Identify how many tokens must be earned before they can be exchanged
Develop a system for progress monitoring and a plan for fading
Appendix C: Graph Component Checklist
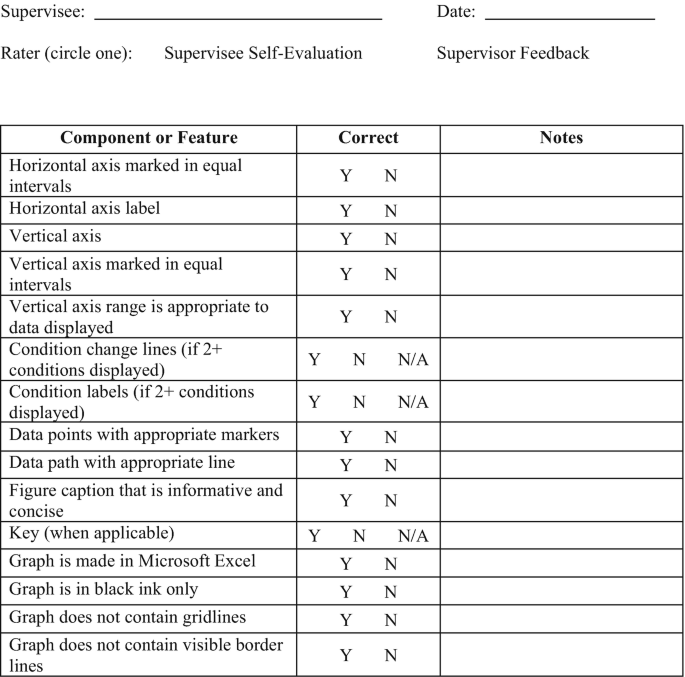
Appendix D: Token Economy Procedural Fidelity Checklist
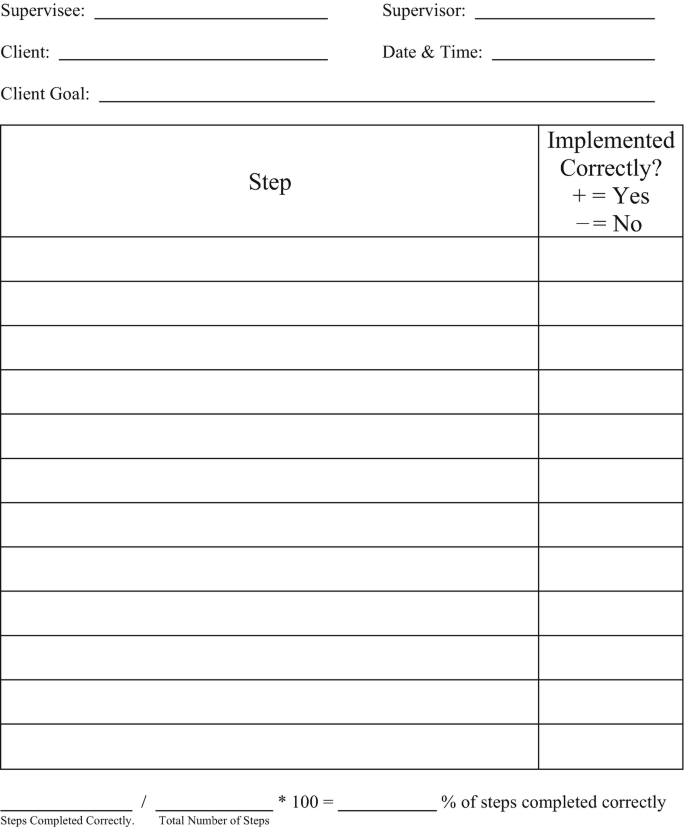
Rights and permissions
Reprints and permissions
Copyright information
© 2022 The Author(s), under exclusive license to Springer Nature Switzerland AG
About this chapter
Davis, T.N., Akers, J.S. (2022). Token Economies. In: A Behavior Analyst’s Guide to Supervising Fieldwork. Springer, Cham. https://doi.org/10.1007/978-3-031-09932-8_27
Download citation
DOI : https://doi.org/10.1007/978-3-031-09932-8_27
Published : 06 January 2023
Publisher Name : Springer, Cham
Print ISBN : 978-3-031-09931-1
Online ISBN : 978-3-031-09932-8
eBook Packages : Behavioral Science and Psychology Behavioral Science and Psychology (R0)
Share this chapter
Anyone you share the following link with will be able to read this content:
Sorry, a shareable link is not currently available for this article.
Provided by the Springer Nature SharedIt content-sharing initiative
- Publish with us
Policies and ethics
- Find a journal
- Track your research
- Help & FAQ
Systematic Review and Meta-Analysis of Token Economy Practices in K-5 Educational Settings, 2000 to 2019
- School of Behavioral Sciences & Education (Harrisburg)
Research output : Contribution to journal › Review article › peer-review
Token economy systems have been widely used as an evidence-based classroom management strategy to reinforce and improve prosocial responses. While token economies have been widely applied to educational settings, there have been mixed results regarding the effectiveness depending on the classroom type. To better understand the components contributing to the effectiveness, the researchers analyzed 24 token economy studies conducted in general and special education classrooms from kindergarten to fifth grade between 2000 and 2019. Eight token economy components and effect sizes were identified for each study and compared across different classroom types. The results showed that the token economy intervention yielded large effect sizes for both general and special education classroom types. There were differences in the usage of token components including backup reinforcer types, token production rate, and exchange production rate based on classroom types. Implications for future research and practice for educators and clinicians are discussed.
All Science Journal Classification (ASJC) codes
- Developmental and Educational Psychology
- Clinical Psychology
- Arts and Humanities (miscellaneous)
Access to Document
- 10.1177/01454455211058077
Other files and links
- Link to publication in Scopus
- Link to the citations in Scopus
Fingerprint
- Token Economy Medicine & Life Sciences 100%
- Meta-analysis Arts & Humanities 79%
- educational setting Social Sciences 59%
- Meta-Analysis Medicine & Life Sciences 44%
- Systematic Reviews Medicine & Life Sciences 43%
- economy Social Sciences 36%
- Special Education Arts & Humanities 36%
- Effect Size Arts & Humanities 35%
T1 - Systematic Review and Meta-Analysis of Token Economy Practices in K-5 Educational Settings, 2000 to 2019
AU - Kim, Ji Young
AU - Fienup, Daniel M.
AU - Oh, Alice E.
AU - Wang, Ye
N1 - Publisher Copyright: © The Author(s) 2021.
PY - 2022/11
Y1 - 2022/11
N2 - Token economy systems have been widely used as an evidence-based classroom management strategy to reinforce and improve prosocial responses. While token economies have been widely applied to educational settings, there have been mixed results regarding the effectiveness depending on the classroom type. To better understand the components contributing to the effectiveness, the researchers analyzed 24 token economy studies conducted in general and special education classrooms from kindergarten to fifth grade between 2000 and 2019. Eight token economy components and effect sizes were identified for each study and compared across different classroom types. The results showed that the token economy intervention yielded large effect sizes for both general and special education classroom types. There were differences in the usage of token components including backup reinforcer types, token production rate, and exchange production rate based on classroom types. Implications for future research and practice for educators and clinicians are discussed.
AB - Token economy systems have been widely used as an evidence-based classroom management strategy to reinforce and improve prosocial responses. While token economies have been widely applied to educational settings, there have been mixed results regarding the effectiveness depending on the classroom type. To better understand the components contributing to the effectiveness, the researchers analyzed 24 token economy studies conducted in general and special education classrooms from kindergarten to fifth grade between 2000 and 2019. Eight token economy components and effect sizes were identified for each study and compared across different classroom types. The results showed that the token economy intervention yielded large effect sizes for both general and special education classroom types. There were differences in the usage of token components including backup reinforcer types, token production rate, and exchange production rate based on classroom types. Implications for future research and practice for educators and clinicians are discussed.
UR - http://www.scopus.com/inward/record.url?scp=85119252866&partnerID=8YFLogxK
UR - http://www.scopus.com/inward/citedby.url?scp=85119252866&partnerID=8YFLogxK
U2 - 10.1177/01454455211058077
DO - 10.1177/01454455211058077
M3 - Review article
C2 - 34784784
AN - SCOPUS:85119252866
SN - 0145-4455
JO - Behavior Modification
JF - Behavior Modification
- Skip to main content
- Skip to primary sidebar
IResearchNet
Token Economy
Token Economy, a fundamental concept in the realm of behavioral psychology, has been instrumental in shaping the landscape of school psychology. This article provides an in-depth exploration of the theoretical foundations of token economy, tracing its historical development and elucidating the core principles of tokens, backup reinforcers, and contingencies. It subsequently delves into the practical implementation and utilization of token economy within educational settings, highlighting the steps, benefits, and challenges inherent to its application in schools. Furthermore, the article scrutinizes the critiques and ethical considerations associated with token economies and contemplates future directions for this concept. By critically examining the past and present, this article underscores the enduring significance of token economy in the field of psychology and its indispensable role in shaping educational environments.
Introduction
Token Economy is a concept of paramount importance within the realm of behavioral psychology, holding a profound significance for the field and its practical applications. At its core, a token economy is a systematic and structured reinforcement system used to promote desirable behaviors and discourage problematic ones. The utilization of tokens, in the form of tangible or symbolic rewards, serves as a means to reinforce targeted behaviors. These tokens can be accumulated by individuals over time and subsequently exchanged for desirable rewards or privileges, commonly referred to as backup reinforcers. This process hinges on the principles of operant conditioning, where rewards (tokens) function as positive reinforcement, strengthening the association between behavior and reward, thereby increasing the likelihood of the desired behavior recurring.
The historical roots of the token economy can be traced back to the mid-20th century, with its theoretical foundations firmly grounded in the pioneering work of behavioral psychologists, particularly B.F. Skinner. Skinner’s contributions to the field of behaviorism and operant conditioning provided the theoretical framework upon which token economy systems were built. Over time, the concept has evolved and been refined, finding extensive applications in various domains, with a particularly profound impact in the field of school psychology. This article will delve into the theoretical underpinnings of token economy systems, their practical implementation within educational settings, and the implications and critiques associated with their use. It will also explore the ethical considerations and potential future directions for this influential concept, underscoring its enduring significance in the broader discipline of psychology.
Theoretical Foundations of Token Economy
Token economy systems are firmly rooted in the principles of behavioral psychology, with a primary focus on operant conditioning and reinforcement. Understanding these theoretical foundations is crucial for grasping the significance and functionality of token economies.
At the heart of token economies lies the concept of operant conditioning, a behavioral theory developed and popularized by B.F. Skinner and other behaviorists. Operant conditioning posits that behaviors are shaped by their consequences. When a behavior results in a favorable outcome or reward, it becomes more likely to be repeated. Conversely, when a behavior leads to unfavorable consequences, it is less likely to recur. Token economies leverage this principle by introducing a system of immediate reinforcement, utilizing tokens as a bridge between the behavior and the final reinforcement.
The historical development of token economy systems can be traced back to B.F. Skinner’s pioneering work in the mid-20th century. Skinner’s experiments with operant conditioning and the Skinner box laid the foundation for understanding how behaviors can be shaped and maintained through reinforcement. His work emphasized the use of positive reinforcement to increase the likelihood of specific behaviors, which is a fundamental principle of token economies. Over time, other behaviorists such as Ogden Lindsley and Montrose Wolf contributed to the refinement of these concepts and the practical application of token economies.
Token economies encompass several key concepts that underpin their effectiveness. Tokens are symbolic or tangible items (e.g., stickers, points, chips) that serve as immediate reinforcement for desirable behaviors. These tokens are secondary reinforcers because they acquire their value through their association with primary reinforcers (i.e., rewards or privileges). Backup reinforcers are the ultimate rewards that individuals can acquire through the exchange of tokens. These backup reinforcers can vary from simple privileges (e.g., extra playtime) to more substantial rewards (e.g., a special outing or a preferred item). Contingencies in token economies refer to the specific conditions or criteria that must be met to earn tokens and subsequently exchange them for backup reinforcers. These contingencies are clearly defined and established to ensure consistency and effectiveness.
Token economies find diverse and widespread applications in real-world settings, with schools being a prominent example. In educational contexts, teachers often implement token economy systems to reinforce positive behaviors in students. For instance, a teacher may award students with tokens for completing assignments, exhibiting good behavior, or participating in class activities. These tokens can then be accumulated and exchanged for privileges like extra recess time or the opportunity to choose a classroom activity. The clear contingencies and the immediate nature of token reinforcement make them particularly effective in shaping behaviors, especially in children.
Beyond schools, token economies are employed in various settings, including correctional facilities, mental health institutions, and addiction treatment centers. In prisons, for instance, inmates can earn tokens for compliance with rules, participation in rehabilitation programs, or maintaining a clean living space. These tokens may be used to secure additional visitation hours or other incentives. In mental health settings, individuals may receive tokens for engaging in therapeutic activities or demonstrating socially appropriate behaviors.
In essence, the theoretical foundations of token economy systems, grounded in operant conditioning and reinforcement principles, have been refined and extended over time to offer practical solutions for behavior modification in a variety of real-world scenarios. These systems have proven to be effective tools for promoting positive behaviors and achieving specific behavioral goals.
Implementation and Use of Token Economy in School Psychology
Token economy systems have garnered widespread recognition and application in educational settings, with a particular focus on school psychology. These systems are valuable tools in shaping students’ behavior and fostering a positive learning environment. In this section, we will delve into the implementation of token economies in schools, elucidating the steps involved, the benefits and challenges associated with their use, and the compelling research findings regarding their efficacy.
Token economies are extensively used in educational environments, aiming to reinforce desirable behaviors and discourage undesirable ones. School psychologists, teachers, and other educational professionals have employed token economy systems to promote academic engagement, social interactions, and compliance with school rules. By providing a structured and immediate reinforcement system, students are encouraged to meet specific behavioral goals, resulting in a more conducive learning environment.
The implementation of a token economy system in schools involves a series of structured steps:
- Identify Target Behaviors: The first step is to identify the specific behaviors that the school wishes to promote or reduce. These behaviors should be observable, measurable, and realistic.
- Select Appropriate Tokens: Once the target behaviors are identified, the tokens or rewards to be used in the system need to be selected. These tokens should be desirable to the students and easy to distribute.
- Define Contingencies: Clear and well-defined contingencies are crucial. Students should know what behaviors will earn them tokens, how many tokens they need to earn for a specific reward, and when and where they can exchange tokens for rewards.
- Establish a Tracking System: Schools often use charts or point sheets to track students’ progress in earning tokens. This allows for transparency and accountability.
- Implement Consistency: It is essential for all involved school staff to implement the system consistently. This consistency ensures that students understand the rules and expectations and receive reinforcement as appropriate.
- Monitor and Adjust: Regularly monitor the effectiveness of the token economy system. Adjust the system as needed, considering the changing needs and behaviors of the students.
Token economy systems in schools offer a range of benefits:
- Positive Behavior Reinforcement: Token economies provide an immediate and positive reinforcement mechanism, making them effective in promoting desired behaviors such as completing assignments, following rules, and participating in class.
- Individualization: These systems can be tailored to individual students, allowing for personalized reinforcement strategies. This is particularly valuable for students with special needs.
- Teaching Responsibility: Students learn to manage their tokens, budget for rewards, and make choices, fostering responsibility and decision-making skills.
- Reduction of Undesirable Behaviors: Token economies have been successful in reducing problematic behaviors like disruptions, aggression, and noncompliance.
However, challenges also exist:
- Implementation Consistency: Maintaining consistent implementation across all school staff can be challenging. Variability in reinforcement can undermine the effectiveness of the system.
- Overreliance on Tokens: There is a concern that students may become overly reliant on external rewards, potentially reducing their intrinsic motivation.
- Ethical Considerations: Critics argue that token economies can raise ethical concerns related to control and autonomy, as students may feel manipulated to conform to expectations.
Numerous studies support the effectiveness of token economies in school settings. Research findings consistently demonstrate that token economies are associated with increased academic engagement, improved classroom behavior, and reduced disruptive conduct. For instance, a study conducted by Kazdin and Bootzin (1972) revealed that token economy systems were effective in reducing disruptive behavior in elementary school students. Additionally, a meta-analysis by Van Lier and Koot (2010) found that token economy interventions were associated with reductions in externalizing behaviors among children.
In conclusion, the implementation of token economy systems in schools, guided by the principles of behaviorism and reinforcement, has proven to be a valuable strategy for promoting positive behaviors and reducing problematic behaviors in students. Although challenges exist, the extensive research supporting the efficacy of these systems underscores their importance in school psychology and the potential for continued refinement and application in educational contexts.
Critiques, Ethical Considerations, and Future Directions
While token economy systems have demonstrated their efficacy in promoting positive behavior, they are not without criticisms and ethical concerns. This section explores the critiques and limitations of token economies in psychology and education, discusses ethical considerations, and delves into current trends and future directions in the field of token economies, including technological advancements and ethical enhancements.
Critiques and Limitations:
- Overreliance on External Rewards: One common critique is that token economies may lead to an overreliance on external rewards, potentially diminishing students’ intrinsic motivation. Students might focus solely on earning tokens, rather than developing a genuine interest in learning or behaving well.
- Generalization Challenges: Token economy systems often work well within the controlled environment of the classroom but may struggle to generalize to real-world situations. Students may exhibit the desired behaviors only in the context where tokens are earned.
- Resistance to Fading: As students become accustomed to receiving tokens, they may become resistant to the gradual fading of token reinforcement. This can complicate the long-term sustainability of the system.
Ethical Considerations:
- Control and Autonomy: Critics argue that token economies may exert a level of control over students that limits their autonomy. Students might feel coerced into conformity, potentially undermining their sense of self-determination.
- Stigmatization: Some students may be stigmatized or embarrassed by the public nature of token reinforcement systems. This can negatively impact their self-esteem and peer relationships.
- Potential Negative Consequences: If not well-implemented, token economies can lead to negative consequences, such as excessive competition among students, cheating or gaming the system, or resentment among those who do not receive tokens.
Current Trends and Future Directions:
- Technology-Based Token Systems: One of the current trends in token economies is the integration of technology. Digital platforms and apps allow for more efficient tracking and management of token systems. They also provide opportunities for gamification, making the process more engaging and interactive for students.
- Enhanced Ethical Considerations: Researchers and educators are exploring ways to address ethical concerns. This includes modifying token systems to incorporate elements of choice, providing students with more autonomy in selecting rewards, and ensuring the privacy and dignity of participants.
- Individualized Programs: Future directions in token economies may involve the development of highly individualized programs. Recognizing that one-size-fits-all solutions may not be ideal, educators are exploring ways to tailor token systems to the unique needs and preferences of each student.
- Combination with Other Interventions: Token economies can be integrated with other behavior intervention strategies, creating a more comprehensive approach to behavior modification. For example, combining token systems with social skills training or counseling can address underlying issues while reinforcing positive behavior.
- Research on Long-Term Effects: There is a growing interest in understanding the long-term effects of token economies on students. Research is needed to investigate whether the positive behaviors nurtured by token systems persist beyond the immediate reinforcement period and into adulthood.
In conclusion, while token economies have been a valuable tool in psychology and education, it is essential to consider the critiques and ethical concerns associated with their use. The field is evolving with current trends embracing technology and focusing on enhancing ethical considerations. The future of token economies may involve more individualization and a greater emphasis on long-term impact, positioning them as dynamic tools for behavior modification in evolving educational environments.
Token Economy, a foundational concept in behavioral psychology, has played a pivotal role in shaping the landscape of school psychology and behavior modification. This article has provided a comprehensive exploration of the theoretical underpinnings, practical implementation, ethical considerations, and future directions of token economy systems.
In summary, token economies leverage the principles of operant conditioning and reinforcement to promote positive behaviors in educational settings. Their historical development, rooted in the work of behaviorists like B.F. Skinner, has led to their widespread application in schools. Through the judicious use of tokens, backup reinforcers, and clearly defined contingencies, token economies have effectively improved student behavior, engagement, and learning outcomes.
However, it is essential to acknowledge the critiques and ethical concerns associated with token economies, including issues related to control, autonomy, and potential negative consequences. The field is evolving, with technology-based systems and enhanced ethical considerations at the forefront.
Token economy systems continue to hold immense potential for further research and development. Their adaptability, individualization, and potential long-term impact underscore their enduring significance in the field of psychology. As educational environments evolve, token economies remain valuable tools for fostering positive behaviors, offering a bridge between psychological theory and real-world application.
References:
- Kazdin, A. E., & Bootzin, R. R. (1972). The token economy: A decade later. Journal of Applied Behavior Analysis, 5(4), 343-353.
- Van Lier, P. A., & Koot, H. M. (2010). Developmental cascades of peer relations and symptoms of externalizing behavior. Development and Psychopathology, 22(1), 217-232.
- Skinner, B. F. (1938). The behavior of organisms: An experimental analysis. D. Appleton-Century Company.
- Lindsley, O. R. (1992). From Skinner to precision teaching: The child knows best. In S. Axelrod & J. Apsche (Eds.), The effects of punishment on human behavior (pp. 19-53). Academic Press.
- Wolf, M. M. (1978). Social validity: The case for subjective measurement or how applied behavior analysis is finding its heart. Journal of Applied Behavior Analysis, 11(2), 203-214.
- Cooper, J. O., Heron, T. E., & Heward, W. L. (2020). Applied behavior analysis (3rd ed.). Pearson.
- Kazdin, A. E. (1982). The token economy: A review and evaluation. Plenum Press.
- Alberto, P. A., & Troutman, A. C. (2019). Applied behavior analysis for teachers. Pearson.
- Sigurdardottir, V. L., & Green, G. (1991). Behavioral training for problem behavior in children with mental retardation. Behavioral Interventions, 6(3), 181-198.
- Riordan, B. C., & Graffam, J. (2007). The utility of token economies in the management of disruptive behavior in intellectual disabilities: A review. Research in Developmental Disabilities, 28(5), 475-492.
- Vollmer, T. R., & Iwata, B. A. (1992). The concept of automatic reinforcement: Implications for behavioral assessment of individuals with developmental disabilities. Behavior Analysis in Practice, 1(1), 4-14.
- Slaton, J. D., Hanley, G. P., & Raftery, K. J. (2003). Teacher-versus peer-mediated discrete trial training for children with autism. Journal of Applied Behavior Analysis, 36(2), 161-165.
- Smith, R. G., & Iwata, B. A. (1997). Antecedent influences on problem behavior. Journal of Applied Behavior Analysis, 30(2), 343-375.
- Ahearn, W. H., Clark, K. M., MacDonald, R. P., & Chung, B. I. (2007). Assessing and treating vocal stereotypy in children with autism. Journal of Applied Behavior Analysis, 40(2), 263-275.
- Neef, N. A., Iwata, B. A., Page, T. J., & Neef, A. (1977). Teaching university students to give and follow instructions. Journal of Applied Behavior Analysis, 10(3), 337-347.
- Carr, E. G., & Durand, V. M. (1985). Reducing behavior problems through functional communication training. Journal of Applied Behavior Analysis, 18(2), 111-126.
- Fisher, W. W., Piazza, C. C., Bowman, L. G., Hagopian, L. P., Owens, J. C., & Slevin, I. (1992). A comparison of two approaches for identifying reinforcers for persons with severe and profound disabilities. Journal of Applied Behavior Analysis, 25(2), 491-498.
- Mace, F. C., & Lalli, J. S. (1991). Linking descriptive and experimental analyses in the treatment of bizarre speech. Journal of Applied Behavior Analysis, 24(3), 553-562.
- Piazza, C. C., Adelinis, J. D., Hanley, G. P., Goh, H. L., & Delia, M. D. (2000). An evaluation of the effects of matched stimuli on behaviors maintained by automatic reinforcement. Journal of Applied Behavior Analysis, 33(1), 13-27.
- Hanley, G. P., Iwata, B. A., & McCord, B. E. (2003). Functional analysis of problem behavior: A review. Journal of Applied Behavior Analysis, 36(2), 147-185.
- Frontiers in Blockchain
- Blockchain in Industry
- Research Topics
Blockchain and Tokenomics for Sustainable Development
Total Downloads
Total Views and Downloads
About this Research Topic
The purpose of innovation is to deliver a better world to future generations. Over the last decade, the emergence of blockchain technology as a key enabler in unlocking a new phase of the Internet evolution has attracted significant interest from both the academic and the industrial world. As the blockchain ecosystem matures, it is important to clarify how the new breed of global infrastructures created may be turned into value for society. The Sustainable Development Goals (SDGs), endorsed by the UN in 2015, offer a comprehensive framework for addressing a wide range of pressing global issues including poverty, inequality, climate change, and sustainable economic growth. The purpose of this request for scientific contributions is to investigate and evaluate how blockchain technology may be leveraged to accelerate the achievement of SDGs. The overarching aim of this special issue is to shed light on how blockchain may contribute to the attainment of a triple-sustainability paradigm within the global economy. It does so by encouraging the exploration of new business and governance models, as well as incentive systems aimed at better allocation, redistribution, and preservation of both financial and natural resources. Additionally, given the global nature of the most pressing policy challenges, there is a need to increase the level of international collaboration and coordination. In this respect, blockchain - through transparency and immutability - can play a key role in building a layer of trust, which represents a prerequisite for any collaboration effort at both the institutional and individual levels. This Research Topic is therefore specifically interested in developing and strengthening original research on the emerging concept of blockchain towards the development of sustainable solutions that are also connected with the current SDGs. It seeks interdisciplinary contributions exploring the potential of blockchain in addressing various environmental, social, and economic challenges outlined in the SDGs and foster discussions on the sustainable applications and implications of blockchain technology in achieving these global objectives. We invite researchers, academics, industry professionals, and policy makers to submit their abstracts and original research papers based on theoretical and/or empirical research applying rigorous qualitative (i.e. case studies, review articles) and/or quantitative methods (i.e. performance assessment). Published articles are expected to be approximately 8,000 words in length. Topics covered by the Research Topic may include, but are not limited to: • Token-based incentive systems including carbon emissions tracking and offsetting for the promotion of climate-neutral behaviors (climate change adaptation and mitigation) (SDG 13: Climate Action) • Transparency in food origin and production processes leveraging blockchain and data space technology (SDG2: Zero Hunger) • Token-enabled business models for regenerative finance (SDG 8: Decent Work and Economic Growth) • Token-based systems for biodiversity management and protection (SDG 15: Life on Land) • Blockchain-based business and governance models for philanthropy (SDG 17: Partnerships for the Goals) • Blockchain for transparent and accountable governance in sustainability initiatives including cross-border I-Voting and privacy, security, and ethical considerations (SDG 16: Peace, Justice, and Strong Institutions) • Sustainable Transportation, DPP and blockchain technology (SDG 11: Sustainable Cities and Communities) • Decentralized energy systems and renewable energy trading using blockchain (SDG 7: Affordable and Clean Energy) • Blockchain applications in waste management and circular economy (SDG 12: Responsible Consumption and Production) including biodiversity conservation and ecosystem management (SDG 15: Life on Land) • Blockchain-enabled solutions for sustainable supply chain management (SDG 12: Responsible Consumption and Production) • Sustainable agriculture and food transparency and traceability using blockchain (SDG 2: Zero Hunger) including consumer goods (SDG 12: Responsible Consumption and Production) • Social impact of blockchain technology in underserved communities (SDG 10: Reduced Inequalities)
Keywords : Token-based incentive systems, Tokenomics, Blockchain governance models, Decentralized systems, Sustainability aspects of BCT, Social impact of BCT
Important Note : All contributions to this Research Topic must be within the scope of the section and journal to which they are submitted, as defined in their mission statements. Frontiers reserves the right to guide an out-of-scope manuscript to a more suitable section or journal at any stage of peer review.
Topic Editors
Topic coordinators, submission deadlines, participating journals.
Manuscripts can be submitted to this Research Topic via the following journals:
total views
- Demographics
No records found
total views article views downloads topic views
Top countries
Top referring sites, about frontiers research topics.
With their unique mixes of varied contributions from Original Research to Review Articles, Research Topics unify the most influential researchers, the latest key findings and historical advances in a hot research area! Find out more on how to host your own Frontiers Research Topic or contribute to one as an author.

An official website of the United States government
The .gov means it’s official. Federal government websites often end in .gov or .mil. Before sharing sensitive information, make sure you’re on a federal government site.
The site is secure. The https:// ensures that you are connecting to the official website and that any information you provide is encrypted and transmitted securely.
- Publications
- Account settings
Preview improvements coming to the PMC website in October 2024. Learn More or Try it out now .
- Advanced Search
- Journal List
- J Exp Anal Behav
- v.91(2); 2009 Mar
TOKEN REINFORCEMENT: A REVIEW AND ANALYSIS
Token reinforcement procedures and concepts are reviewed and discussed in relation to general principles of behavior. The paper is divided into four main parts. Part I reviews and discusses previous research on token systems in relation to common behavioral functions—reinforcement, temporal organization, antecedent stimulus functions, and aversive control—emphasizing both the continuities with other contingencies and the distinctive features of token systems. Part II describes the role of token procedures in the symmetrical law of effect, the view that reinforcers (gains) and punishers (losses) can be measured in conceptually analogous terms. Part III considers the utility of token reinforcement procedures in cross-species analysis of behavior more generally, showing how token procedures can be used to bridge the methodological gulf separating research with humans from that with other animals. Part IV discusses the relevance of token systems to the field of behavioral economics. Token systems have the potential to significantly advance research and theory in behavioral economics, permitting both a more refined analysis of the costs and benefits underlying standard economic models, and a common currency more akin to human monetary systems. Some implications for applied research and for broader theoretical integration across disciplines will also be considered.
A token is an object or symbol that is exchanged for goods or services. Tokens, in the form of clay coins, first appeared in human history in the transition from nomadic hunter-gatherer societies to agricultural societies, and the expansion from simple barter economies to more complex economies ( Schmandt-Besserat, 1992 ). Since that time, token systems, in one form or another, have provided the basic economic framework for all monetary transactions. From the supermarket to the stock market, any economic system of exchange involves some form of token reinforcement.
Token systems have been successfully employed as behavior-management and motivational tools in educational and rehabilitative settings since at least the early 1800s (see Kazdin, 1978 ). More recently, token reinforcement systems played an important role in the emergence of applied behavior analysis in the 1960–70s ( Ayllon & Azrin, 1968 ; Kazdin, 1977 ), where they stand as among the most successful behaviorally-based applications in the history of psychology.
Laboratory research on token systems dates back to pioneering studies by Wolfe (1936) and Cowles (1937) with chimpanzees as experimental subjects. Conducted at the Yerkes Primate Research Laboratories, and published as monographs in the Journal of Comparative Psychology , these papers describe an interconnected series of experiments addressing a wide range of topics, including discrimination of tokens with and without exchange value, a comparison of tokens and food reinforcers in the acquisition and maintenance of behavior, an assessment of response persistence under conditions of immediate and delayed reinforcement, preference between tokens associated with different reinforcer magnitudes and with qualitatively different reinforcers (e.g., food vs. water, play vs. escape), and social behavior engendered by token reinforcement procedures, to name just a few.
The focus of these early investigations was on conditioned reinforcement—the conditions giving rise to and maintaining the acquired effectiveness of the tokens as reinforcing stimuli. A central question concerned the degree to which tokens, as conditioned reinforcers, were functionally equivalent to unconditioned reinforcers. This emphasis on the conditioned reinforcement value of tokens was maintained in later work by Kelleher (1956) , also with chimpanzees and also conducted at the Yerkes Laboratories (see Dewsbury, 2003 , 2006 , for an interesting historical account of this period at Yerkes). Kelleher's work was notable in that it was the first to place token reinforcement in the context of reinforcement schedules. The emphasis then shifted from response acquisition to maintenance, and from discrete-trial to free-operant methods. It also opened the study of token reinforcement to more refined techniques for assessing conditioned reinforcement value (e.g., chained and second-order schedules). Prior to Kelleher's work, conditioned reinforcement was generally studied in acquisition or extinction, and the effects were weak and transient. With the emerging technology of reinforcement schedules, however, conditioned reinforcement in general, and token reinforcement in particular, was studied in situations that permitted continued pairing of stimuli with food, producing more robust effects.
Kelleher's work suggested another important role of tokens—that of organizing coordinated sequences of behavior over extended time periods. An emphasis on conditioned reinforcement and temporal organization has been carried through in later investigations of token reinforcement with rats ( Malagodi, Webbe, & Waddell, 1975 ) and pigeons ( Foster, Hackenberg, & Vaidya, 2001 ). Token reinforcement procedures have also been extended to the study of choice and self-control ( Jackson & Hackenberg, 1996 ), and to aversive control ( Pietras & Hackenberg, 2005 ), where they have proven useful in cross-species comparisons and to behavioral-economic formulations more generally ( Hackenberg, 2005 ).
Despite periods of productive research activity, the literature on token reinforcement has developed sporadically, with little integration across research programs. And to date, no published reviews of laboratory research on token systems exist. The purpose of the present paper is to review what is known about token reinforcement under laboratory conditions and in relation to general principles of behavior. The extensive literature on token systems in applied settings is beyond the scope of the present paper, although it will consider some implications for applied research of a better understanding of token-reinforcement principles.
Two chief aims of the paper are to identify gaps in the research literature, and to highlight promising research directions in the use of token schedules under laboratory conditions. The paper is organized into four main sections. In Section I, past research on token systems will be reviewed and discussed in relation to common behavioral functions—reinforcement, temporal organization, antecedent stimulus functions, and aversive control. This kind of functional analysis provides an effective way to organize the literature, revealing continuities between token reinforcement and other types of contingencies.
Although resembling other contingencies, token-based procedures have distinctive characteristics that make them effective tools in the analysis of behavior more generally. The present paper considers three areas in which token systems have proven especially useful as methodological tools. Section II discusses the use of token systems in addressing the symmetrical law of effect—the idea that reinforcers (gains) and punishers (losses) can be viewed in conceptually analogous terms, as symmetrical changes along a common dimension. Such a view is foundational in major theoretical accounts of behavior, yet supporting laboratory evidence is surprisingly sparse. Token systems provide a methodological context in which to explore such questions across species and outcome types.
This common methodological context makes token systems useful in a comparative analysis more generally, where minimizing procedural differences is paramount. This is the main focus of Section III, a review and discussion of procedures and concepts in the area of choice and self-control, showing how token systems can be used to bridge key procedural barriers separating research with humans from that with other animals.
Section IV explores the implications of token systems for behavioral economics. Laboratory research in the experimental analysis of behavior has contributed to behavioral economics for over three decades ( Green & Rachlin, 1975 ; Hursh, 1980 , Lea, 1978 ), though with few exceptions ( Kagel, 1972 ; Kagel & Winkler, 1972 ; Winkler, 1970 ), this work has not made direct contact with token reinforcement methods or concepts. This is unfortunate, because token systems have great potential for exploring a wide range of relationships between behavior and economic contingencies, and for uniting the different branches of behavioral economics.
I. Functional Analysis of Token Reinforcement
A token reinforcement system is an interconnected set of contingencies that specifies the relations between token production, accumulation, and exchange. Tokens are often manipulable objects (e.g., poker chips, coins, marbles), but can be nonmanipulable as well (e.g., stimulus lamps, points on a counter, checks on a list). A token, as the name implies, is an object of no intrinsic value; whatever function(s) a token has is established through relations to other reinforcers, both unconditioned (e.g., food or water) or conditioned (e.g., money or credit). As such, a token may serve multiple functions—reinforcing, punishing, discriminative, eliciting—depending on its relationship to these other events. The present review will be organized around known behavioral functions: reinforcement, temporal organization, antecedent stimulus functions (discriminative and eliciting), and punishment.
Reinforcing Functions
Tokens are normally conceptualized as conditioned reinforcers, established as such through their relationship to other reinforcers. In the studies by Wolfe (1936) and Cowles (1937) , the chimpanzees were trained to deposit poker chips into a vending-machine apparatus (as shown in the top panel of Figure 1 ) for a variety of unconditioned reinforcers (e.g., grapes, water). Initially, depositing was modeled by the experimenter; exchange opportunities were continuously available and each token deposit was reinforced immediately with food, ensuring contiguous token–food pairings. The importance of such pairings was demonstrated in subsequent conditions, in which depositing was brought under stimulus control: The chimps were trained to respond differentially with respect to tokens with exchange value (white tokens, exchangeable for grapes) and without exchange value (brass tokens, exchangeable for nothing). Such differential behavior was evidenced by (a) exclusive preference for white over brass tokens, and (b) extinction of depositing brass tokens. Periodic tests throughout the study verified the selective reinforcing effectiveness of the white chips: When white and brass tokens were scattered on the floor, the chimpanzees picked up and deposited only the white (food-paired) tokens.

Subject and apparatus used in Wolfe (1936) . Top: Chimpanzee depositing a poker-chip token into token receptacle. Bottom: Outside view of experimental apparatus. See text for details. From Yerkes (1943) . Property of the Yerkes National Primate Research Center, Emory University. Reprinted with permission.
Acquisition and Maintenance of New Behavior
Once token depositing had been established, the chimpanzees were then trained to operate a weight-lifting apparatus for access to tokens. The animals lifted a bar from a horizontal to a vertical position, the force of which could be adjusted by adding weight to the bar, a stem of which extended outside the work chamber (see bottom of Figure 1 ). The main question was whether tokens could establish and maintain behavior as effectively as food reinforcers. Several of Wolfe's (1936) experiments addressed this question, systematically comparing token-maintained behavior with food-maintained behavior. In one experiment, for example, sessions in which tokens were earned alternated with sessions in which food was earned. Tokens were earned after 10 responses and exchanged immediately for food. For 3 of 4 subjects, there was little difference in the time required to complete 10 trials in the token versus food sessions. For the other subject, the time was considerably shorter in the food sessions. Similar results were obtained using a different procedure, in which the weight requirement increased in fixed increments with each reinforcer (an early type of escalating, or progressive, schedule). Again, for all but one subject, there was essentially no difference in the terminal weights achieved under token and food conditions. For the remaining animal, the weights were slightly but consistently higher under food than under tokens, indicating greater efficacy of food over token reinforcers.
Using an apparatus similar to Wolfe (1936) , as well as some of the same subjects, Cowles (1937) compared the effects of tokens (poker chips) and food (raisins and seeds) under a variety of experimental arrangements. In one experiment, for example, chimpanzees were trained on a two-position discrimination task, in which the position of the alternative associated with tokens was alternated repeatedly until it was selected exclusively. Tokens could be exchanged for food at the end of the session, which lasted either 10 or 20 trials. The discrimination was rapidly learned by 2 of 3 subjects on this procedure, and improved with repeated testing (from 1 st to 2 nd session within a day) and across repeated reversals. In another experiment, the same 2 chimpanzees were trained on a 5-position discrimination-learning task, in which correct responses produced either tokens later exchangeable for food, or food alone. The discrimination was learned rapidly for both subjects, and appeared to improve over time. One subject learned better (higher accuracy and fewer trials to meet criterion) with food than with tokens, while the other learned equally well with both reinforcers. However, even for the latter, token sessions were characterized by more “off-task” behavior, especially early in the exchange ratio. Under conditions in which a limited number of trials were provided, learning was more rapid under food than under token-food conditions for both subjects. Thus, despite little difference in asymptotic performance under both reinforcers, learning proceeded somewhat more rapidly with food than with tokens.
Subsequent experiments by Cowles (1937) showed that the tokens derived their reinforcing capacity through their relation to the terminal (food) reinforcers. In one experiment along these lines, Cowles compared the relative efficacy of food-paired and nonpaired tokens to that of food on the accuracy of a kind of delayed match-to-sample discrimination under conditions of immediate and delayed exchange. In delayed exchange, the tokens accumulated in a tray across a block of trials and were deposited in a room adjacent to that in which the tokens were earned. Accuracy was somewhat higher under food–token than nonfood–token conditions. In immediate exchange, the chimpanzees earned and exchanged tokens in the same room, and deposits were required to initiate the next trial. Accuracy increased under both food-paired and nonfood-paired token conditions, markedly so under paired conditions. Under conditions in which tokens were compared to food directly, accuracy was slightly but consistently better under food than food-paired token conditions, and significantly better under food than nonfood-paired token conditions.
In sum, the results of these experiments demonstrate that tokens function as effective reinforcers, albeit somewhat less effective than unconditioned reinforcers. This differential effectiveness of tokens versus food reinforcers is to be expected, if the tokens are functioning as conditioned reinforcers. The research also showed that tokens derive their reinforcing functions through relations to food. While this, too, may hardly be surprising, few studies have demonstrated the necessary and sufficient conditions for tokens to function as conditioned reinforcers. Indeed, little is known about the training histories needed to establish reinforcing functions of tokens. Is explicit token-food pairing necessary? Must conditional stimulus (CS) functions of tokens be established before they will function as effective reinforcers? In short, what are the optimal conditions for establishing and maintaining tokens as reinforcers? These are fundamental but unresolved questions requiring additional research.
Generalized Reinforcing Functions
When tokens are paired with multiple terminal reinforcers, they are said to be generalized reinforcers ( Skinner, 1953 ). It is common in token systems with humans, for example, to include a store in which tokens can be exchanged for an array of preferred items and activities. Such generalizability should greatly enhance the durability of tokens, making them less dependent on specific motivational conditions, although there is surprisingly little empirical support for this. Perhaps the closest was an early experiment by Wolfe (1936) . Three chimpanzees were given choices between black tokens (exchangeable for peanuts) and yellow tokens (exchangeable for water) under conditions of 16-hr deprivation from food or water (alternating every two sessions). All 3 subjects generally preferred the tokens appropriate to their current deprivation conditions, though the preference was not exclusive. Two additional subjects were therefore run under 24-hr deprivation conditions and were permitted free access to the alternate reinforcer for 1 hr prior to each session. Eliminating the motivation for one reinforcer was thought to heighten the motivation for the other. These subjects generally preferred the deprivation-specific reinforcer, and did so more strongly than the 3 subjects studied under more modest deprivation conditions.
Had a third token type been paired with both food and water, generalized reinforcing functions of the tokens could then have been evaluated. To date, however, generalized functions of tokens have yet to be explored. This remains a key topic of research on token reinforcement, especially in light of the immense theoretical importance attached to the concept of generalized reinforcement ( Skinner, 1953 ). What types of experiences are necessary in establishing generalized functions? Once established, how do generalized reinforcers compare to more specific reinforcers? Are generalized reinforcers more durable than specific reinforcers? Are generalized reinforcers more resistant to extinction, or other disruptions, than more conventional reinforcers? Will generalized reinforcers be preferred to specific reinforcers? Will generalized reinforcers generate more work to obtain them? Are generalized reinforcers more aversive to lose than more specific reinforcers? In short, under what conditions will generalized reinforcers prove more valuable than specific reinforcers? These are just a few of the many foundational questions brought within the scope of an experimental analysis of generalized reinforcement.
Bridging Reinforcement Delay
Another function commonly ascribed to conditioned reinforcers is that of bridging temporal gaps between behavior and delayed reinforcers ( Kelleher, 1966a ; Skinner, 1953 ; Williams, 1994a , b ). In an early experiment along these lines, Wolfe (1936) showed that tokens could maintain behavior in the face of extensive delays to food reinforcement. Four conditions were studied: (1) tokens were earned immediately, but could not be exchanged until after a delay (immediate token, delayed exchange/food); (2) food was earned for each response but delivered after a delay (no token, delayed food); (3) same as 2, except that brass chips were available during the delays (nonpaired token, delayed food); and (4) tokens were earned and could be deposited immediately, but food was delivered only after a delay (immediate token/exchange, delayed food). This latter condition differed from (1) in that no tokens were present during the delay interval.
In all four conditions, the delays increased progressively with each food delivery, until a breakpoint was reached—a point beyond which responding ceased (5 min without a response). Responding was clearly strongest in Condition 1 (immediate token, delayed exchange/food) for all 4 chimpanzees studied, reaching delays of 20 min and 1 hr for 2 subjects. For the other 2 subjects, breakpoints under Condition 1 were undefined, both in excess of 1 hr. The breakpoints under Conditions 2–4 were generally short (less than 3 min) for all subjects and did not differ systematically across conditions. Interestingly, there was evidence of pausing under the latter conditions, but not under Condition 1, indicating that the immediate delivery of a token helped sustain otherwise weak behavior temporally remote from food.
Performances in Conditions 1 and 4 are relevant to accounts of conditioned reinforcement based on concepts of marking —the facilitative effects of response-produced stimulus changes. According to this view, the rate-enhancing effects of stimuli paired with food arise not from the temporal pairing of stimulus with food (conditioned reinforcement), but from the response-produced stimulus change, which is said to mark, or make more salient, the response that produces the reinforcer ( Lieberman, Davidson, & Thomas, 1985 ; Williams, 1991 ). If the tokens function mainly to mark events in time, then no differences would be expected under Conditions 1 and 4, as both involve similar response-produced stimulus changes: immediate token presentation. Conversely, if tokens function as conditioned reinforcers via temporal pairings with food, then greater persistence would be expected under Condition 1 than Condition 4. The higher breakpoints obtained under Condition 1 versus Condition 4 are clearly more consistent with the latter view, suggesting that the tokens were indeed serving as conditioned reinforcers—that their function depended on explicit pairing with food—rather than merely as temporal markers.
Similar results were reported in a nontoken context by Williams (1994b , Experiment 1), with response acquisition under delayed reinforcement. Rats' lever presses produced food after a 30-s delay. In marking conditions, each lever press produced a 5-s tone at the outset of the 30-s delay interval. In conditioned-reinforcement conditions, each lever press produced a 5-s tone at the beginning and end of the delay interval. Responding was acquired more rapidly under the latter conditions, showing the importance of the temporal pairing of the stimulus with food. In a second experiment, Williams compared acquisition under these latter conditions to that under conditions in which the tone remained on during the entire 30-s delay interval, designed to assess the bridging functions of the stimulus. Although both conditions included temporal pairing of tone and food, the bridging conditions proved less effective than conditions in which the tone was presented only at the beginning and end of the interval. Williams attributed these differences to the higher rate of food delivery (per unit time) in the presence of the briefer signals.
Some conditions in Wolfe's (1936) experiment also bear on potential bridging functions of the tokens. For the 2 subjects whose responding was maintained at 1-hr delays under Condition 1, delays exceeding 1 hr were examined. As before, the delay increased systematically across sessions. Because it was impractical to retain the subjects in the workspace under such long delays, the chimpanzees were permitted to leave the experimental space (and the token) 5 min after making a response and were returned 5 min prior to the end of the delay interval. Both subjects continued to respond at the maximum delays tested: 5 hrs for one subject, 24 hrs for the other. This suggests that the continued presence of the token was not essential to the reinforcing value of the tokens, as long as temporal pairing of tokens and food was intact. This conclusion must be tempered by the fact that these conditions were conducted following extended exposure to conditions in which the tokens filled the temporal gap, and may therefore have depended in part on that history. Additional research is needed to isolate the necessary and sufficient conditions under which response-produced stimuli enhance tolerance for delayed reinforcers. It should be apparent from this brief review, however, that token reinforcement procedures are well suited to the task.
Temporal Organization: Schedules of Token Reinforcement
Despite the conceptual importance of the pathbreaking work by Wolfe (1936) and Cowles (1937) , research on token reinforcement lay dormant for approximately two decades. Kelleher revived research on token reinforcement in the 1950s, combining it with the emerging technology of reinforcement schedules. A fundamental insight of Kelleher's (and, later, Malagodi's) research was the conceptualization of a token reinforcement contingency as a series of three interconnected schedule components: (a) the token-production schedule , the schedule by which responses produce tokens, (b) the exchange-production schedule , the schedule by which exchange opportunities are made available, and (c) the token-exchange schedule , the schedule by which tokens are exchanged for other reinforcers. Research has shown that behavior is systematically related both to the separate and combined effects of these schedule components. This research will be reviewed below, along with a discussion of the relevance of such work to other schedules used to study conditioned reinforcement.
Token-Production Schedules
The first demonstration of schedule-typical behavior under token reinforcement schedules was reported by Kelleher (1956) . Two chimpanzees were first trained to press a lever on fixed-ratio (FR) and fixed-interval (FI) schedules of food reinforcement, in which responses produced food after a specified number of responses (FR) or the first response after a specified period of time (FI). Once lever pressing was established, the subjects were then trained to deposit poker-chip tokens in a receptacle for food. Unlike the earlier studies by Wolfe and Cowles, in which exchange responses were enabled or prevented by opening or closing a shutter in front of the token receptacle, Kelleher brought exchange responding under discriminative control. Deposits in the presence of an illuminated window produced food, whereas deposits when the window was dark did not. When depositing was under stimulus control, the animals were taught to press a lever to obtain poker chips. Under both simple and multiple schedules, Kelleher found that FR and FI schedules of token production generated response patterning characteristic of FR and FI schedules of food production.
In a subsequent study, Kelleher (1958) performed a more extensive analysis of FR schedules of token reinforcement. Responses in the presence of a white light produced tokens (poker chips) that could be exchanged for food during an exchange period (signaled by a red light) at the end of the session. With the exchange schedule held constant at FR 60 (i.e., 60 tokens were required to produce the exchange period) and the token-exchange schedule held constant at FR 1 (i.e., once the exchange period was reached, each of the 60 token deposits produced a food pellet), the token-production ratio was varied from FR 60 to FR 125 gradually over the course of 10 sessions. Responding was characterized by a two-state pattern: alternating bouts of responding and pausing, similar to that seen under FR schedules of food reinforcement. The break-run pattern persisted in extinction with token schedules discontinued, an effect also seen with FR schedules of food reinforcement ( Ferster & Skinner, 1957 ).
Malagodi (1967a , b , c) extended Kelleher's research on token-reinforced behavior, using rats rather than chimpanzees as subjects and marbles rather than poker chips as tokens. The basic apparatus is depicted in Figure 2 , which shows a photograph of the front wall of the chamber and a diagram of the token receptacle. Lever presses produced marbles, dispensed into the token hopper. During scheduled exchange periods (denoted by a clicker and local illumination of the token receptacle), depositing the tokens in the token receptacle produced food, dispensed into the food hopper.

Photograph of intelligence panel and diagram (side view) of token receptacle used in Malagodi's research with rats as subjects and marbles as tokens. See text for details. From Malagodi (1967a). Reprinted with permission.
In several experiments, Malagodi (1967 a , b , c) examined response patterning under various token-production schedules. Illustrative cumulative records from Malagodi (1967a) are shown in Figure 3 , with VI and FR token-production responding on the left and right panels, respectively. The exchange-production and token-exchange (deposit) schedules were held constant at FR 1. Consistent with Kelleher's findings, FR token-production patterns showed a high steady rate with occasional preratio pausing, characteristic of FR patterning with food reinforcers. Similarly, VI token-production responding was characterized by a lower but steady rate, resembling response patterning on simple VI schedules. The token-exchange (deposit) records show a relatively constant rate, appropriate to the FR-1 exchange schedule. Similar schedule-typical response patterns under FR and VI token-production schedules were reported by Malagodi (1967 b , c) .

Representative cumulative records for rats exposed to token-reinforcement schedules. Left panels: VI 1-min token-production schedules. Right panels: FR 20 token-production schedules. Pips indicate token deliveries. Exchange-production and token-exchange schedules (labeled deposit in the figure) were both FR 1. From Malagodi (1967a). Reprinted with permission.
More recently, Bullock and Hackenberg (2006) expanded the analysis of token-production effects, parametrically examining behavior under both FR token-production and exchange-production schedules under steady-state conditions. The subjects were pigeons and the tokens consisted of stimulus lamps (LEDs) mounted in a horizontal array above the response keys in an otherwise standard experimental chamber. With the token-exchange schedule held constant at FR 1, the token-production ratio was varied systematically across conditions from FR 25 to FR 100 at each of three different exchange-production FR values (2, 4, and 8). Figure 4 shows response rates on the token-production schedule as a function of the token-production and exchange-production ratios. In general, response rates declined with increases in the token-production FR (left panels), especially at the higher exchange-production ratios (as denoted by symbols), an effect that corresponds well to that reported with simple FR schedules ( Mazur, 1983 ). (Exchange-production effects [right panels] will be taken up in the next section.) There was only suggestive evidence of such an effect in Kelleher (1958) , as the token-production FR was increased incrementally over approximately 10 sessions, with data shown only for the lowest and highest ratio.

Token-production and exchange-production response rates as a function of FR token-production ratio (left panels) and exchange-production ratio (right panels) of 4 pigeons across the final five sessions of each condition. Note that the graphs in left and right panels are derived from the same data, plotted differently to highlight the impact of token-production and exchange-production ratios. Different symbols represent different ratio sizes for the exchange-production ratios (left panels) and token-production ratios (right panels). Unconnected points represent data from replicated conditions; error bars represent standard deviations. From Bullock & Hackenberg (2006). Copyright 2006, by the Society for the Experimental Analysis of Behavior, Inc. Reprinted with permission.
Taken together, the results of Kelleher (1958) with chimpanzees and poker-chip tokens, of Malagodi (1967a , b , c) with rats and marble tokens, and of Bullock and Hackenberg (2006) with pigeons and stimulus-lamp tokens, show that performance under schedules of token production resemble, in both patterning and rate, performance under schedules of food reinforcement ( Mazur, 1983 ), suggesting that tokens do indeed serve as conditioned reinforcers. Token-reinforced behavior is also modulated, however, by the exchange-production schedule that determines how and when exchange opportunities are made available. In the Bullock and Hackenberg experiment, for example, response rates were generally low in the early segments of the exchange-production cycle, but increased throughout the cycle, as additional tokens were earned. Response rates were thus a joint function of the token-production schedule and exchange-production schedule.
Exchange-Production Schedules
More direct evidence of exchange-production effects comes from studies in which the exchange-production schedule value or type is directly manipulated. For example, Webbe and Malagodi (1978) compared rats' response rates under VR and FR exchange-production schedules while holding constant the token-production schedule (FR 20) and token-exchange schedule (FR 1) requirements. In other words, a fixed number of lever presses were required to produce tokens (marbles) and to exchange tokens for food, but groups of tokens were required to produce an exchange period. In some conditions, the exchange-production schedule was FR 6 (six tokens required to produce exchange periods), and in others VR 6 (an average of six tokens required to produce exchange periods). Responding was maintained under both schedule types, but at higher rates under the VR schedules. Most of the rate differences were due to differential postexchange pausing produced by the schedules. The FR exchange-production schedule generated extended pausing early in the cycle giving way to higher rates later in the cycle—the “break-run” pattern characteristic of simple FR schedules. This bivalued pattern was greatly attenuated under the VR exchange-production schedule.
Foster et al. (2001) reported similar effects with FR and VR exchange ratios varied over a much wider range of values. Pigeons' keypecks produced tokens (stimulus lamps) according to an FR 50 schedule and exchange opportunities according to FR and VR schedules. Exchange schedule type (FR or VR) was varied within a session in a multiple-schedule arrangement, whereas the schedule value was systematically varied from 1 to 8 across conditions (requiring between 50 and 400 responses per exchange period). Response rates were consistently higher, and pausing consistently shorter, under VR than under comparable FR exchange-production schedules. Moreover, rates were more sensitive to exchange-production ratio size under FR than under VR schedules. These effects are portrayed in Figure 5 , which shows response rates as a function of exchange-production ratio size and schedule type. Here and in Bullock and Hackenberg (2006) , right panels of Figure 4 , response rates decreased with increases in the size of the FR exchange-production schedule. These effects on response rates, as well as those on preratio pausing (not shown), resemble effects typically obtained with simple FR and VR schedules, suggesting that extended segments of behavior may be organized by exchange schedules in much the same way that local response patterns are organized by simple schedules.

Token-production response rates of 3 pigeons as a function of exchange-ratio size and schedule type: FR (open circles) and VR (closed circles) over the final five sessions of each condition. Unconnected points denote data from replicated conditions. Error bars indicate the range of values contributing to the means. From Foster, Hackenberg, & Vaidya (2001) . Copyright, 2001 by the Society for the Experimental Analysis of Behavior, Inc. Reprinted with permission.
Viewed in this way, token schedules closely resemble second-order schedules of reinforcement ( Kelleher, 1966a ), in which a pattern of behavior generated under one schedule (the first-order, or unit schedule) is treated as a unitary response reinforced according to another schedule (the second-order schedule). In a token arrangement, first-order (token-production) units are reinforced according to a second-order (exchange-production) schedule. If the exchange-production schedules are acting as higher-order schedules, then the rate and patterning of token-production units should be schedule-appropriate. In the Foster et al. (2001) study, for example, the rate of FR 50 token-production units was higher under VR than under FR exchange, owing mainly to extended pausing in the early segments under the FR exchange-production ratio. That the functions relating pausing and response rates to schedule value and type also holds at the level of second-order schedules, suggests that token-production units might profitably be viewed as individual responses themselves reinforced according to a second schedule, supporting a view of token schedules as a kind of second-order schedule.
Further evidence of exchange-schedule modulation over token-reinforced behavior comes from studies utilizing time-based exchange-production schedules. In a study by Waddell, Leander, Webbe, and Malagodi (1972) , rats' lever presses produced tokens (marbles) under FR 20 schedules in the context of FI exchange schedules, which varied parametrically from 1.5 to 9 min across conditions. The first token produced after the FI exchange interval timed out produced an exchange period (light and clicker) in the presence of which each token could be exchanged for a food pellet.
Token-production patterns were characterized by bivalued (break-run) patterns, such that responding either occurred at high rates or not at all, similar to that seen under simple FR schedules. These patterns are illustrated in Figure 6 , which shows cumulative records of responding across the exchange-production FI cycle (within each panel) and as a function of exchange-schedule FI durations (across panels). The temporal pattern of token-production units within an exchange cycle was positively accelerated, similar to that seen under simple FI schedules, with longer pauses near the beginning of a cycle giving way to higher rates as the exchange period grew nearer. Overall rates decreased and quarter-life values increased with increases in the FI exchange schedule, similar to that seen under FI schedules of food presentation. The overall pattern of results is consistent with that seen under second-order schedules of brief stimulus presentation ( Kelleher, 1966b ), further supporting the view that token-production schedules are units conditionable with respect to second-order schedule requirements.

Representative cumulative records for one rat exposed to FR 20 token-production schedules as a function of exchange-production schedules: FI 1.5 min (top), FI 4.5 min (middle) and FI 9 min (bottom). Token deliveries are indicated by pips, exchange periods by resets of the response pen. From Waddell, Leander, Webbe, & Malagodi (1972). Reprinted with permission.
Token-Exchange Schedules
In the studies reviewed thus far, the token-production and/or exchange-production schedules have been manipulated in the context of a constant token-exchange schedule. That is, once the exchange period is produced, each token-exchange response removes a token and produces food. In the only published study in which the token-exchange schedule was manipulated ( Malagodi, Webbe, & Waddell, 1975 ), rats' lever presses produced marble tokens according to an FR 20 token-production schedule, and exchange periods according to either FR 6 (2 rats) or FI t -s schedules (2 rats). The token-exchange ratio was systematically manipulated across conditions, such that multiple tokens were required to produce each food pellet.
Figure 7 shows initial-link response rates across successive token-production FRs as a function of the terminal-link token schedule for the pair of FR subjects (divided by phase for Rat T-22). Response rates increased in proximity to the terminal-link exchange schedule, and were ordered inversely with the token-exchange FR. Similar effects were observed in rats exposed to the FI token-exchange schedule (not shown). Together, these results are consistent with those obtained with chained schedules more generally ( Ferster & Skinner, 1957 ; Hanson & Witoslawski, 1959 ). Moreover, the token-production FR units resembled simple FR units, consistent with findings in the literature on second-order schedules ( Kelleher, 1966a ), suggesting that token-production schedules create integrated response sequences reinforced according to second-order exchange-production and token-exchange schedules.

Response rates across successive FR token-production segments as a function of token-exchange FR for 2 rats. Overall mean response rates are shown on the right-hand side of each plot. From Malagodi, Webbe, & Waddell (1975). Copyright 1975, by the Society for the Experimental Analysis of Behavior, Inc. Reprinted with permission.
In sum, behavior under token reinforcement schedules is a joint function of the contingencies whereby tokens are produced and exchanged for other reinforcers. Other things being equal, contingencies in the later links of the chain exert disproportionate control over behavior. Token schedules are part of a family of sequence schedules that includes second-order and extended chained schedules. Like these other schedules, token schedules can be used to create and synthesize behavioral units that participate in larger functional units under the control of other contingencies. Token schedules differ, however, from these other sequence schedules in several important ways.
First, unlike conventional chained and second-order schedules, the added stimuli in token schedules are arrayed continuously, and each token is paired with food during exchange periods. These token–food relations may render token schedules more susceptible to stimulus–stimulus intrusions than other sequence schedules, as discussed below in relation to antecedent stimulus functions. Second, unlike other sequence schedules, token schedules provide a common currency (tokens) in relation to which reinforcer value can be scaled. This makes the procedures useful in exploring symmetries between the behavioral effects of losses and gains, as discussed in Section II. Third, the interdependent components of a token system (production and exchange for other reinforcers) closely resemble the point or money reinforcement systems commonly used in laboratory research with humans. This makes token procedures especially useful in narrowing the methodological gulf separating research with humans from that with other animals, as discussed in Section III. Finally, added stimuli (number of tokens) in token schedules are correlated not only with temporal proximity to food (as in other sequence schedules) but with amount of reinforcement available in exchange. This makes token schedules especially useful tools in studying economic concepts, such as unit price, as discussed in Section IV.
Token Accumulation
Another distinctive feature of token reinforcement schedules is the accumulation of tokens prior to exchange. In an early experiment by Cowles (1937) , chimpanzees were studied under three conditions: (1) tokens were earned and accumulated but could not be exchanged until the end of the interval, (2) food was earned and accumulated but could not be consumed until the end of the interval, and (3) food was earned but did not accumulate and could not be consumed until the end of the interval. Subjects were trained under three different FI exchange values (1, 3, and 5 min) before the final value of 10 min was reached. For one subject, responding was maintained under (1) and (2) but not under (3). For the other subject, responding was weakly but consistently maintained under (1) but not under (2) or (3). For both subjects, the number of tokens earned decreased throughout the interval (counter to the typical FI pattern), an effect attributed to “token satiation.” In support of this notion, when tokens (5, 15, or 30) were provided at the beginning of the session, token-maintained behavior decreased, such that an approximately equal number of tokens (free + earned) were obtained. To complicate matters, however, the total number of tokens or grapes decreased across sessions, suggesting perhaps additional motivational factors.
More recently, Sousa and Matsuzawa (2001) reported accumulation of tokens (coins) by chimpanzees in a match-to-sample procedure. Correct responses produced a token that could be exchanged at any time by inserting into a slot. Once inserted, a single exchange response would deliver food reinforcement. Performance initially established via food reinforcement showed little or no deterioration when token reinforcers were substituted for food. The token reinforcement contingency was also sufficient to generate accurate responding in new discriminations. Thus, consistent with the older studies by Cowles (1937) and Wolfe (1936) , token reinforcement contingencies appear to be effective in establishing and maintaining discriminative performance in a variety of tasks. The authors also noted “spontaneous” savings of tokens in one of the chimps—spontaneous in the sense that it was not strictly required by the contingencies. The authors reasoned that such behavior was adaptive, requiring less effort in the long run. That is, accumulating groups of tokens before exchange means that the exchange response (in this case, moving from one part of the chamber to another) need only be executed once rather than repeatedly, increasing the overall reinforcer density (number of reinforcers per unit time). Although plausible, their procedures do not permit a clear test of this notion.
Yankelevitz, Bullock and Hackenberg (2008) examined patterns of token accumulation under a variety of ratio-based token-production and exchange schedules, permitting clearer specification of the relative costs of accumulating and exchanging tokens. Cycles began with the illumination of a token-production key. Satisfying an FR on this key produced a token and simultaneously illuminated a second key, the exchange-production key. Satisfying the exchange-production ratio produced the exchange period, during which all of the tokens earned that cycle could be exchanged for food. Unlike the extended-chained schedules reviewed above, in which token-production and exchange-production schedules were arranged sequentially in chain-like fashion, the token-production and exchange-production ratios here were arranged concurrently after the first token had been earned, providing subjects with a choice between accumulating additional tokens and moving directly to the exchange period.
The token-production and exchange-production ratios were systematically varied across conditions, with the exchange-production ratio manipulated at three different token-production ratios. Summary data are shown in Figure 8 . The top panel shows percentage of cycles in which accumulation occurred (two or more tokens prior to exchange) as a function of FR exchange-production size, averaged across 3 subjects. The bottom panel shows the average number of tokens accumulated per exchange, also expressed as a function of FR exchange-production size. The different FR token-production values are displayed separately (denoted by different symbols and data paths). In general, accumulation varied directly with the exchange-production ratio and inversely with the token-production ratio. In other words, as the costs associated with producing the exchange period increased, more tokens were accumulated prior to exchange. Conversely, as the costs of producing each token increased, fewer tokens were accumulated.

Mean token accumulation as a function of FR exchange-production ratio. Top panel: Percentage of cycles in which multiple tokens accumulated prior to exchange. Bottom panel: Mean tokens accumulated per exchange period. The different symbols represent different token-production FR sizes. Data are based on the final five sessions per condition, averaged across 3 subjects. (Adapted from Yankelevitz, Bullock, & Hackenberg, 2008) .
Viewing the tokens as conditioned reinforcers, the results are consistent with prior research on reinforcer accumulation ( Cole, 1990 ; Killeen, 1974 ; McFarland & Lattal, 2001 ), but over a wider parametric range of conditions. Moreover, as Cole and others have noted, accumulation procedures involve tradeoffs between short-term and longer-term reinforcement variables. Going immediately to exchange minimizes the upcoming reinforcer delay, but at the expense of increasing the overall response:reinforcer ratio. Conversely, accumulation increases the delay to the upcoming reinforcer, but reduces the number of responses per unit of food (unit price). Token accumulation procedures thus bear on current debates over the proper time scale over which behavior is related to reinforcement variables. This topic will be discussed in more detail in Section IV.
Antecedent Stimulus Functions of Tokens
The evidence reviewed above strongly suggests conditioned reinforcing functions of the tokens. As stimuli temporally related to other reinforcers, however, tokens acquire antecedent functions as well, discriminative and eliciting. Research bearing on these functions will be addressed in this section.
Discriminative Functions
In the Kelleher (1958) study reviewed above, low response rates in the early token-production segments gave way to high and roughly invariant rates in the later segments (in closer proximity to the exchange period and food). Weak responding in the early links was attenuated by providing free tokens at the outset of the session, suggesting that the tokens were serving a discriminative function, signaling temporal proximity to the exchange periods and food. Thus, despite no change in the schedule requirements (the same number of responses were required to produce tokens and exchange periods), responding was strengthened by arranging stimulus conditions more like those prevailing later in the cycle. The discriminative functions of stimuli early in the sequence parallels effects seen on extended-chained schedules with ratio components ( Jwaideh, 1973 ; see review by Kelleher & Gollub, 1962 ).
Similar discriminative functions were likely at work in the so-called token satiation effect reported by Cowles (1937) , in which providing free tokens at the beginning of a session resulted in fewer tokens earned. Recall that responding decreased throughout the interval, such that the stimulus conditions late in the interval controlled low rates of behavior. More direct evidence of discriminative effects of tokens on accumulation comes from the Yankelevitz et al. (2008) study, described in the preceding section. When the tokens were removed but all other contingencies held constant, accumulation decreased markedly, suggesting that the tokens were discriminative for further token earning.
Eliciting Functions
In addition to reinforcing and discriminative functions, pairing tokens and food may also establish CS functions of the tokens. In one of Cowles' (1937) discrimination experiments, food-paired tokens were provided for correct responses and nonpaired tokens for incorrect responses. In addition to serving as a more effective reinforcer, the paired but not the unpaired tokens evoked strong token-directed consummatory responses. The chimpanzees came to respond to the paired tokens as if they were food, putting them into their mouths, and so on. Such token-directed consummatory behavior has also been reported in other studies with manipulable tokens: poker chips ( Kelleher, 1958 ), marbles ( Malagodi, 1967d ), and ball bearings ( Boakes, Poli, Lockwood, & Goodall, 1978 ; Midgley, Lea, & Kirby, 1989 ).
In the Midgley et al., (1989) study, for example, rats were trained to deposit ball bearings in a floor receptacle by reinforcing precursor responses (e.g., handling, rolling tokens toward the receptacle) with food. In two experiments, depositing was successfully trained in 13 of 15 rats. In addition to the reinforced response, however, the authors noted frequent instances of “misbehavior,” defined as an excess frequency of precursor responses. That such behavior continued to occur despite postponing food deliveries suggests the involvement of evocative or eliciting functions of the tokens. A procedural analysis of token procedures lends plausibility to this account. Tokens are closely coupled with exchange periods and food throughout most token-reinforcement contingencies, ensuring repeated food–token pairings. In addition to the close temporal pairing of tokens and food during exchange periods, the presentation and accumulation of tokens on many token procedures is temporally correlated with food, differentially signaling delays to food. Thus, apart from the discriminative functions noted above, tokens may also evoke or elicit behavior based on their temporal relations to food.
Such token-evoked behavior is probably more likely with manipulable tokens such as marbles and poker chips than with nonmanipulable tokens such as lights. Manipulable tokens are handled in various ways throughout the sequence of earning and exchanging them for food, and this physical contact with the tokens then becomes part of the behavior engendered by the procedures. With nonmanipulable tokens, on the other hand, such physical contact with the tokens is permitted but not required by the contingencies. Although pigeons have been known to orient to and occasionally peck at token lights, such token-directed behavior occurs much less often than with manipulable tokens.
Behavior evoked or elicited by tokens is an interesting and important topic in its own right, and may play an important role in a comprehensive analysis of token systems. Because such behavior is often incompatible with behavior reinforced by tokens, however, it may be desirable when examining primarily operant functions of tokens to use nonmanipulable tokens, less susceptible to intrusions from embedded stimulus–food relations. Specific methodological choices therefore depend on the types of questions asked.
Punishing Functions
If tokens function as conditioned reinforcers, then the loss of tokens should function as conditioned punishers. Such token-loss contingencies, also known as response-cost contingencies, are common in application and in everyday life, but until recently have not been an explicit topic of laboratory investigation with nonhumans. The main obstacle appears to be methodological. With manipulable tokens, such as marbles or poker chips, the tokens are in a subject's possession until they are exchanged for food; there is no obvious way to remove a token once it is has been earned. With nonmanipulable tokens, such as lights, however, tokens are earned and accumulated but are not physically in a subject's possession. Removing a token is just as straightforward as presenting one, by extinguishing rather than illuminating a token light. This makes such procedures especially well-suited to the study of conditioned punishment via token loss.
In the first published study along these lines, Pietras and Hackenberg (2005) examined token-loss contingencies in a multiple-schedule arrangement with pigeons. Tokens consisted of stimulus lights, mounted in a horizontal array above the response keys (as described above). Identical VR 4 (RI 30 s) schedules of token reinforcement operated in both components, such that tokens were produced on average every 30 s, and exchanges were produced on average following every four tokens. A conjoint FR schedule of token loss was arranged in one component (punishment component), such that every n responses (either 10 or 2, in different conditions) removed a token from the token array.
Response rates were differentially suppressed in the punishment component, demonstrating a clear punishment function of token loss. Because both the exchange-production and token-loss schedules were ratio based, exchanges and food were infrequent in the punishment component. Despite extremely low rates of food reinforcement, responding was not completely suppressed, reaching approximately 30–40% of baseline (unpunished) levels, even under the most stringent (FR 2) token-loss schedule. Only when the tokens were no longer produced under extinction conditions was responding eliminated. This suggests that the mere production of tokens as conditioned reinforcers maintained behavior even when they were only rarely exchanged for food. To test this, Pietras and Hackenberg (2005) conducted an additional condition, termed exchange extinction , in which the token-loss contingency was suspended—permitting production and accumulation of tokens—but tokens could not be exchanged for food. Exchange responses turned off tokens but did not produce food. Response rates under these conditions stabilized well above rates in the extinction condition and at approximately the same levels as in the punishment conditions, suggesting that responding was maintained by the production and accumulation of tokens as conditioned reinforcers even in the signaled absence of food. These effects parallel those seen with other conditioned reinforcers ( Horney & Fantino, 1984 ).
Overall, the results are consistent with prior findings with human subjects: Response-contingent token loss suppresses behavior on which it is contingent ( Munson & Crosbie, 1998 ; O'Donnell, Crosbie, Williams, & Saunders, 2000 ; Weiner, 1962 , 1963 , 1964 ). Contingent token loss also produces indirect effects on behavior, such as contrast ( Crosbie, Williams, Lattal, Anderson, & Brown, 1997 ), comparable to that seen with electric-shock punishment ( Brethower & Reynolds, 1962 ). Together with the Pietras and Hackenberg (2005) findings with pigeons, the results with human subjects appear to support an interpretation based on negative punishment (i.e., punishment via token loss). In negative-punishment studies, however, suppression due to punishment is confounded with changes in reinforcement density that normally accompany response suppression. That is, because negative punishment, by definition, involves reinforcer loss, reductions in responding may be attributed not to a direct punishment effect, but to concomitant changes in reinforcer density.
To overcome this interpretive difficulty, Raiff, Bullock, and Hackenberg (2008) compared response suppression under token-loss contingencies to that under yoked schedules with comparable rates of food density. Like the Pietras and Hackenberg (2005) study, a multiple-schedule format was used with pigeons as subjects and lights as tokens. Identical schedules of token gain, VR 4 (RI 30 s), operated in both components; a conjoint FR 2 token-loss schedule was superimposed on the token schedule in one component. To separate the direct effects of punishment from indirect effects of changes in the density of food, a yoked-control condition was included, in which the punishment contingency was suspended but the density of food reinforcement was yoked to that from the token-loss condition.
Figure 9 shows summary data, averaged across the final five sessions under baseline (hatched bars) and loss (filled bars) conditions for each pigeon. The filled bars labeled TL (token loss) represent data from punishment conditions, those labeled YF (yoked food) from yoked control conditions, expressed as a proportion of the response rates in the no-loss components of the same sessions. Response rates were somewhat reduced in the YF condition, indicating some food-related decrements, but remained consistently higher than under TL conditions. Because the reinforcer densities were equivalent under the two loss conditions, the greater reductions under token-loss conditions support a punishment interpretation.

Response rates and standard deviations in baseline (hatched bars) and loss (filled bars) conditions, expressed as a percentage of rates in the no-loss component within the same session, for 4 pigeons across the final five sessions of each condition. The labels TL and YF represent data from Token-Loss and Yoked-Food conditions, respectively. See text for additional details. Adapted from Raiff, Bullock, & Hackenberg (2008) .
Although the research on token loss to date is consistent with a punishment interpretation, much remains to be done. For example, little is known about how punishment effects relate to (a) punishment variables (e.g., rate, magnitude, and schedule of token loss), (b) reinforcement variables (e.g., rate, magnitude, and schedule of reinforcement maintaining the punished behavior), (c) motivational variables (e.g., deprivation, generalizability of the tokens), or (d) the availability of unpunished alternatives, to name just a few. The use of token systems may help revitalize the empirical study of aversive control, a field that has been in decline over the past few decades ( Critchfield & Rasmussen, 2007 ).
II. Token Systems and the Symmetrical Law of Effect
One implication of results of token-loss punishment studies is that reinforcers and punishers may be viewed in conceptually analogous terms, as symmetrical changes along a common dimension. This common dimension has long been assumed in major theories of behavior, though empirical support is surprisingly scarce. A major obstacle is methodological: reinforcers are typically appetitive events (e.g., food and water) whereas punishers are typically aversive events (e.g., electric shock). This poses serious scaling issues. For example, how does food compare to shock? Can they be measured in comparable units? One approach to the problem has been to scale reinforcers and punishers empirically, using post hoc descriptive modeling to fit the parameters ( deVilliers, 1980 ; Farley, 1980 ; Farley & Fantino, 1978 ).
An alternative approach is to use token procedures in which gains and losses can be measured in common currency units. This was the strategy adopted by Critchfield, Paletz, MacAleese, and Newland (2003) in a study with human subjects, designed to test two different quantitative models of punishment—a direct suppression model and a competitive suppression model. The former assumes punishment is a primary process and symmetrical effects of losses and gains; the latter assumes punishment is a secondary process and asymmetrical effects of losses and gains. Human subjects were exposed to a variety of concurrent schedules in which choices both occasionally produced and lost points (later exchangeable for money). The schedules of point gain were always unequal: One schedule paid off at a higher rate. The loss schedules were arranged in such a way that the predictions of the two models diverged. In 17 of 20 cases across three experiments when the models made different predictions, the direct suppression model provided a better account of the data. These results are broadly consistent with the few studies conducted with nonhuman animals and electric-shock punishment ( deVilliers, 1980 ; Farley, 1980 ), but the use of point gains and losses obviates the need for post hoc functional scaling of different consequence types.
More recently, Rasmussen and Newland (2008) studied concurrent-schedule performance in humans with schedules of point gain and loss, using the generalized matching law to quantify bias and sensitivity. Under no-punishment conditions, there was slight undermatching and little or no bias. When contingent point loss was superimposed on one of the two schedules, however, sensitivity was sharply reduced, with strong bias toward the unpunished alternative. The bias was more pronounced than would be expected from a consideration of relative reinforcement rates alone, suggesting asymmetrical effects of gains and losses. More specifically, the authors estimated that in this experiment a loss was worth approximately three times more than a gain.
Using a similar analytic strategy with human subjects, Magoon and Critchfield (2008) examined responding under similarly structured concurrent schedules of positive (point gain) and negative (point-loss avoidance) reinforcement. Two matching functions were obtained per subject—one under homogenous conditions (all positive reinforcement) and one under heterogenous conditions (positive and negative reinforcement, arranged concurrently). Because both gains and losses were of similar magnitude, a bias would reflect the differential impact of one consequence type over the other. No such bias was found, indicating symmetrical effects of losses and gains.
Extending these lines of research to token-based procedures with nonhumans would shed light on the cross-species generality of the symmetrical law of effect, putting a sharper quantitative point on the token-loss findings reviewed above. It would also provide a fresh perspective on a range of decision-making phenomena studied extensively in psychology and economics, such as risky choice. To take just one example, when choosing between certain and uncertain outcomes, human subjects are more likely to select the probabilistic (risky) option in a loss context than in a gain context ( Kahnemen & Tversky, 1979 , 2000) . In other words, humans tend to be risk-prone for losses and risk-averse for gains. So pervasive is this finding that it is deemed axiomatic in the most influential theories of human decision-making. Kahneman and Tversky's (1979) Prospect Theory, for example, holds that the subjective value function is convex and steeper for losses than for gains: Losses loom larger—carry greater subjective value—than corresponding gains.
Gain-Loss Symmetry in Comparative Perspective
The gain-loss asymmetry seen in many risky choice studies with humans is part of a larger pattern of biases known as framing effects —the tendency for decisions to be influenced by the context or format in which they are presented ( Kahneman & Tversky, 2000 ). Frames are normally verbal constructions, such as hypothetical scenarios that describe different courses of action. This raises questions about the generality of framing-induced behavioral biases. Are these effects limited to language-proficient humans, or are they more fundamental? To answer this question, recent research has explored framing effects in nonhuman primates.
In a clever experimental adaptation of methods used with human subjects, Chen, Lakshminarayanan, and Santos (2006) tested loss aversion in captive capuchin monkeys. The monkeys were trained to exchange tokens (metal coins) with experimenters for preferred food items. The monkeys then received a budget of 12 tokens that could be spent on either of two options, and were exposed to several conditions designed to assess framing. In one, the monkeys were given choices between two variable options—one framed as a gain and the other as a loss. The gain option began each trial with one food item (displayed by the experimenter) to which a second item was added with 50% probability. The loss option began each trial with two food items from which one was lost with 50% probability. Both options thus provided the same overall return (1.5 food items per trial), but differed in how they were framed. The monkeys generally preferred the gain option—spending more of their token budget on it—a result not explainable on the basis of return rate alone. Instead, the authors argued that the monkeys have an inherent aversion to loss, similar to that commonly observed in humans (but see Silberberg, Roma, Huntsberry, Warren-Boulton, Sakagami, Ruggiero, et al., 2008 , for an alternative interpretation).
In a similar vein, Brosnan, Jones, Lamberth, Mareno, Richardson, and Schapiro (2007) reported suggestive evidence in chimpanzees of an endowment (or status-quo) effect—the seemingly paradoxical tendency to disproportionately weight items already in one's possession. The results of these and other recent studies with primates have important implications for the cross-species generality of behavioral biases, and their presumed evolutionary basis. It has been argued on the basis of these results that behavioral biases are not unique to humans, but rather, are shared by other primates, and in this sense may be a fundamental characteristic of primate cognition. Before this or any other explanation can be properly assessed, however, we need to know a great deal more about behavioral biases in nonhuman animals—the conditions that create them and the range of conditions (and species) under which they are observed. However such research turns out, it promises to reveal important information on the generality of effects deemed foundational to major theories of behavior.
III. Token Systems and Cross-Species Comparisons
A theme running through the studies reviewed in the preceding section is that of cross-species generality—the degree to which principles of behavior are applicable across species. In providing a common methodological context, token procedures are well suited to cross-species comparative studies. One area in which token systems have already proven useful in cross-species comparisons is that of choice and self-control. This section will include a review and discussion of such work.
In a series of important papers, Logue and colleagues (see review by Logue, 1988 ) examined humans' choices between sooner–smaller reinforcers (SSRs) and larger–later reinforcers (LLRs) with token reinforcers (points later exchangeable for money). In a study by Logue, Pena-Correal, Rodriguez, and Kabela (1986) , for example, subjects selected between a small number of points available immediately and a larger number of points available after a delay. Postreinforcer delays followed the SSR, such that overall rate of reinforcement was equal for both options. Under a wide range of conditions, humans generally preferred the LLR, the option providing the greatest net reinforcement.
This basic result has been reported in several other studies of self-control with human subjects and token reinforcers ( Logue, King, Chavarro, & Volpe, 1990 ; Flora & Pavlik, 1992 ). Although such performances have been taken as evidence of self-control, the delays to tokens (points) are only one of several delays in the procedure. Other and perhaps more critical are the delays to the exchange period (the periods during which points are exchangeable for money) and the delays to the terminal reinforcers. In the Logue et al. (1986) study, as in most laboratory studies, the exchange delays were held constant, at the end of a subject's participation in the experiment. If these later reinforcers in the chain—the delays to exchange and money—are viewed as the operative reinforcers, then the self-control seen in most laboratory studies with human subjects may instead be viewed as sensitivity to monetary reinforcer amount with monetary reinforcer delay held constant. Such sensitivity to reinforcer amount is an interesting and important finding in its own right, as there are few such data on reinforcer magnitude effects in humans. By itself, however, the finding may hold little relevance to the issue of self-control. To constitute self-control, a subject must face contrasting delays to the terminal reinforcers.
Reconceptualizing the traditional procedures as a token-reinforcement system suggests that self-control observed in prior results may be limited to those cases in which self-control and impulsivity are defined with respect to token delays and the exchange delays are held constant. In support of this, Hyten, Madden, and Field (1994) found evidence of an exchange-delay effect with humans in a self-control procedure: Subjects were more likely to exhibit self-control (i.e., select the larger number of points each trial) when the exchange delays were equal (e.g., a week after the session) than when they were unequal and shorter for the small-reinforcer option (see also Roll, Reilly, & Johanson, 2000 , for analogous effects in choices between money and cigarettes).
Jackson and Hackenberg (1996) reported similar findings in an analogous study with pigeons and token reinforcers. The tokens were stimulus lights, as described above, designed to mimic the display of points in analogous experiments with human subjects. Like points, the light tokens served as currency; each token was worth 2-s food, in that it could be exchanged for that amount of food during scheduled exchange periods. In the basic procedure, pigeons were given repeated choices between SSR (1 token delivered immediately) and LLR (3 tokens delivered after a delay), with the overall rate of (token and food) reinforcement held constant. In some conditions (Experiment 4), the delays to the exchange periods (when any earned tokens could be exchanged for food) were equal irrespective of which choice had been made. These equal-exchange delay (ED) conditions were designed to mimic the typical human experiment in which the delays to the exchange period and money are held constant. In other conditions, the exchange periods were scheduled just after token delivery, and were therefore unequal and shorter for the SSR. These unequal-exchange delay conditions (UD) are more like the conditions under which self-control is typically studied with nonhuman subjects. If choices are governed not by the token delays, but by the exchange delays and food, then one would predict preference for the LLR under ED conditions and the SSR under UD conditions.
Summary results are shown in Figure 10 , which displays the number of choices (in a 10-trial session) of the LLR across exchange-delay conditions: UD for unequal exchange delay and ED for equal exchange delay. All 3 pigeons strongly preferred the small-reinforcer option under UD conditions and the large-reinforcer option under ED conditions. Thus, consistent with the Hyten et al. (1994) results with humans, pigeons' choices were governed not by token delays but by the delays to periods during which those tokens are exchanged for other reinforcers.

Mean number of large-reinforcer choices per 10-trial session for 3 pigeons in a token-based self-control procedure as a function of exchange-delay conditions. Delays to the exchange periods were either unequal and shorter for the small-reinforcer option (UD) or equal for both options (ED). Data are from the final 10 sessions per condition; error bars reflect the range of values contributing to the mean. From Jackson & Hackenberg (1996). Copyright 1996, by the Society for the Experimental Analysis of Behavior, Inc. Reprinted with permission.
Hackenberg and Vaidya (2003) replicated and extended these results, separately manipulating not only the exchange delays but the terminal-reinforcer (food) delays as well. Together, the results of these studies demonstrate disproportionate control by reinforcers later in the chain (exchange and terminal-reinforcer delays) relative to token reinforcers. That delays to exchange and terminal reinforcers exert strong control over behavior is not surprising given the exchange-schedule effects reviewed above, and the extensive literature on extended-chained ( Gollub, 1977 ) and concurrent-chained schedules ( Fantino, 1977 ). Indeed, such effects follow directly from a conceptualization of a token schedule as a kind of hybrid schedule with extended and concurrent-chained components. In support of this, in conditions in the Jackson and Hackenberg (1996) study in which exchange periods followed blocks of choice trials (Experiments 1 and 2), and in which tokens therefore accumulated prior to exchange, choice latencies were long in the early trials of a block, and short and undifferentiated in the later trials of a block.
Taken as a whole, the results with humans ( Hyten et al., 1994 ) and pigeons ( Jackson & Hackenberg, 1996 ; Hackenberg & Vaidya, 2003 ) converge on a similar point: In token-based self-control procedures, choice patterns are governed not by token delays but by delays to the periods during which tokens are exchangeable for other reinforcers. When these delays are equal, preferences for the LLR usually prevail; when the delays are unequal, preferences for the SSR usually prevail. In other words, under conditions more typical of research with humans, more “human-like” performance is seen (i.e., self-control), whereas under conditions more typical of research with nonhuman animals, more “animal-like” performance is seen (i.e., impulsivity). This is not to imply that all differences between human and nonhuman self-control are due to procedural variables. Other factors, such as human verbal and rule-governed histories, may interact with procedural variables. It is not possible to isolate the impact of these or any other factors, however, unless procedural differences are taken into proper account. A failure to do so may result in misleading or premature conclusions in cross-species comparisons ( Hackenberg, 2005 ).
IV. Token Systems and Behavioral Economics
Token reinforcement procedures are highly relevant to behavioral economics. From an economic perspective, tokens can be conceptualized as a type of currency, earned and exchanged for other commodities. Token production is analogous to a wage, exchange opportunities to procurement costs, accumulation to savings, terminal reinforcers to expenditures, and so on. To date, however, there has been little substantive contact between behavioral economics and token reinforcement. This is unfortunate, because token systems provide a controlled and analytically tractable environment in which to explore interactions between behavior and economic variables.
In this section, the role of token systems in behavioral economics will be discussed. First, relations between tokens and consumer-demand concepts will be explored, showing how token systems permit more refined analyses of price than traditional formulations. Second, the utility of establishing common currency in assessing demand for qualitatively different commodities (including generalized commodities) will be discussed. Third, some applied implications of token economic systems will be considered. Finally, the role of token systems in forging a cross-disciplinary approach to behavioral economics will be discussed.
Demand Elasticity and Unit Price
The Bullock and Hackenberg (2006) study, reviewed above, showed that response rates declined systematically with increases in token-production ratio and exchange-production ratio (see Figure 4 ). These results also follow from an economic analysis: Response output depended both on the costs of producing a token (wages) and the costs of producing exchange opportunities (procurement costs). In everyday terms, one might think of these as the costs associated with earning money (e.g., on a job) and the costs associated with spending that money (e.g., driving to the store, making an internet purchase). That responding decreased across both sets of variables suggests that costs can be meaningfully segregated into token production (wages) and exchange production (procurement).
A third way to vary price in token schedules is illustrated by the Malagodi et al. (1975) study, reviewed above. Rather than altering the price via number of responses required to produce a token (wage), or number of tokens required to produce an exchange (procurement), Malagodi et al. manipulated the number of tokens required to produce each food delivery—the exchange price. Outside the lab, these costs are analogous to the selling price of a commodity—how much currency is spent on each unit of the commodity. As would be expected from an economic perspective, response rates in the initial links were a decreasing function of exchange rate (number of tokens required per unit of food).
The declining response rates with increases in price (more stringent token-production, exchange-production, and token-exchange requirements) resemble the downward-sloping demand curves of behavioral economic analysis ( Hursh & Silberberg, 2008 ), suggesting that demand is sensitive to all three types of price. It is also possible on these procedures to compute price differently, as a unit price , a composite cost–benefit measure based on the combined effects of the reinforcer size and price ( DeGrandpre, Bickel, Hughes, Layng, & Badger, 1993 ). In laboratory experiments, unit price is typically defined as the number of responses per unit of reinforcer. Token schedules are especially well suited to a unit-price analysis because the number of tokens is correlated not only with proximity to the terminal reinforcer but with the magnitude of that reinforcer. With ratio-based token schedules, the unit price equals the cumulative number of responses required to produce a food reinforcer divided by the number of food reinforcers.
In the Bullock and Hackenberg (2006) study, for example, a token-production ratio of 25 and an exchange-production ratio of 4 yields a unit price of 25 (100 responses for four reinforcers). Holding constant the exchange-production ratio at 4, but increasing the token-production ratio to 100 yields a four-fold increase in the unit price (400 responses per four reinforcers). Consistent with these increases in unit price, response rates decreased with increases in the size of the token-production ratio (left side of Figure 4 ). Conversely, holding the token-production schedule constant at FR 25, but changing the exchange-production schedule from 2 to 8 yields equivalent unit prices—50 responses for two reinforcers (unit price of 25) vs. 400 responses for eight reinforcers (unit price of 25). Based on the equivalent unit prices, one might expect equivalent response rates across variations in exchange-production schedules. Contrary to this prediction, however, response rates decreased with increases in the exchange-production ratio, despite equivalent unit prices (right side of Figure 4 ). Responding appeared to be governed not by the unit price (number of responses per unit of reinforcer), but rather by the number of responses (or delay) per exchange period, without regard to the number of reinforcers available per exchange.
To further explore unit price in a token reinforcement context, Foster and Hackenberg (2004) gave pigeons repeated choices between FR token-reinforcement schedules with equal and unequal unit prices. Consistent with a straightforward application of the unit price model, when unit prices were unequal, pigeons strongly preferred the alternative with the lower unit price. When unit prices were equal, however, pigeons preferred alternatives with smaller FRs and less food to those with larger FRs and more food. Although inconsistent with the standard unit price equation, the data were broadly consistent with a modified version incorporating temporal discounting ( Madden, Bickel, & Jacobs, 2000 ). And if viewed in relation to delays rather than responses, the results are also consistent with delay-reduction theory ( Fantino, 1977 ). Like the data with single alternatives, time and/or responses to the exchange period appear to be critical determinants of choice behavior.
In a similar vein, the results of the Yankelevitz et al. (2008) study, reviewed above, are also consistent with a unit-price formulation. In that study, different patterns of accumulation yielded different unit prices. Because the exchange-production ratio had only to be completed once to exchange all of the tokens that cycle, accumulating the maximum of 12 tokens was the optimal pattern, the pattern that always yielded the lowest unit price in the long run. Conversely, accumulating no tokens—moving to exchange at the first opportunity—yielded the highest overall unit price. Intermediate levels of accumulation yielded intermediate unit prices. To the extent that the token-production and exchange-production ratios were concurrently available, token accumulation can be conceptualized as a series of choices between accumulating one or more additional tokens versus exchanging tokens already earned that cycle—a tradeoff between different unit prices, computed across different time periods. In the Yankelevitz et al. study, accumulation varied directly with the exchange-production ratio, with a modified unit-price model providing a reasonably accurate description of the data.
In sum, these results illustrate how the economic concept of unit price may be brought to bear on token-reinforcement procedures. Unlike traditional conceptions of unit price in which costs are defined solely in terms of response output, however, token systems permit a more refined analysis of cost—the costs of producing tokens (wages), the costs of producing exchange opportunities (procurement), and the costs of exchanging tokens for other commodities (selling price). This may be useful, depending on the particular context in which price is assessed, as there is clear evidence that token-reinforced behavior is multiply determined by the token-production schedule, the exchange-production schedule, and the token-exchange schedule. An economic analysis needs to consider how such schedule effects enter into broader cost–benefit formulations.
Common Currency Units
Another major benefit of token systems is the possibility of developing a common currency with which to assess with greater quantitative precision the relationships between qualitatively different commodities. This is a central aim of behavioral economics, but to this point the currency has been defined almost exclusively in relation to response or time allocation; in other words, price of a reinforcer is paid in the currency of responses or time. Simple response allocation and time allocation measures can sometimes be problematic, however, especially with comparisons between low-frequency activities of high value and high-frequency activities of low value. This is in part what motivated research on concurrent-chains schedules ( Autor, 1969 ; Fantino, 1977 ), in which preference for a reinforcer (behavior in the initial link) can be separated from behavior in the presence of signals paired with that reinforcer (behavior in the terminal link). In this way, preference is measured in the same currency units (relative initial-link responses).
Token systems provide an alternate approach to the problem of common currency. Rather than defining currency in responses or time, the currency is defined in common token units, and preference as the number of tokens a subject is willing to spend on an activity or commodity. Tokens are a kind of commodity economists term fungible , or mutually replaceable for another of like kind. To the extent that tokens are interchangeable, one for another, they can be used to scale qualitatively different commodities.
Such scaling procedures would be especially useful in assessing demand for generalized commodities. Generalized commodities are pervasive in everyday human economic contexts, money being the prime example. Money is such powerful currency because it is associated with a wide variety of other goods and services—its functions are generalized rather than specific to a particular currency and economic context. In principle, such generalized commodities should be more durable than specific reinforcers; or, in economic terms, less sensitive to price changes (less elastic). One might also expect generalized commodities to hold their value across a wider range of motivational conditions; or, in economic terms, to function as substitutes for less generalized (specific) commodities. Although both of these assertions are plausible, there is little empirical evidence to support them. Indeed, convincing demonstrations of generalized reinforcement under laboratory conditions are virtually nonexistent. Most attempts have either failed ( Myers & Trapold, 1966 ) or produced weak and transient effects ( Lubinski & Thompson, 1987 ).
Token systems are well suited to address generalized reinforcement functions. Establishing and evaluating the efficacy of tokens as generalized reinforcers would provide an important bridge to monetary-based economies in human affairs, a crucial step in the extrapolation of laboratory-based experimental economics to that of everyday human economic behavior. This would help bring within methodological reach a host of scientific problems heretofore limited to research with humans. Economic issues aside, establishing and maintaining generalized reinforcement under laboratory conditions would itself be a groundbreaking development in the experimental analysis of behavior, opening new research vistas.
Applied Implications
A better understanding of token systems also has important practical implications. Token economies are among the oldest and most successful programs in all of applied psychology. Despite their well documented therapeutic and educational benefits ( Glynn, 1990 ; Kazdin, 1982 ; Williams, Williams, & McLaughlin, 1989 ), research on token economies in the applied realm has developed with little or no recognition of laboratory research on token systems, nor has it benefited from an economic perspective. Laboratory research on token systems and experimental economics suggest a myriad of techniques for maximizing the efficacy of token systems as motivational and behavior-management tools in applied settings, including ways to establish new behavior, to maintain behavior under long delays to reinforcement, to encourage savings and sensitivity to long-term outcomes, to name just a few. In short, laboratory research on token systems, especially research that takes an explicitly economic focus, has important but underappreciated implications for clinical and educational programs.
At the same time, reconceptualizing applied token programs in behavioral-economic terms—as applied laboratories for investigating economic behavior—has the potential to significantly advance research and theory in behavioral economics. In an interesting series of papers in the 1970s, Winkler and colleagues encouraged a view of token systems as experimental economies, arguing that token systems were ripe for an economic analysis. Using this type of analysis, several studies of token systems in state psychiatric hospitals found broad support for economic demand theory ( Battalio, Kagel, Winkler, Fisher, Bassman, & Krasner, 1974 ; Fisher, Winkler, Krasner, Kagel, Battalio, & Basmann, 1978 ; Winkler, 1970 , 1973 , 1980 ), suggesting the general utility of an economic perspective on token systems.
This applied behavioral-economic work failed to gain a foothold at the time, but there are reasons to suspect a more supportive intellectual climate today. A number of applied researchers have successfully applied behavioral-economic concepts and analyses to an ever-widening range of topics, including reinforcer assessment ( DeLeon, Iwata, Goh, & Worsdell, 1997 ), substitution effects ( Hanley, Iwata, Lindberg, & Connors, 2003 ), and remediation of problem behavior ( Roane, Call, & Falcomata, 2005 ; Tustin, 1994 ), to name just a few. Combining this emerging economic perspective with token economies in applied research has the potential to transform applied settings (classrooms, group homes, work centers, and the like) into laboratories for examining economic behavior.
The field of substance abuse liability and treatment has drawn extensively from behavioral-economic analyses ( Bickel, DeGrandpre, & Higgins, 1995 ; Hursh, 1991 ), especially the subfield utilizing contingency management, or voucher reinforcement ( Silverman, 2004 ). Vouchers are tokens exchangeable for money or goods, made contingent on drug abstinence. This form of treatment has been shown to be a highly effective approach to drug abstinence with various drugs (see Higgins, Heil, & Lussier, 2004 , for a review), although a relatively narrow range of contingencies has been explored to date. In the typical program, an escalating magnitude of reinforcement schedule is used, whereby successive urine-free samples earn increasing monetary amounts. The schedule is often coupled with a punishment schedule, whereby a positive sample resets the earnings to a lower value. Although this has proven effective in a majority of drug-dependent research participants, little is known about these kinds of schedules and the degree to which they are optimal for producing abstinence. Combining this line of research with a laboratory-based analysis of token reinforcement contingencies may further enhance the clinical efficacy of these already successful programs. At the same time, this would expand the study of token schedules, bringing an interesting new range of contingencies within the scope of an experimental analysis.
Laboratory studies of drug self-administration may also benefit from the use of token schedules. In a typical self-administration experiment, subjects (usually nonhuman primates) are trained to respond for access to drug infusions. Using both simple and concurrent schedules, such procedures have proven useful in assessing the relative reinforcing efficacy of a variety of drugs. A potential difficulty with such procedures, however, is that the pharmacological effects of a drug may interact with its reinforcing efficacy. For example, large doses of drugs produce pharmacological and motoric effects that interfere with behavior maintained by access to the drug. Second-order schedules of brief stimulus presentation have been used to help circumvent this problem by providing a context in which drug-paired stimuli maintain extended sequences of behavior in the absence of drug ( Kelleher & Goldberg, 1977 ). Expanding these methods to second-order schedules of token reinforcement would combine an economic perspective with the considerable methodological advantages of second-order schedules. For example, one can envision a token system in which tokens earned in an extended work component could be used to purchase drug infusions at various prices, either in isolation or in combination with other drug and nondrug reinforcers. Such work would considerably advance the study of drugs as reinforcers, permitting an analysis of demand in relation to other reinforcers in a context that approximates human economic systems.
Toward a Cross-Disciplinary Behavioral Economics
Behavioral economics is not a single unified theory, but rather, a family of models and interpretations sharing some common assumptions. There are two main branches of behavioral economics, arising from somewhat different traditions of research and interpretation. One comes mainly from consumer demand theory—how the value of a commodity is influenced by economic variables (e.g., price, income, availability of substitutable goods). This is the main behavioral-economic approach familiar to readers of this journal, and the one emphasized in the preceding sections.
The other behavioral-economic approach arises from more cognitively-oriented studies of human judgment and decision-making, emphasizing anomalies or biases in judgment ( Camerer, Loewenstein, & Rabin, 2003 ). Spawned by Kahneman and Tversky's highly influential research on context and framing effects ( Kahneman & Tversky, 1979 , 2000 ; Tversky & Kahneman, 1974 , 1992 ), other lines of investigation have revealed systematic departures from classical economic theory and its implicit assumption of utility maximization, including studies of behavioral finance ( Thaler, 1993 ), time discounting ( Frederick, Loewenstein, & O'Donoghue, 2002 ), behavioral game theory ( Camerer, 2003 ), risk assessment ( Fischhoff, 1995 ), and the expanding field of neuroeconomics ( Camerer, Loewenstein, & Prelec, 2005 ; McClure, Laibson, Loewenstein, & Cohen, 2004 ), to name just a few.
Although both branches of behavioral economics are concerned broadly with behavior in an economic context—and both adopt the term behavioral economics —they have developed largely in parallel, with little cross-fertilization of ideas and concepts. This is unfortunate, because these two traditions of research and interpretation yield complementary perspectives, and both are necessary parts of a comprehensive behavioral-economic conception of the subject matter. A major obstacle is the lack of a standard methodology. Behavioral economics from the consumer-demand tradition has typically used nonhuman animals as subjects and commodities with immediate consummatory value (e.g., food, water, drug). Behavioral economics from the judgment and decision making realm, on the other hand, has typically used humans as subjects and monetary-based commodities (or hypothetical scenarios with monetary-based outcomes). Until these different traditions are brought into greater methodological alignment, the possibilities for a broader, more integrative approach, are remote.
Token systems provide a methodological and analytic context in which behavioral economics of both persuasions can develop in more coherent and mutually supportive ways. A token system combines the best features of each approach. Like the approach emanating from the consumer-demand realm, laboratory-based token systems maximize experimental control, permitting parametric manipulation of variables useful in an economic analysis. Like the approach growing out of the judgment and decision-making literature, token systems are modeled after monetary-based economic systems, in which tokens are earned, accumulated, and exchanged for other commodities. And when combined with generalized tokens, exchangeable for multiple commodities, laboratory-based token systems bring within reach an exciting range of possibilities for an experimental analysis of economic behavior.
V. Summary and Conclusions
Token systems are ubiquitous in human culture, providing the basic framework for economic transactions with the world. Yet despite laboratory research dating back to the 1930s and the highly successful educational and therapeutic applications of token procedures since the 1960s, relatively little is known about token reinforcement per se—about its basic principles of operation. Two chief aims of the present paper were to review what is known about token reinforcement under laboratory conditions, and to highlight promising research directions.
Research reviewed in Part I explored the various behavioral functions of tokens, including the conditions that give rise to and maintain the reinforcing functions of tokens, the generalizability of token reinforcers, the role of tokens in temporally organized behavior, the antecedent (discriminative and signaling) functions of tokens, and the conditioned aversive functions of token loss. When viewed as an interrelated set of contingencies, token schedules share important properties with other sequentially-arranged contingencies (e.g., chained and second-order schedules). Like these other schedules, token schedules can be used both to establish and maintain behavior over extended time periods, and to create and synthesize behavioral units that participate in larger functional units. And, like these other schedules, the added stimuli in token-reinforcement schedules can serve multiple functions—reinforcing, discriminative, and eliciting—depending on the particular temporal-correlative relations with other events. Token schedules, in particular among sequence schedules, fall squarely in the middle of important but unresolved issues in the field of conditioned reinforcement.
In addition to the sequential arrangement of contingencies they share with other schedules, token schedules possess unique characteristics that make them useful as tools in investigating a wide range of other scientific problems. And it is in these extensions to new areas of research and theory where the potential impact of token reinforcement looms largest. This was the focus of research reviewed in the later sections of the paper.
Section II explored the use of token schedules in addressing the symmetrical law of effect, the view that gains and losses exert equivalent but opposite effects on behavior. This issue is of fundamental importance in the analysis of behavior, but past efforts have been hampered by qualitative differences in the nature of the reinforcer (gain) and punisher (loss) stimuli. With token schedules, it is possible to define gains and losses in conceptually analogous terms, as changes in sign along a common dimension of value. Research (mostly with human subjects) has begun to reveal the relationships between gains and losses, but this work is still quite preliminary. Using token procedures to explore these questions with nonhuman subjects would provide important information on the cross-species generality of the phenomena, bringing new investigative realms within the scope of a laboratory analysis.
Research reviewed in Section III considered the use of token schedules in cross-species analysis of behavior more generally. Token schedules are well suited to such comparative analyses, as they mimic common procedural features of laboratory research with humans, and thus provide a common methodological context in which to compare human performance to that of other animals. Token schedules have been used to minimize procedural disparities across species, bringing human and nonhuman performances into better accord. While much remains to be done in this realm, the use of token schedules holds great promise as a methodological tool in comparative analyses.
The research reviewed in Section IV illustrated some of the ways in which token systems inform and extend an economic analysis of behavior. Enabling detailed measurement of economic behavior (e.g., labor, consumption, preference, savings) on multiple levels of analysis and in relation to a wide range of economic variables (e.g., prices, wages, interest, inflation), token systems provide an experimental test bed for fundamental concepts in behavioral economics. At the same time, economic methods and concepts (demand curves, unit price, fungible currency) may yield insights into the complex interdependencies between various components of a token system, putting a sharper quantitative point on functional relationships between behavior and reinforcement variables.
The relationship between analysis and extension goes both ways. As more is learned about its basic principles of operation, the more effective token systems become as methodological tools, and the more likely they are to yield conceptual advances. In this way, the extensions of token reinforcement to new domains of research and theory may begin to repay the methodological debt in conceptual breakthroughs including, perhaps, even new ways to approach the old problem of conditioned reinforcement with which this research began.
Acknowledgments
This paper is dedicated to the memory of E. F. Malagodi for his enduring contributions to the study of token reinforcement. Manuscript preparation was supported by NSF Grant IBN 0420747. I am indebted to Edmund Fantino and an anonymous reviewer for comments on an earlier version of the paper, and to Rachelle Yankelevitz and Bethany Raiff for assistance with figure preparation.
- Autor S.M. The strength of conditioned reinforcers as a function of frequency and probability of reinforcement. In: Hendry D.P, editor. Conditioned reinforcement. Homewood, IL: Dorsey Press; 1969. pp. 127–162. [ Google Scholar ]
- Ayllon T, Azrin N.H. The token economy: A motivational system for therapy and rehabilitation. New York: Appleton-Century-Crofts; 1968. [ Google Scholar ]
- Battalio R.C, Kagel J.H, Winkler R.C, Fisher E.B, Jr, Bassman R.L, Krasner L. An experimental investigation of consumer behavior in a controlled environment. Journal of Consumer Research. 1974; 1 :52–60. [ Google Scholar ]
- Bickel W.K, DeGrandpre R.J, Higgins S.T. The behavioral economics of concurrent drug reinforcers: A review and reanalysis of drug self-administration research. Psychopharmacology. 1995; 118 :250–259. [ PubMed ] [ Google Scholar ]
- Boakes R.A, Poli M, Lockwood M.J, Goodall G. A study of misbehavior: Token reinforcement in the rat. Journal of the Experimental Analysis of Behavior. 1978; 29 :115–134. [ PMC free article ] [ PubMed ] [ Google Scholar ]
- Brethower D.M, Reynolds G.S. A facilitative effect of punishment on unpunished behavior. Journal of the Experimental Analysis of Behavior. 1962; 5 :191–199. [ PMC free article ] [ PubMed ] [ Google Scholar ]
- Brosnan S.F, Jones O.D, Lamberth S.P, Mareno C, Richardson A.S, Schapiro S.J. Endowment effect in chimpanzees. Current Biology. 2007; 17 :1704–1707. [ PubMed ] [ Google Scholar ]
- Bullock C.E, Hackenberg T.D. Second-order schedules of token reinforcement with pigeons: Implications for unit price. Journal of the Experimental Analysis of Behavior. 2006; 85 :95–106. [ PMC free article ] [ PubMed ] [ Google Scholar ]
- Camerer C.F. Behavioral game theory: Experiments in strategic interaction. Princeton, NJ: Princeton University Press; 2003. [ Google Scholar ]
- Camerer C.F, Loewenstein G.F, Prelec D. Neuroeconomics: How neuroscience can inform economics. Journal of Economic Literature. 2005; 43 :9–64. [ Google Scholar ]
- Camerer C.F, Loewenstein G, Rabin M, editors. Advances in behavioral economics. Princeton, NJ: Princeton University Press; 2003. [ Google Scholar ]
- Chen M.K, Lakshminarayanan V, Santos L.R. How basic are behavioral biases? Evidence from Capuchin monkey trading behavior. Journal of Political Economy. 2006; 114 :517–537. [ Google Scholar ]
- Cole M.R. Operant hoarding: A new paradigm for the study of self-control. Journal of the Experimental Analysis of Behavior. 1990; 53 :247–261. [ PMC free article ] [ PubMed ] [ Google Scholar ]
- Cowles J.T. Food-tokens as incentives for learning by chimpanzees. Comparative Psychological Monographs. 1937; 12 :1–96. [ Google Scholar ]
- Critchfield T.S, Paletz E.M, Macaleese K.R, Newland M.C. Punishment in human choice: Direct or competitive suppression. Journal of the Experimental Analysis of Behavior. 2003; 80 :1–27. [ PMC free article ] [ PubMed ] [ Google Scholar ]
- Critchfield T.S, Rasmussen E.R. It's aversive to have an incomplete science of behavior. Mexican Journal of Behavior Analysis. 2007; 33 :1–6. [ Google Scholar ]
- Crosbie J, Williams A.M, Lattal K.A, Anderson M.M, Brown S.M. Schedule interactions involving punishment with pigeons and humans. Journal of the Experimental Analysis of Behavior. 1997; 68 :161–175. [ PMC free article ] [ PubMed ] [ Google Scholar ]
- DeGrandpre R.J, Bickel W.K, Hughes J.R, Layng M.P, Badger G. Unit price as a useful metric in analyzing effects of reinforcer magnitude. Journal of the Experimental Analysis of Behavior. 1993; 60 :641–666. [ PMC free article ] [ PubMed ] [ Google Scholar ]
- DeLeon I.G, Iwata B.A, Goh H, Worsdell A.S. Emergence of reinforcer preference as a function of schedule requirements and stimulus similarity. Journal of Applied Behavior Analysis. 1997; 30 :439–449. [ PMC free article ] [ PubMed ] [ Google Scholar ]
- de Villiers P.A. Toward a quantitative theory of punishment. Journal of the Experimental Analysis of Behavior. 1980; 33 :15–25. [ PMC free article ] [ PubMed ] [ Google Scholar ]
- Dewsbury D.A. Conflicting approaches: Operant psychology arrives at a primate laboratory. The Behavior Analyst. 2003; 26 :253–265. [ PMC free article ] [ PubMed ] [ Google Scholar ]
- Dewsbury D.A. Monkey Farm: A history of the Yerkes Laboratories of Primate Biology, Orange Park, Florida, 1930–1965. Lewisburg, PA: Bucknell University Press; 2006. [ Google Scholar ]
- Fantino E. Conditioned reinforcement: Choice and information. In: Honig W.K, Staddon J.E.R, editors. Handbook of operant behavior. Englewood Cliffs, NJ: Prentice-Hall; 1977. pp. 313–339. [ Google Scholar ]
- Farley J. Reinforcement and punishment effects in concurrent schedules: A test of two models. Journal of the Experimental Analysis of Behavior. 1980; 33 :311–326. [ PMC free article ] [ PubMed ] [ Google Scholar ]
- Farley J, Fantino E. The symmetrical law of effect and the matching relation in choice behavior. Journal of the Experimental Analysis of Behavior. 1978; 29 :37–60. [ PMC free article ] [ PubMed ] [ Google Scholar ]
- Ferster C.B, Skinner B.F. Schedules of reinforcement. New York: Appleton-Century-Crofts; 1957. [ Google Scholar ]
- Fischhoff B. Ranking risks. Risk: Health, Safety and Environment. 1995; 6 :189–200. [ Google Scholar ]
- Fisher E.B, Jr, Winkler R.C, Krasner L, Kagel J, Battalio R.C, Basmann R.L. Economic perspectives in behavior therapy: Complex interdependencies in token economies. Behavior Therapy. 1978; 9 :391–403. [ Google Scholar ]
- Flora S.R, Pavlik W.B. Human self-control and the density of reinforcement. Journal of the Experimental Analysis of Behavior. 1992; 57 :201–208. [ PMC free article ] [ PubMed ] [ Google Scholar ]
- Foster T.A, Hackenberg T.D. Choice and unit price in a token reinforcement context. Journal of the Experimental Analysis of Behavior. 2004; 81 :5–25. [ PMC free article ] [ PubMed ] [ Google Scholar ]
- Foster T.A, Hackenberg T.D, Vaidya M. Second-order schedules of token reinforcement with pigeons: Effects of fixed- and variable-ratio exchange schedules. Journal of the Experimental Analysis of Behavior. 2001; 76 :159–178. [ PMC free article ] [ PubMed ] [ Google Scholar ]
- Frederick S, Loewenstein G, O'Donoghue T. Time discounting and time preference: A critical review. Journal of Economic Literature. 2002; 40 :351–401. [ Google Scholar ]
- Glynn S.M. Token economy approaches for psychiatric patients: progress and pitfalls over 25 years. Behavior Modification. 1990; 14 :383–407. [ PubMed ] [ Google Scholar ]
- Gollub L. Conditioned reinforcement: Schedule effects. In: Honig W.K, Staddon J.E.R, editors. Handbook of operant behavior. Englewood Cliffs, NJ: Prentice-Hall; 1977. pp. 288–312. [ Google Scholar ]
- Green L, Rachlin H. Economic and biological influences on a pigeon's key peck. Journal of the Experimental Analysis of Behavior. 1975; 23 :55–62. [ PMC free article ] [ PubMed ] [ Google Scholar ]
- Hackenberg T.D. Of pigeons and people: Some observations on species differences in choice and self-control. Brazilian Journal of Behavior Analysis. 2005; 1 :135–147. [ Google Scholar ]
- Hackenberg T.D, Vaidya M. Second-order schedules of token reinforcement with pigeons: Effects of fixed and variable-ratio exchange schedules. Journal of the Experimental Analysis of Behavior. 2001; 76 :159–178. [ PMC free article ] [ PubMed ] [ Google Scholar ]
- Hackenberg T.D, Vaidya M. Determinants of pigeons' choices in token-based self-control procedures. Journal of the Experimental Analysis of Behavior. 2003; 79 :207–218. [ PMC free article ] [ PubMed ] [ Google Scholar ]
- Hanley G.P, Iwata B.A, Lindberg J.S, Conners J. Response-restriction analysis: I. Assessment of activity preferences. Journal of Applied Behavior Analysis. 2003; 36 :47–58. [ PMC free article ] [ PubMed ] [ Google Scholar ]
- Hanson H.M, Witoslawski J.J. Interaction between the components of a chained schedule. Journal of the Experimental Analysis of Behavior. 1959; 2 :171–177. [ PMC free article ] [ PubMed ] [ Google Scholar ]
- Higgins S.T, Heil S.H, Lussier J.P. Clinical implications of reinforcement as a determinant of substance abuse disorders. Annual Review of Psychology. 2004; 55 :431–461. [ PubMed ] [ Google Scholar ]
- Horney J, Fantino E. Choice for conditioned reinforcers in the signaled absence of primary reinforcement. Journal of the Experimental Analysis of Behavior. 1984; 41 :193–201. [ PMC free article ] [ PubMed ] [ Google Scholar ]
- Hursh S.R. Economic concepts for the analysis of behavior. Journal of the Experimental Analysis of Behavior. 1980; 34 :219–238. [ PMC free article ] [ PubMed ] [ Google Scholar ]
- Hursh S.R. Behavioral economics of drug self-administration and drug abuse policy. Journal of the Experimental Analysis of Behavior. 1991; 56 :377–393. [ PMC free article ] [ PubMed ] [ Google Scholar ]
- Hursh S.R, Silberberg A. Economic demand and essential value. Psychological Review. 2008; 115 :186–198. [ PubMed ] [ Google Scholar ]
- Hyten C, Madden G.J, Field D.P. Exchange delays and impulsive choice in adult humans. Journal of the Experimental Analysis of Behavior. 1994; 62 :225–233. [ PMC free article ] [ PubMed ] [ Google Scholar ]
- Jackson K, Hackenberg T.D. Token reinforcement, choice, and self-control in pigeons. Journal of the Experimental Analysis of Behavior. 1996; 66 :29–49. [ PMC free article ] [ PubMed ] [ Google Scholar ]
- Jwaideh A.R. Responding under chained and tandem fixed-ratio schedules. Journal of the Experimental Analysis of Behavior. 1973; 19 :259–267. [ PMC free article ] [ PubMed ] [ Google Scholar ]
- Kagel J.H. Token economies and experimental economics. Journal of Political Economy. 1972; 80 :779–785. [ Google Scholar ]
- Kagel J.H, Winkler R.C. Behavioral economics: Areas of cooperative research between economics and applied behavioral analysis. Journal of Applied Behavior Analysis. 1972; 5 :335–342. [ PMC free article ] [ PubMed ] [ Google Scholar ]
- Kahneman D, Tversky A. Prospect theory: An analysis of decisions under risk. Econometrica. 1979; 47 :263–291. [ Google Scholar ]
- Kahneman D, Tversky A, editors. Choices, values, and frames. New York: Cambridge University Press; 2000. [ Google Scholar ]
- Kazdin A.E. The token economy: A review and evaluation. New York: Plenum Press; 1977. [ Google Scholar ]
- Kazdin A.E. History of behavior modification: Experimental foundations of contemporary research. Baltimore, MD: University Park Press; 1978. [ Google Scholar ]
- Kazdin A.E. The token economy: A decade later. Journal of Applied Behavior Analysis. 1982; 15 :431–445. [ PMC free article ] [ PubMed ] [ Google Scholar ]
- Kelleher R.T. Intermittent conditioned reinforcement in chimpanzees. Science. 1956; 124 :679–680. [ PubMed ] [ Google Scholar ]
- Kelleher R.T. Fixed-ratio schedules of conditioned reinforcement with chimpanzees. Journal of the Experimental Analysis of Behavior. 1958; 1 :281–289. [ PMC free article ] [ PubMed ] [ Google Scholar ]
- Kelleher R.T. Chaining and conditioned reinforcement. In: Honig W.K, editor. Operant behavior: Areas of research and application. New York: Appleton-Century-Crofts; 1966a. pp. 160–212. [ Google Scholar ]
- Kelleher R.T. Conditioned reinforcement in second-order schedules. Journal of the Experimental Analysis of Behavior. 1966b; 9 :475–485. [ PMC free article ] [ PubMed ] [ Google Scholar ]
- Kelleher R.T, Goldberg S.R. Fixed-interval responding under second-order schedules of food presentation or cocaine injection. Journal of the Experimental Analysis of Behavior. 1977; 28 :221–231. [ PMC free article ] [ PubMed ] [ Google Scholar ]
- Kelleher R.T, Gollub L.R. A review of positive conditioned reinforcement. Journal of the Experimental Analysis of Behavior. 1962; 5 :543–597. [ PMC free article ] [ PubMed ] [ Google Scholar ]
- Killeen P. Psychophysical distance functions for hooded rats. The Psychological Record. 1974; 24 :229–235. [ Google Scholar ]
- Lea S.E.G. The psychology and economics of demand. Psychological Bulletin. 1978; 85 :441–466. [ Google Scholar ]
- Lieberman D.A, Davidson F.H, Thomas G.V. Marking in pigeons: The role of memory in delayed reinforcement. Journal of Experimental Psychology: Animal Behavior Processes. 1985; 11 :611–624. [ Google Scholar ]
- Logue A.W. Research on self-control: An integrating framework. Behavioral and Brain Sciences. 1988; 11 :665–709 (includes commentary). [ Google Scholar ]
- Logue A.W, King G.R, Chavarro A, Volpe A.S. Matching and maximizing in a self-control paradigm using human subjects. Learning and Motivation. 1990; 21 :340–368. [ Google Scholar ]
- Logue A.W, Pena-Correal T.E, Rodriguez M.L, Kabela E. Self-control in adult humans: Variation in positive reinforcer amount and delay. Journal of the Experimental Analysis of Behavior. 1986; 46 :159–173. [ PMC free article ] [ PubMed ] [ Google Scholar ]
- Lubinski D, Thompson T. An animal model of the interpersonal communication of interoceptive (private) states. Journal of the Experimental Analysis of Behavior. 1987; 48 :1–15. [ PMC free article ] [ PubMed ] [ Google Scholar ]
- Madden G.J, Bickel W.K, Jacobs E.A. Three predictions of the economic concept of unit price in a choice context. Journal of the Experimental Analysis of Behavior. 2000; 73 :45–64. [ PMC free article ] [ PubMed ] [ Google Scholar ]
- Magoon M.A, Critchfield T.S. Concurrent schedules of positive and negative reinforcement: Differential-impact and differential-outcomes hypotheses. Journal of the Experimental Analysis of Behavior. 2008; 90 :1–22. [ PMC free article ] [ PubMed ] [ Google Scholar ]
- Malagodi E.F. Acquisition of the token-reward habit in the rat. Psychological Reports. 1967a; 20 :1335–1342. [ PubMed ] [ Google Scholar ]
- Malagodi E.F. Fixed-ratio schedules of token reinforcement. Psychonomic Science. 1967b; 8 :469–470. [ Google Scholar ]
- Malagodi E.F. Variable-interval schedules of token reinforcement. Psychonomic Science. 1967c; 8 :471–472. [ Google Scholar ]
- Malagodi E.F. University of Miami; 1967d. Second-order chained and tandem schedules of token-reinforcement in the rat. Unpublished doctoral dissertation, [ Google Scholar ]
- Malagodi E.F, Webbe F.M, Waddell T.R. Second-order schedules of token reinforcement: Effects of varying the schedule of food presentation. Journal of the Experimental Analysis of Behavior. 1975; 24 :173–181. [ PMC free article ] [ PubMed ] [ Google Scholar ]
- Mazur J.E. Steady-state performance on fixed-, mixed-, and random-ratio schedules. Journal of the Experimental Analysis of Behavior. 1983; 39 :293–307. [ PMC free article ] [ PubMed ] [ Google Scholar ]
- McClure S.M, Laibson D.I, Loewenstein G, Cohen J.D. Separate reward systems value immediate and delayed monetary rewards. Science. 2004; 306 :503–507. [ PubMed ] [ Google Scholar ]
- McFarland J.M, Lattal K.A. Determinants of reinforcer accumulation during an operant task. Journal of the Experimental Analysis of Behavior. 2001; 76 :321–338. [ PMC free article ] [ PubMed ] [ Google Scholar ]
- Midgley M, Lea S.E.G, Kirby R.M. Algorithmic shaping and misbehavior in the acquisition of token deposit by rats. Journal of the Experimental Analysis of Behavior. 1989; 52 :27–40. [ PMC free article ] [ PubMed ] [ Google Scholar ]
- Munson K.J, Crosbie J. Effects of response cost in computerized programmed instruction. Psychological Record. 1998; 48 :233–251. [ Google Scholar ]
- Myers W.A, Trapold M.A. Two failures to demonstrate superiority of a generalized secondary reinforcer. Psychonomic Science. 1966; 5 :321–322. [ Google Scholar ]
- O'Donnell J, Crosbie J, Williams D.C, Saunders K.J. Stimulus control and generalization of point-loss punishment with humans. Journal of the Experimental Analysis of Behavior. 2000; 73 :261–274. [ PMC free article ] [ PubMed ] [ Google Scholar ]
- Pietras C.J, Hackenberg T.D. Response-cost punishment via token-loss with pigeons. Behavioural Processes. 2005; 69 :343–356. [ PubMed ] [ Google Scholar ]
- Raiff B.R, Bullock C.E, Hackenberg T.D. Response-cost punishment with pigeons: Further evidence of response suppression via token loss. Learning & Behavior. 2008; 36 :29–41. [ PubMed ] [ Google Scholar ]
- Rasmussen E.B, Newland M.C. Asymmetry of reinforcement and punishment in human choice. Journal of the Experimental Analysis of Behavior. 2008; 89 :157–167. [ PMC free article ] [ PubMed ] [ Google Scholar ]
- Roane H.S, Call N.A, Falcomata T.S. A preliminary analysis of adaptive responding under open and closed economies. Journal of Applied Behavior Analysis. 2005; 38 :335–348. [ PMC free article ] [ PubMed ] [ Google Scholar ]
- Roll J.M, Reilly M.P, Johanson C. The influence of exchange delays on cigarette versus money choice: A laboratory analog of voucher-based reinforcement therapy. Experimental and Clinical Psychopharmacology. 2000; 8 :366–370. [ PubMed ] [ Google Scholar ]
- Schmandt-Besserant D. Before writing: Volume 1: From counting to cuneiform. Austin, TX: University of Texas Press; 1992. [ Google Scholar ]
- Silberberg A, Roma P.G, Huntsberry M.E, Warren-Boulton F.R, Sakagami T, Ruggiero A.M, et al. On loss aversion in capuchin monkeys. Journal of the Experimental Analysis of Behavior. 2008; 89 :145–155. [ PMC free article ] [ PubMed ] [ Google Scholar ]
- Silverman K. Exploring the limits and utility of operant conditioning in the treatment of drug addiction. The Behavior Analyst. 2004; 27 :209–230. [ PMC free article ] [ PubMed ] [ Google Scholar ]
- Skinner B.F. Science and human behavior. New York: Macmillan; 1953. [ Google Scholar ]
- Sousa C, Matsuzawa T. The use of tokens as rewards and tools by chimpanzees ( Pan troglodytes ) Animal Cognition. 2001; 4 :213–221. [ PubMed ] [ Google Scholar ]
- Thaler R.H, editor. Advances in Behavioral Finance. Russell Sage Foundation; 1993. [ Google Scholar ]
- Tustin R.D. Preference for reinforcers under varying schedule arrangements: A behavioral economic analysis. Journal of Applied Behavior Analysis. 1994; 27 :597–606. [ PMC free article ] [ PubMed ] [ Google Scholar ]
- Tversky A, Kahneman D. Judgment under uncertainty: Heuristics and biases. Science. 1974; 185 :1124–1131. [ PubMed ] [ Google Scholar ]
- Tversky A, Kahneman D. Advances in prospect theory: Cumulative representation of uncertainty. Journal of Risk and Uncertainty. 1992; 5 :297–323. [ Google Scholar ]
- Waddell T.R, Leander J.D, Webbe F.M, Malagodi E.F. Schedule interactions in second-order fixed-interval (fixed-ratio) schedules of token reinforcement. Learning and Motivation. 1972; 3 :91–100. [ Google Scholar ]
- Webbe F.M, Malagodi E.F. Second-order schedules of token reinforcement: Comparisons of performance under fixed-ratio and variable-ratio exchange schedules. Journal of the Experimental Analysis of Behavior. 1978; 30 :219–224. [ PMC free article ] [ PubMed ] [ Google Scholar ]
- Weiner H. Some effects of response cost upon human operant behavior. Journal of the Experimental Analysis of Behavior. 1962; 5 :201–208. [ PMC free article ] [ PubMed ] [ Google Scholar ]
- Weiner H. Some effects of response cost and aversive control of human operant behavior. Journal of the Experimental Analysis of Behavior. 1963; 6 :415–421. [ PMC free article ] [ PubMed ] [ Google Scholar ]
- Weiner H. Response cost and fixed-ratio performance. Journal of the Experimental Analysis of Behavior. 1964; 7 :79–81. [ PMC free article ] [ PubMed ] [ Google Scholar ]
- Williams B.A. Marking and bridging versus conditioned reinforcement. Animal Learning & Behavior. 1991; 19 :264–269. [ Google Scholar ]
- Williams B.A. Conditioned reinforcement: Experimental and theoretical issues. The Behavior Analyst. 1994a; 17 :261–285. [ PMC free article ] [ PubMed ] [ Google Scholar ]
- Williams B.A. Conditioned reinforcement: Neglected or outmoded explanatory construct. Psychonomic Bulletin & Review. 1994b; 1 :457–475. [ PubMed ] [ Google Scholar ]
- Williams B.F, Williams R.L, McLaughlin T.F. The use of token economies with individuals who have developmental disabilities. In: Cipani E, editor. The treatment of severe behavior disorders. Washington, DC: AAMR Publications; 1989. pp. 3–18. [ PubMed ] [ Google Scholar ]
- Winkler R.C. Management of chronic psychiatric patients by a token reinforcement system. Journal of Applied Behavior Analysis. 1970; 3 :47–55. [ PMC free article ] [ PubMed ] [ Google Scholar ]
- Winkler R.C. An experimental analysis of economic balance, savings, and wages in a token economy. Behavior Therapy. 1973; 4 :22–40. [ Google Scholar ]
- Winkler R.C. Behavioral economics, token economies, and applied behavior analysis. In: Staddon J.E.R, editor. Limits to action. New York: Academic Press; 1980. pp. 269–297. [ Google Scholar ]
- Wolfe J.B. Effectiveness of token rewards for chimpanzees. Comparative Psychological Monographs. 1936; 12 :1–72. [ Google Scholar ]
- Yankelevitz R.L, Bullock C.E, Hackenberg T.D. Reinforcer accumulation in a token-reinforcement context. Journal of the Experimental Analysis of Behavior. 2008; 90 :283–299. [ PMC free article ] [ PubMed ] [ Google Scholar ]
- Yerkes R.M. Chimpanzees: A Laboratory colony. New Haven, CT: Yake University Press; 1943. [ Google Scholar ]

Token Economy in ABA: Examples and Benefits
- by [email protected]
- April 14, 2024
In the field of applied behavior analysis (ABA), a token economy is a structured method designed to reinforce healthy and adaptive behaviors. It serves as a visual representation of progress, allowing individuals to earn tokens for demonstrating target behaviors and eventually exchange them for desired reinforcers.
This systematic approach has proven to be particularly beneficial for individuals with autism and other neurodevelopmental differences.
What is a Token Economy?
A token economy is a behavior management system that utilizes tokens as a form of conditioned reinforcement. Tokens can be in the form of physical objects, such as coins, chips, or points, or they can be represented digitally. These tokens hold no intrinsic value but serve as a symbolic representation of progress and the opportunity to access desired reinforcers.
The token economy process in ABA is developed by Behavior Analysts (BCBAs) who tailor it to the specific needs of each learner or group. The process involves delivering tokens as a tangible conditioned reinforcer for demonstrating target behaviors. These behaviors can include tasks completion, following instructions, appropriate social interaction, or any other behavior that is being targeted for improvement.

How Token Economies Benefit Individuals with Autism
Token economies have demonstrated numerous benefits for individuals with autism. By providing a visual representation of progress and reinforcement, they create a structured and predictable environment that can enhance learning and behavior management.
One significant advantage of token economies in ABA therapy is that they allow for the reinforcement of desired behaviors in real-time. When individuals with autism engage in target behaviors, they are immediately rewarded with tokens. This immediate reinforcement strengthens the connection between the behavior and the desired outcome.
Token economies also provide individuals with autism the opportunity to understand the concept of delayed gratification. By accumulating tokens over time, they learn the value of patience and the importance of working towards a desired outcome. This skill can be invaluable in various areas of life, including academics, vocational training, and social interactions.
Moreover, token economies allow for individualization and customization. Each learner’s token economy can be tailored to their specific needs, preferences, and goals. This personalized approach ensures that the reinforcement system is motivating and meaningful to the individual, increasing the likelihood of success.
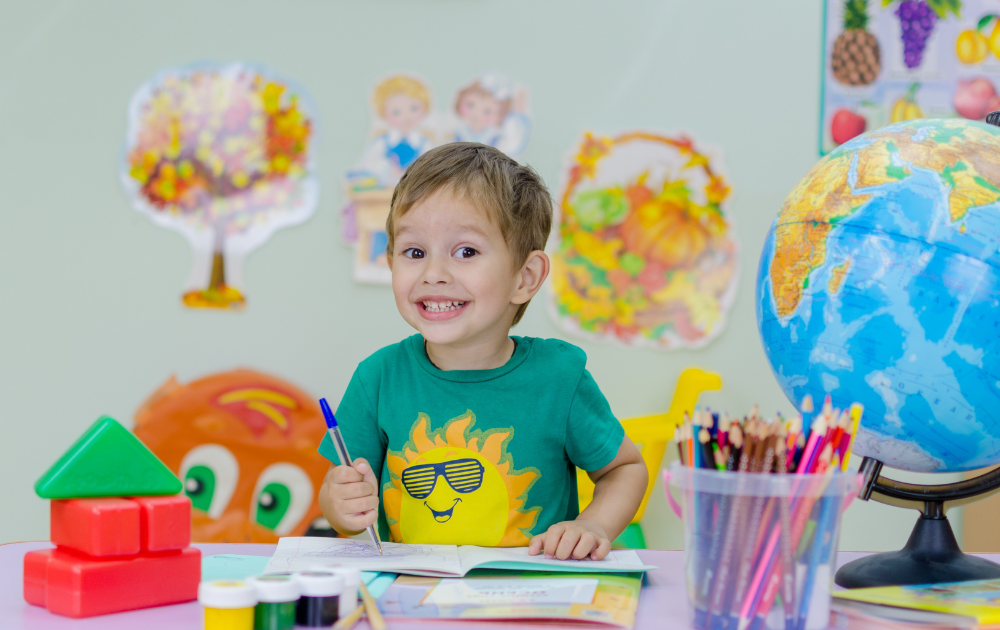
Implementing a Token System
When it comes to implementing a token system as part of Applied Behavior Analysis (ABA) therapy, there are two key factors to consider: the development of the system by behavior analysts and the token reinforcement process itself.
Development by Behavior Analysts
The creation of a token system in ABA therapy is a collaborative effort between behavior analysts (BCBAs) and the individuals involved. Behavior analysts tailor the token economy to the specific needs and goals of each learner or group. This customization ensures that the system is effective and meaningful for the individuals participating in the therapy.
The development process begins with identifying target behaviors that the token system will reinforce. These behaviors are selected based on their relevance to the individual’s treatment goals and the behavior analyst’s expertise. The system is designed to track and reinforce these specific behaviors, promoting their frequency and duration.
Token Reinforcement Process
The token reinforcement process is a fundamental aspect of a token economy system. It involves the delivery of tokens as conditioned reinforcers for demonstrating target behaviors. Tokens, which can take the form of physical tokens, points, stickers, or other tangible items, are initially paired with primary reinforcers, such as praise or preferred items.
During therapy sessions, tokens are provided immediately following the occurrence of the target behavior. The tokens serve as a visual representation of the individual’s progress and act as a bridge between the behavior and the eventual access to backup reinforcers. These backup reinforcers are items or activities that the individual finds motivating and are exchanged for a predetermined number of earned tokens.
The token reinforcement process operates on the principle of positive reinforcement, encouraging the repetition of desired behaviors. It helps individuals with autism and other neurodevelopmental differences understand the connection between their actions and the rewards they can earn through the token economy system.
By reinforcing specific behaviors through the token system, individuals not only earn access to backup reinforcers but also learn valuable skills such as delayed gratification, self-control, and goal-setting. This process can greatly enhance the effectiveness of ABA therapy in promoting positive behavior change.

Token vs. Primary Reinforcers
In the field of applied behavior analysis (ABA), a token economy is a structured method designed to reinforce healthy and adaptive behaviors. It involves the use of tokens as conditioned reinforcers, which can be exchanged for backup reinforcers, reinforcing certain behaviors through the token economy system. Understanding the distinction between tokens and primary reinforcers is essential in comprehending the role of tokens in ABA therapy.
Role of Tokens in ABA Therapy
In ABA therapy, tokens play a crucial role as secondary or conditioned reinforcers. Rather than being innately valuable like primary reinforcers, tokens gain their reinforcing value through association with other reinforcers. For example, a token may be given to an individual with autism for completing a specific task or exhibiting a desirable behavior. These tokens can later be exchanged for primary reinforcers such as preferred items, activities, or privileges.
The use of tokens in ABA therapy serves several purposes. Firstly, tokens provide a visual representation of progress and reinforcement, helping individuals with autism understand their accomplishments and work towards earning rewards.
This visual system can enhance motivation and engagement in therapy sessions. Secondly, tokens enable the reinforcement of desired behaviors in real-time, as they can be immediately delivered upon the occurrence of the targeted behavior. This immediate reinforcement facilitates the learning process and helps individuals with autism understand the connection between their actions and the consequences.
Contrasting Token and Primary Reinforcers
Token reinforcers differ from primary reinforcers in their nature and function. Primary reinforcers are inherently valuable to all humans, as they fulfill physiological needs. Examples of primary reinforcers include food, water, shelter, and warmth. These reinforcers have an immediate and direct impact on an individual’s well-being and are universally desirable.
On the other hand, tokens in a token economy system are not valuable in and of themselves. Their value lies in their association with the backup reinforcers they can be exchanged for. It’s important to note that the specific backup reinforcers used in a token economy should be tailored to each individual’s preferences and interests. This personalization ensures that the tokens hold value and act as effective reinforcers for the desired behaviors.
With tokens as secondary reinforcers, ABA therapists can reinforce positive behaviors, track progress, and promote skill development in individuals with autism and other neurodevelopmental differences.
Effectiveness Across Neurodiversities
Token economies, commonly used in ABA Therapy Services , have proven to be effective not only for children with autism but also for individuals with other neurodevelopmental differences. These structured reinforcement systems provide valuable benefits and support in promoting positive behaviors and skill development.
Benefits for Children with Autism
The structured nature of a token economy helps provide clear expectations and consistent reinforcement, making it easier for children with autism to understand and engage in desired behaviors. Tokens serve as a tangible representation of progress, allowing individuals to see their accomplishments and work towards earning desired rewards.
ABA therapy, which often incorporates token economies, focuses on teaching and reinforcing specific skills and behaviors. By utilizing token systems, therapists and caregivers can effectively reinforce desired behaviors and provide immediate feedback, promoting skill acquisition and reducing problem behaviors.
Token economies are not limited to children with autism alone. These structured reinforcement systems can be effective for individuals with other neurodevelopmental differences as well, including attention deficit hyperactivity disorder (ADHD) and other behavioral challenges.
Children with ADHD often struggle with impulse control, attention, and hyperactivity. Token economies offer a structured approach to promoting self-regulation and positive behavior. The visual nature of the system helps individuals with ADHD stay focused and motivated, as they can observe their progress and work towards earning rewards.
Furthermore, token economies can be adapted to support individuals with various neurodevelopmental differences, such as intellectual disabilities or learning disorders. The flexibility of these systems allows for individual tailoring to meet specific needs and promote positive change.
Designing a Token Economy
Tailoring to individual learners.
Token economies should be designed to meet the specific needs and abilities of each individual learner. This customization allows for a more personalized approach to behavior management and ensures that the system is motivating and meaningful for the individual. Here are some considerations when tailoring a token economy to an individual learner:
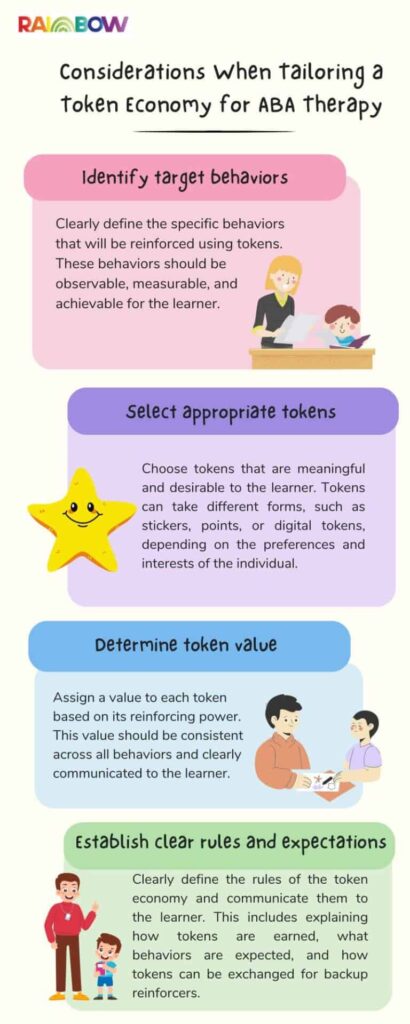
By tailoring the token economy to the individual learner, the system becomes more effective in promoting positive behavior change and increasing motivation.
Structured Reinforcement Systems
In a token economy, tokens serve as conditioned reinforcers that are delivered contingent on engaging in specific target behaviors. These tokens then gain their reinforcing value through association with other backup reinforcers. Implementing a structured reinforcement system is crucial for maintaining consistency and ensuring the effectiveness of the token economy. Here are some considerations when implementing structured reinforcement systems:
- Define backup reinforcers: Determine the backup reinforcers that the individual will be able to access once a specific number of tokens has been earned. These backup reinforcers should be highly motivating and meaningful to the learner.
- Establish clear exchange rates: Set clear guidelines for the number of tokens required to exchange for backup reinforcers. This exchange rate should be consistent and transparent to ensure fairness and predictability.
- Monitor token accumulation: Regularly track and monitor the number of tokens earned by the learner. This allows for reinforcement delivery at the appropriate time and helps the learner understand their progress.
- Provide feedback and reinforcement: Continuously provide feedback to the learner regarding their performance and progress within the token economy. This feedback, along with reinforcing the exchange of tokens for backup reinforcers, helps to maintain the learner’s motivation and engagement.
Designing a token economy involves tailoring the system to the individual learner and implementing structured reinforcement systems. In a token economy, when tokens are no longer given for a particular behavior, the individual may initially show an extinction burst , seeking the previously reinforced behavior more vigorously before it diminishes.
By personalizing the token economy and providing clear guidelines for reinforcement, this approach can effectively promote positive behavior change and maximize its benefits within the context of ABA therapy.
- https://centralreach.com/blog/token-economy-aba/#:~:text=Tokens%20are%20earned%20for%20engaging,Patrol%20stickers%20for%20their%20tokens .
- https://strategiesforspecialinterventions.weebly.com/token-system-and-token-economies.html
- https://www.education.uw.edu/ibestt/wp-content/uploads/2018/02/Token-System.pdf
Recent Posts
- Sulforaphane Treatment of Autism Spectrum Disorder
- Camel Milk for Autism: a Review
- Risperidone for Autism: Side Effects and Considerations
- Serotonin Autism Treatment: Key Considerations
- Best Melatonin for Autistic Child
Recent Comments
- February 2024
- January 2024
- December 2023
- November 2023
- October 2023
- September 2023
- August 2023
- ABA Therapy
- Autism and Mental Health
- Autism and School
- Autism Causes
- Autism Comorbidities
- autism diagnosis
- Autism Interventions
- Autism Statistics
- Autism Tools
- communication
- Parents' Guide
- Uncategorized
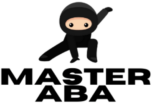
Token Economy: Examples and Applications in ABA
Within the field of Applied Behavior Analysis (ABA), professionals use reinforcement to strengthen behavior. Decades of research support the use of token economy as a means of delivering reinforcement in a variety of settings to address diverse behaviors (Matson & Boisjoli, 2009; Boniecki & Moore, 2203; Carnett et al., 2014). Token economy offers a flexible way to deliver reinforcement to meet the needs of learners and interventionists.
Token economy is a reinforcement strategy where generalized reinforcers (tokens) are exchanged for backup reinforcers (something the learner wants). Interventionists create token economy systems that reinforce skills such as academics, communication, self-help, or prosocial behavior (Matson & Boisjoli, 2009). Research supports the use of token economy in various environments including schools, homes, summer camps, and inpatient programs. This broad applicability makes it an essential tool in your ABA toolbox.
Related Posts

The World Economy as a Token Economy System Introducing Token Economy Creating Token Systems that Motivate Token Economy Examples Advantages and Disadvantages of Token Economy Other Considerations for Token Economy Ethical Considerations When Implementing Token Economy Research Related to Implementing Token Economy References and Related Reading
The World Economy as a Token Economy System
Token economy works much like our world economy where money serves as the token that allows for the purchase of backup reinforcers (i.e. anything we want of comparable value). As adults, we go to work to earn money. The money by itself isn’t worth anything. It’s a piece of paper or a lump of metal. But it has value because it can be exchanged for things we need and want. With money, we can buy food, shelter, and entertainment.
Watch this quick video comparing token economy to the world economy:
Viewing token economy from this perspective helps professionals utilize the strategy effectively. If you wouldn’t work all week for a few pretzels, don’t expect your learner to complete a mountain of work for something they don’t value. The amount of work needed to earn the tokens must match the learner’s perceived effort.

Introducing Token Economy
How you introduce token economy to your learner depends on many factors including the skills and interests of the learner, resources available, and who will deliver the tokens. You must first decide:
- What tokens you will use
- What criteria the learner must meet to earn a token
- How many tokens the learner must earn to trade for the backup reinforcer
- What backup reinforcers you will offer the learner
Token economies range from very simple to highly complex. Any system where generalized reinforcers (reinforcers with no inherent value) are exchanged for backup reinforcers (something of value to the individual). The right system depends on the skills of both the interventionist and the learner.
When to Use Token Economy
Although you can apply token economy widely, there are several factors to consider before choosing this type of reinforcement. Use token economy:
- With learners who benefit from structured reinforcement
- When you want to avoid an FR1 ratio of reinforcement, but your learner continues to require frequent reinforcement
- You need an intervention that’s easy to use
- When your learner quickly satiates on available tangible reinforcers
Token economy is effective for many different types of learners. Learners with a lot of language quickly develop an understanding of the value of tokens. Simply explain what they earn in exchange for a designated number of tokens. For children with sufficient language to understand when you explain the contingency, clearly spell out the criteria for earning tokens and what the choices the child has for a backup reinforcer. Many children, even those with a solid vocabulary, benefit from visual representations for both the expectations and the reinforcer choices.
How to teach that tokens have value
For learners with more limited language abilities, token economy can still be an effective intervention, but you must teach the value of tokens in a different way. For these learners:
- Begin with a simple token board that clearly shows where tokens belong on the board.
- Remove only 1 token for the board.
- When the learner earns a token, provide immediate tangible reinforcement along with the token and verbal praise “Nice work! You earned a token! Here’s ____.”
- After the learner has several opportunities to earn the identified reinforcer, remove 2 tokens from the board.
- When the learner earns the first token, pair that token with a reinforcer (ideally something edible or something else you don’t need to remove such as physical touch) along with verbal praise “You did it! You earned a token! One more to go!”
- When the learner earns the second token, provide immediate tangible reinforcement along with the token and verbal praise “Nice work! You earned a token! Here’s ____.”
- Continue to pair tokens with established reinforcers while gradually removing more tokens from the board until the learner needs to earn all tokens on his board to exchange for the backup reinforcer.
- Gradually reduce pairing the token with other tangible reinforcers, but continue to pair them with verbal praise.
Creating Token Systems that Motivate
Tokens can be physical objects such as a coin, poker chip, ticket, or sticker, or even a checkmark on a board or piece of paper. With a little creativity, you can create a token system that motivates your learner beyond receiving the backup reinforcer. Carnett et al. (2014) discovered that using tokens of perseverative interests of the learner resulted in a greater treatment effect. What this means is that if your learner has a special interest in a particular topic, you should use that topic to create your token economy system.
Token Economy and Intrinsic Motivation
When you begin using reinforcement, motivation comes from external sources (the reinforcer). The child completes the task and receives a reinforcer as a result. As the child becomes comfortable with the use of reinforcement, switching to a token economy system delays the reinforcement. Over time you can thin the reinforcement as the child builds intrinsic motivation.
As professionals, we often hear questions such as:
“Wouldn’t it be better if the child were intrinsically motivated to do the things you wanted him to do?”
The answer to this question is both yes and no. If the child is not already intrinsically motivated to do something, the chances of that just starting to happen is minimal, especially if that child has autism. In reality, we all require some external motivation for various tasks we don’t want to do. Additionally, using some of the techniques of ABA, you can begin to build some of this intrinsic motivation.
According to Verywell Mind , “intrinsic motivation refers to behavior that is driven by internal rewards” When someone is intrinsically motivated to engage in a particular behavior, the individual finds that behavior to be naturally satisfying. The behavior is its own reinforcer.
Many children are intrinsically motivated to please their parents or teachers; however, many children with autism are not motivated by these social factors. Children with autism experience social deficits that often impact the value of social interactions.
ABA steps in when intrinsic motivation is insufficient. Through the use of techniques such as token economy, pairing and thinning of reinforcement schedules, you can help build intrinsic motivation for a wide variety of behaviors.
Token Economy Example to Build Intrinsic Motivation
Take a look at this example of token economy in practice:
You want to teach your client, Craig, to become more intrinsically motivated to complete a puzzle. You chose this activity because Craig struggles with engaging in appropriate leisure activities and often engages in challenging behavior when he has nothing to do. Currently, Craig completes a 12 piece jigsaw puzzle and receives a Goldfish cracker after he puts together each piece. It takes him about 5 minutes to finish the puzzle.
You find a 24 piece puzzle and get started. You set out a token board with 12 tokens. Initially, you pair each token with a Goldfish cracker and when Craig earns all 12 tokens, he will trade them in for 3 minutes of his favorite video. For this first puzzle, he earns a token for each piece he puts together and with each token, he gets a cracker. He stops the puzzle halfway through to watch the video and earns another 3 minutes when he completes the puzzle. This puzzle took him 20 minutes to complete and he appeared happy to get the Goldfish and watch the video.
Thinning the Schedule
Over time, you thin the schedule of reinforcement using the token economy system. He begins to earn a toke for every 2 pieces he puts together, then every 3. You continue to pair the tokens with social praise and incorporate social praise in between the delivery of tokens.
Over time, Craig begins to tackle 50 piece puzzles and completes the whole puzzle before earning a token. Through the process of pairing with reinforcement and thinning the schedule of reinforcement, Craig has become somewhat intrinsically motivated to complete the puzzle. With continued exposure to this pairing process, the puzzle itself may actually be able to serve as a reinforcer.
Learn More about Intrinsic Motivation and Token Economy
Research shows that social reinforcement may lead to greater intrinsic motivation. The question remains for children with autism, how do you build the value of social exchanges for children who have significant social deficits. The use of token economy and pairing may be part of the answer. Check out these articles to learn more about token economy:
Ayllon, Teodoro & Azrin, Nathan. The Token Economy: A Motivational System for Therapy and Rehabilitation . Appleton Century Crofts (June 1968).
Kazdin, A. E. (1982). The token economy: A decade later . Journal of applied behavior analysis , 15(3), 431-445.
McLaughlin, T. F., & Malaby, J. (1972). INTRINSIC REINFORCERS IN A CLASSROOM TOKEN ECONOMY 1 . Journal of Applied Behavior Analysis , 5(3), 263-270.
Deci, E. L. (1972). Intrinsic motivation, extrinsic reinforcement, and inequity . Journal of personality and social psychology , 22(1), 113.

Token Economy Examples
For some learners, simple token boards encourage learners to work to collect their tokens to earn the backup reinforcer. Many of us are familiar with the type of token board pictured below. There is a spot to indicate what the learner is working for as well as 4 spaces to show the learner must earn 4 tokens to exchange for the backup reinforcer.

While the board above might result in improved responding, using a fun token board improves motivation and the value of tokens beyond the value of the backup reinforcer. A board such as the one pictured below holds the interest of a child who enjoys rockets (you can download this one below).

With access to technology increasing across environments, and the interest so many learners have in technology, consider using a digital animated token board. The video below shows a demonstration of some premade animated token boards available only through our Master ABA Dojo membership. They are easy to use on any device such as a computer, tablet, or smartphone.
The video below shows you how you can make your own animated token boards in Keynote. Although the video demonstrates how to do it in Keynote, many of the steps are the same in PowerPoint.
The following video describes the use of token economy and provides an example of using a digital token board when doing compliance training with a learner:
Examples of Token Economy at Home
Token economy is a great intervention to use at home. It’s easy for parents to implement and doesn’t require extensive resources. It’s a flexible intervention that parents can apply to a variety of behaviors so they don’t need to learn a whole array of interventions.
Simple token systems
Simple token boards like the ones above can be used to reinforce cooperative behavior, homework completion, or even play skills. Parents who don’t have access to a laminator or who would prefer not to spend the time creating a token board can easily purchase them on Amazon.
Parents should identify which behavior they want to reinforce. Limiting the delivery of tokens for only one behavior increases the chance of successful implementation. Parents can reinforce:
- Turn-taking
- Steps for brushing teeth
- Sitting at the table for mealtime (deliver the token every x minutes)
- Cleaning up toys
- Following directions
- Generalization of ABA programming such as motor imitation or listener responding programs
Once parents know what behavior to reinforce, they need to select backup reinforcers. Many learners enjoy a “prize box” filled with small items (usually from a dollar store) that they can choose from when they earn a reward, but if parents deliver tokens frequently, this becomes unmanageable. Parents often know what motivates their child, but struggle with limiting access to these things, making them ineffective reinforcers. Learners must only have access to the backup reinforcers for the token economy only when they earn them through the token board. The more frequently parents deliver tokens, the smaller the reinforcer they should offer. Possible reinforcers include:
- Time with a favorite toy
- A special art project with a parent or sibling
- TV or video game time
- Making a silly video with a parent and then watching it
- Time outside
- Taking a walk
- A favorite treat
Sometimes your learner wants something bigger and must earn it over time. Your learner may be ready for this if he already has an understanding of token economy. Building on this foundation you can teach him the jar system.
Here’s an example of the jar system:
- Identify what your learner wants to work for (i.e. a trip to Chuck E. Cheese, a game for his game system, going to the movies, etc You may need to work with the learner’s caregiver to identify a reinforcer that will be meaningful)
- Find a clear plastic jar and many small items you will use to fill the jar (i.e. colorful pom poms, unifix cubes, colorful pebbles, etc.). The size of the jar will depend on the size of the items you choose to fill the jar. You want the jar big enough to holed 20-30 of the items, but not so big it would take 100 items to fill the jar.
- Each time you see your learner engage in the target behavior give him one of the small items. State clearly what he did that you noticed. For example, “You did such a great job putting your shoes on when I asked! Great job! Here’s a pom pom for your jar!”
- Your learner should collect these items in the jar.
- When the jar is full, he has earned the reinforcer he was working for (identified in step 1 above).
This system works best for children with some advanced language comprehension skills, but you can adapt it to help children who don’t communicate verbally. For these children, start much smaller and work up to your learner understanding this type of system. Take your time and wait for your learner to be ready so you don’t frustrate him or yourself.
Get Creative!
You can be creative with the jar system to keep your learner interested and motivated. If your learner wants to go to Chuck E. Cheese, schedule a time when her caregiver can take her that’s maybe 2 weeks away. Ask the caregiver to pre-buy the maximum number of game tokens they are willing to let your learner have. Each time you see your learner engaging in the target behavior give him one of the tokens to put in the jar. When it’s time for the big trip to the restaurant, he can use all of the tokens in the jar. Instruct the caregiver not to buy more tokens while they are there.
Helpful Tips for a Token Economy or Jar System
- Once your learner earns a token or a piece for the jar, do not take them away for bad behavior.
- When first teaching the system, be generous with the tokens or pieces for the jar so that he is able to earn the reinforcer quickly.
- Always tell your learner what he did to earn the token or piece for the jar.
- Be enthusiastic when giving praise.
- Don’t argue, negotiate with, or scold your learner when they are not engaging in the target behavior.
More complex token systems
Learners who have more advanced skills may benefit from a point or other system where learners can access a bigger reward. Parents again, should choose which behavior to reinforce, but with a more complex system could choose more than one behavior. For example, the parent may award points for completing chores and being kind to a sibling as long as the learner understands which behaviors earn reinforcement.
While parents can tally points on a dry erase board or add pom poms to a jar to show progress toward a goal, the system below, Amazon provides a simple solution for rewarding multiple behaviors. Parents can assign a behavior to each color, then set the goal for each behavior by adjusting the ring. Parents can attach different rewards to each behavior or allow their child to work toward one big goal. This more complex system can reinforce behavior across a longer amount of time such as a week or even a month.
Behaviors appropriate for this type of system include:
- Homework completion
- Sleeping in their own bed
- Leaving for school without problem behavior
- Completing the bedtime routine each night
Since it takes learners a longer amount of time to contact reinforcement, the reinforcer offered should match the learner’s perceived effort. Again, parents must limit access to the selected reinforcers when the learner hasn’t yet earned the reward. Parents can get creative to keep their child motivated. Reinforcers might include:
- A trip to a favorite arcade, restaurant, or zoo
- Video game time with a parent
- A special toy they have wanted from the store
- A trip to see something of special interest in the community (such as the UPS store for a child whose special interest is shipping)
Token economy is a great tool for use by parents. Help them come up with fun ideas to motivate their child to do those things he’s struggling to do.
Examples of Token Economy at School
In much the same way that token economies benefit parents at home, they are a wonderful tool for teachers at school. Teachers can create simple systems for individual learners or develop a more complex system to address the behavior of each student in the class. Teachers can reinforce behaviors such as:
- Raising a hand for a turn to speak
- Sitting quietly
- Staying on task
- Walking quietly in the hallway
- Helping a peer
- Cleaning up materials
- Completion of academic tasks
- Standing safely in line
Teachers are busy and are pulled in many different directions at once. Token economy lends itself for the quick delivery of reinforcement throughout the day with more time spent delivering the backup reinforcer at a more convenient time. Backup reinforcers in schools may include:
- Items selected from a prize box or “store”
- Lunch with the teacher
- Extra recess time
- Time spent with a favorite toy
- A movie party
Teachers can deliver reinforcers to the class as a whole or to individual students depending on the needs of her class. The flexibility of token economy allows teachers to use the intervention as needed and then change the intervention as the needs of the class change.
Advantages and Disadvantages of Token Economy
- Teaches delayed gratification. Your learner doesn’t get the reinforcer immediately. He must earn several tokens to be able to earn the reinforcer. This makes a token economy a natural next step after you begin using reinforcement routinely with your learner.
- Is flexible. A token economy is a framework that can be used to reinforce any behavior. You can require as many tokens, or as few, as is appropriate for the learner and the task. You can use any small items as a token, which allows you to choose items that may be of interest to your learner. You can purchase a token board or easily create one yourself.
- Allows you to reinforce behaviors when the backup reinforcer isn’t available. With reinforcement you need to reinforce the behavior as soon as it happens. If your backup reinforcer isn’t something that is immediately available all the time, tokens can help bridge the gap between the behavior and the backup reinforcer.
- Keeps your learner engaged. Over time children tend to become satiated or bored with reinforcers they receive all the time. Children often find a token economy fun and helps reduce the number of times the learner receives the backup reinforcer.
- Can be used with multiple children. A token economy is easy to use with multiple children who might be working toward different backup reinforcers.
- Is consistent. Planning a token economy helps keep you and others working with your learner reinforcing behavior consistently. This is the best way to ensure effective change.
- Teaches a real-world concept. The world economy is essentially a token economy. When you teach your learner that tokens have value, he will have an easier time understanding the value of money as he gets older.
Disadvantages
- Tokens don’t have innate value. Even after trying to teach your learner the value of the token, he might struggle to understand this idea and become frustrated with this process.
- A token economy takes planning. For a token economy to be effective you need to be sure your learner can receive, store and keep track of his tokens any time he completes the task or behavior. You need to plan ahead to be sure you can achieve this.
- Your learner might lose interest or become frustrated. If he doesn’t learn the value in the tokens, the backup reinforcer isn’t motivating or your learner doesn’t earn tokens fast enough, he might lose interest or become frustrated with a token economy. If your system doesn’t seem to be working try choosing a different backup reinforcer or making it easier for your learner to earn the tokens. You can continue to adjust over time as your learner becomes more comfortable with the process.
Other Considerations for Token Economy
While token economy is a simple intervention, many factors influence its effectiveness. A learner must understand the trade-in value of the tokens. Some learners understand when you explain the concept of tokens to them, but others must experience the association for themselves. This requires systematic pairing and teaching strategies as explained above. Below are some additional things to consider when implementing token economy with your learners.
Thinning the Schedule of Reinforcement with Token Economy
Token economies allow for a structured way to thin the schedule of reinforcement without losing the effectiveness of the reinforcer. A child who progresses from a dense schedule of reinforcement to a thinner schedule may show reduced responding as the response effort needed to achieve reinforcement increases. Token economy can mitigate this effect by providing some lower quality reinforcement between the delivery of the ultimate reinforcer. When thinning the schedule, ensure to do so gradually to maintain the reinforcing value.
Here’s an example:
You successfully thinned the schedule of reinforcement from a FR1 to a FR4 without a noticeable decrease in responding. When you continued to thin the schedule, you observed an increase in problem behavior and a decrease in responding. You introduce the token board with 4 tokens. After teaching your client about the value of tokens, you gradually begin to deliver tokens on a VR2 schedule, leading to delivery of the actual reinforcer on a VR8 schedule.
For more information about schedules of reinforcement, read our post: Understanding Consequence Interventions: Punishment vs Reinforcement .
Satiation of the Reinforcer Options
Most children eventually become satiated on reinforcers you present over and over again. Preferences also change over time. For this reason, you should conduct regular preference or reinforcer assessments. If you’re not sure what the difference is, read this post Choosing Reinforcers: Reinforcer Assessments or Preference Assessments .
For many learners, frequent preference assessments are necessary to maintain motivation. Learners with sufficient vocabulary may simply tell you what they want to work for. Other learners need a more structured assessment.
Use of Response Cost
Response cost is a punishment procedure that may be used with token economy. When implementing response cost, specific behaviors lead to the removal of tokens earned. While this may seem like a minor consequence, it can have long-lasting negative effects depending on the learner and the situation. Use this intervention with care. Here’s an example of response cost:
You’re working with a 6-year-old boy, Travis. He is using token economy to increase his participation in classroom activities. Each time he makes an appropriate comment related to the teaching material or completes a worksheet, he earns a token. Once he has 10 tokens, he can trade them in for something from his list of available reinforcers. You decide to implement response cost to address serious, disruptive behaviors that have led to him being removed from the classroom in the past. Each time he swears in the classroom, he loses a token. The removal of tokens is response cost.

Want a resources that will help you conduct an FBA and create a function-based BIP? Check out our Master ABA Dojo membership! *
Ethical Considerations When Implementing Token Economy
The table below presents some important ethical considerations when implementing token economy with your learners. The table includes specific action steps to help you ensure you practice in an ethical way.
Research Related to Token Economy
Below is a table summarizing research articles related to implementing token economy. The table includes important action steps to help you put these ideas into practice.
References and Related Reading
Boniecki, K. A., & Moore, S. (2003). Breaking the silence: Using a token economy to reinforce classroom participation . Teaching of Psychology , 30 (3), 224-227.
Carnett, A., Raulston, T., Lang, R., Tostanoski, A., Lee, A., Sigafoos, J., & Machalicek, W. (2014). Effects of a perseverative interest-based token economy on challenging and on-task behavior in a child with autism . Journal of Behavioral Education , 23 (3), 368-377.
Doll, C., McLaughlin, T. F., & Barretto, A. (2013). The token economy: A recent review and evaluation . International Journal of basic and applied science , 2 (1), 131-149.
Falligant, J. M., & Kranak, M. P. (2022). Rate Dependence and Token Reinforcement? A Preliminary Analysis. The Psychological Record , 72 (4), 751-757.
Ivy, J. W., Meindl, J. N., Overley, E., & Robson, K. M. (2017). Token economy: A systematic review of procedural descriptions. Behavior Modification , 41 (5), 708-737.
Kazdin, A. E. (1982). The token economy: A decade later. Journal of applied behavior analysis , 15 (3), 431-445.
Kim, J. Y., Fienup, D. M., Reed, D. D., & Jahromi, L. B. (2022). A Rapid Assessment of Sensitivity to Reward Delays and Classwide Token Economy Savings for School-Aged Children. Journal of Behavioral Education , 1-24.
Massaro, I. (2022). Evaluation of the value of activity-based token reinforcement (Doctoral dissertation, Rutgers University-Graduate School of Applied and Professional Psychology).
Matson, J. L., & Boisjoli, J. A. (2009). The token economy for children with intellectual disability and/or autism: A review . Research in Developmental Disabilities , 30 (2), 240-248.
Murniyati, B., & Wardhani, J. D. (2023). Economic Token Techniques as an Effort to Increase the Independence of Children Aged 4-5 Years. Early Childhood Research Journal (ECRJ) , 5 (2), 29-43.
National Autism Center. (2009). National standards report . Retrieved December 27, 2020, from http://www.nationalautismcenter.org/
Radhamani, K., & Kalaivani, D. (2023). Token economies in the classroom: A review of literature. International Journal of Educational Psychology , 13(1), 1-15.
Richling, S. M., Williams, W. L., & Carr, J. E. (2019). The effects of different mastery criteria on the skill maintenance of children with developmental disabilities. Journal of Applied Behavior Analysis , 52 (3), 701-717.
Share this:
" * " indicates required fields
Functions of Behavior
Understanding the function of a behavior is a cornerstone of Applied Behavior Analysis.
*All images are models, not individuals with autism.
Why does Julia grab a toy from her brother? Is it to gain access to the toy? Or maybe it's to access Mom's attention?
When you can identify the function of the behavior, you can limit reinforcement for the undesired behavior and work to teach a functionally equivalent replacement behavior.
This questionnaire is designed to help you consider when a behavior is most likely to occur. There are 9 groups of 6 possibilities. In each of the 9 groups, select the option that best describes the target behavior. The results will show you a likely function of the behavior. You can retake the questionnaire to see you you get the same results.
While questionnaires are a useful tool, they should supplement (not replace) other ABA strategies including collection ABC data.
Click New Entry to begin. To update a record double click in the table or click one time and hit the Edit button (to pop up the data entry window).
To filter on a behavior, select the behavior name from the drop down (you may select multiple clients from the list). If you’re entering data for a new client you will need to refresh this page to update your filter options.
THE BALANCED BCBA
The key to breaking free from billable hours
and insurance companies.
Wait! You almost missed it!
This course is NOT available outside our membership
…unless you subscribe.

- Help & FAQ
Effects of Token Manipulation on Responding within a Token Economy Implemented with Children with Autism
Research output : Contribution to journal › Article › peer-review
Token economies are an effective behavior-management tool used across multiple populations and settings. However, researchers have shown that several variables may impact its effectiveness (Hackenberg 2009, 2018). One variable within a token economy that has not been thoroughly studied is token manipulation or the extent to which the learner physically manipulates a tangible token. Thus, the current study compared the effectiveness of a token economy when participants physically manipulated tokens (token manipulation) to when the experimenter manipulated the tokens (no manipulation). In addition, we evaluated participant preference between the two conditions (i.e., token manipulation and no manipulation). The results showed higher rates of the target response during the no token manipulation condition for one participant, while no differences were observed for the other two participants. Two participants preferred the token manipulation condition, whereas the other participant showed no preference.
- Schedule of reinforcement
- Token economy
- Token reinforcement
ASJC Scopus subject areas
- Developmental and Educational Psychology
Access to Document
- 10.1007/s43494-020-00014-2
Other files and links
- Link to publication in Scopus
- Link to the citations in Scopus
Fingerprint
- Token Economy Medicine & Life Sciences 100%
- Autistic Disorder Medicine & Life Sciences 58%
- autism Social Sciences 56%
- manipulation Social Sciences 52%
- economy Social Sciences 36%
- Child Medicine & Life Sciences 25%
- Research Personnel Medicine & Life Sciences 13%
- Population Medicine & Life Sciences 7%
T1 - Effects of Token Manipulation on Responding within a Token Economy Implemented with Children with Autism
AU - Sleiman, Andressa A.
AU - Betz, Alison M.
AU - Rey, Catalina N.
AU - Blackman, Abigail L.
N1 - Publisher Copyright: © 2020, Association for Behavior Analysis International.
PY - 2020/12
Y1 - 2020/12
N2 - Token economies are an effective behavior-management tool used across multiple populations and settings. However, researchers have shown that several variables may impact its effectiveness (Hackenberg 2009, 2018). One variable within a token economy that has not been thoroughly studied is token manipulation or the extent to which the learner physically manipulates a tangible token. Thus, the current study compared the effectiveness of a token economy when participants physically manipulated tokens (token manipulation) to when the experimenter manipulated the tokens (no manipulation). In addition, we evaluated participant preference between the two conditions (i.e., token manipulation and no manipulation). The results showed higher rates of the target response during the no token manipulation condition for one participant, while no differences were observed for the other two participants. Two participants preferred the token manipulation condition, whereas the other participant showed no preference.
AB - Token economies are an effective behavior-management tool used across multiple populations and settings. However, researchers have shown that several variables may impact its effectiveness (Hackenberg 2009, 2018). One variable within a token economy that has not been thoroughly studied is token manipulation or the extent to which the learner physically manipulates a tangible token. Thus, the current study compared the effectiveness of a token economy when participants physically manipulated tokens (token manipulation) to when the experimenter manipulated the tokens (no manipulation). In addition, we evaluated participant preference between the two conditions (i.e., token manipulation and no manipulation). The results showed higher rates of the target response during the no token manipulation condition for one participant, while no differences were observed for the other two participants. Two participants preferred the token manipulation condition, whereas the other participant showed no preference.
KW - Autism
KW - Schedule of reinforcement
KW - Token economy
KW - Token reinforcement
UR - http://www.scopus.com/inward/record.url?scp=85089016945&partnerID=8YFLogxK
UR - http://www.scopus.com/inward/citedby.url?scp=85089016945&partnerID=8YFLogxK
U2 - 10.1007/s43494-020-00014-2
DO - 10.1007/s43494-020-00014-2
M3 - Article
AN - SCOPUS:85089016945
SN - 0748-8491
JO - Education and Treatment of Children
JF - Education and Treatment of Children
The Macroeconomic Impact of Climate Change: Global vs. Local Temperature
This paper estimates that the macroeconomic damages from climate change are six times larger than previously thought. We exploit natural variability in global temperature and rely on time-series variation. A 1°C increase in global temperature leads to a 12% decline in world GDP. Global temperature shocks correlate much more strongly with extreme climatic events than the country-level temperature shocks commonly used in the panel literature, explaining why our estimate is substantially larger. We use our reduced-form evidence to estimate structural damage functions in a standard neoclassical growth model. Our results imply a Social Cost of Carbon of $1,056 per ton of carbon dioxide. A business-as-usual warming scenario leads to a present value welfare loss of 31%. Both are multiple orders of magnitude above previous estimates and imply that unilateral decarbonization policy is cost-effective for large countries such as the United States.
Adrien Bilal gratefully acknowledges support from the Chae Family Economics Research Fund at Harvard University. The views expressed herein are those of the authors and do not necessarily reflect the views of the National Bureau of Economic Research.
MARC RIS BibTeΧ
Download Citation Data
Mentioned in the News
More from nber.
In addition to working papers , the NBER disseminates affiliates’ latest findings through a range of free periodicals — the NBER Reporter , the NBER Digest , the Bulletin on Retirement and Disability , the Bulletin on Health , and the Bulletin on Entrepreneurship — as well as online conference reports , video lectures , and interviews .
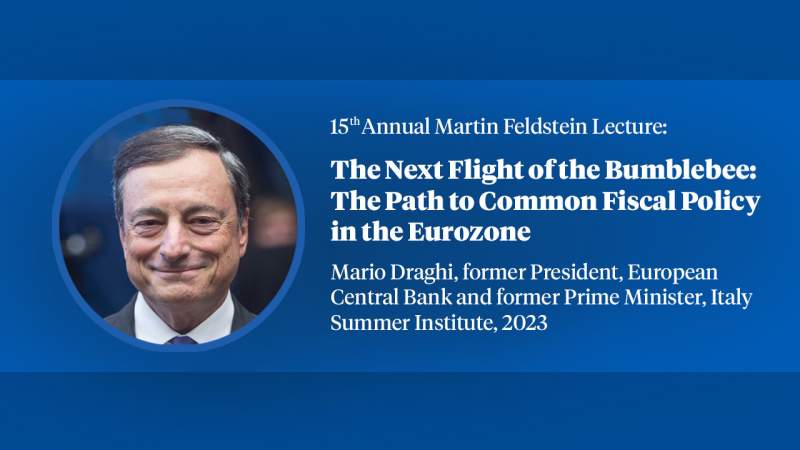
- Work & Careers
- Life & Arts
Become an FT subscriber
Try unlimited access Only $1 for 4 weeks
Then $75 per month. Complete digital access to quality FT journalism on any device. Cancel anytime during your trial.
- Global news & analysis
- Expert opinion
- Special features
- FirstFT newsletter
- Videos & Podcasts
- Android & iOS app
- FT Edit app
- 10 gift articles per month
Explore more offers.
Standard digital.
- FT Digital Edition
Premium Digital
Print + premium digital, ft professional, weekend print + standard digital, weekend print + premium digital.
Essential digital access to quality FT journalism on any device. Pay a year upfront and save 20%.
- Global news & analysis
- Exclusive FT analysis
- FT App on Android & iOS
- FirstFT: the day's biggest stories
- 20+ curated newsletters
- Follow topics & set alerts with myFT
- FT Videos & Podcasts
- 20 monthly gift articles to share
- Lex: FT's flagship investment column
- 15+ Premium newsletters by leading experts
- FT Digital Edition: our digitised print edition
- Weekday Print Edition
- Videos & Podcasts
- Premium newsletters
- 10 additional gift articles per month
- FT Weekend Print delivery
- Everything in Standard Digital
- Everything in Premium Digital
Complete digital access to quality FT journalism with expert analysis from industry leaders. Pay a year upfront and save 20%.
- 10 monthly gift articles to share
- Everything in Print
- Make and share highlights
- FT Workspace
- Markets data widget
- Subscription Manager
- Workflow integrations
- Occasional readers go free
- Volume discount
Terms & Conditions apply
Explore our full range of subscriptions.
Why the ft.
See why over a million readers pay to read the Financial Times.
International Edition

A .gov website belongs to an official government organization in the United States.
A lock ( ) or https:// means you've safely connected to the .gov website. Share sensitive information only on official, secure websites.
- Test for Fentanyl
- if You Think Someone is Overdosing
- Stop Overdose
- Naloxone FAQs
- Stigma Reduction
About Stop Overdose
- Through preliminary research and strategic workshops, CDC identified four areas of focus to address the evolving drug overdose crisis.
- Stop Overdose resources speak to the reality of drug use, provide practical ways to prevent overdoses, educate about the risks of illegal drug use, and show ways to get help.
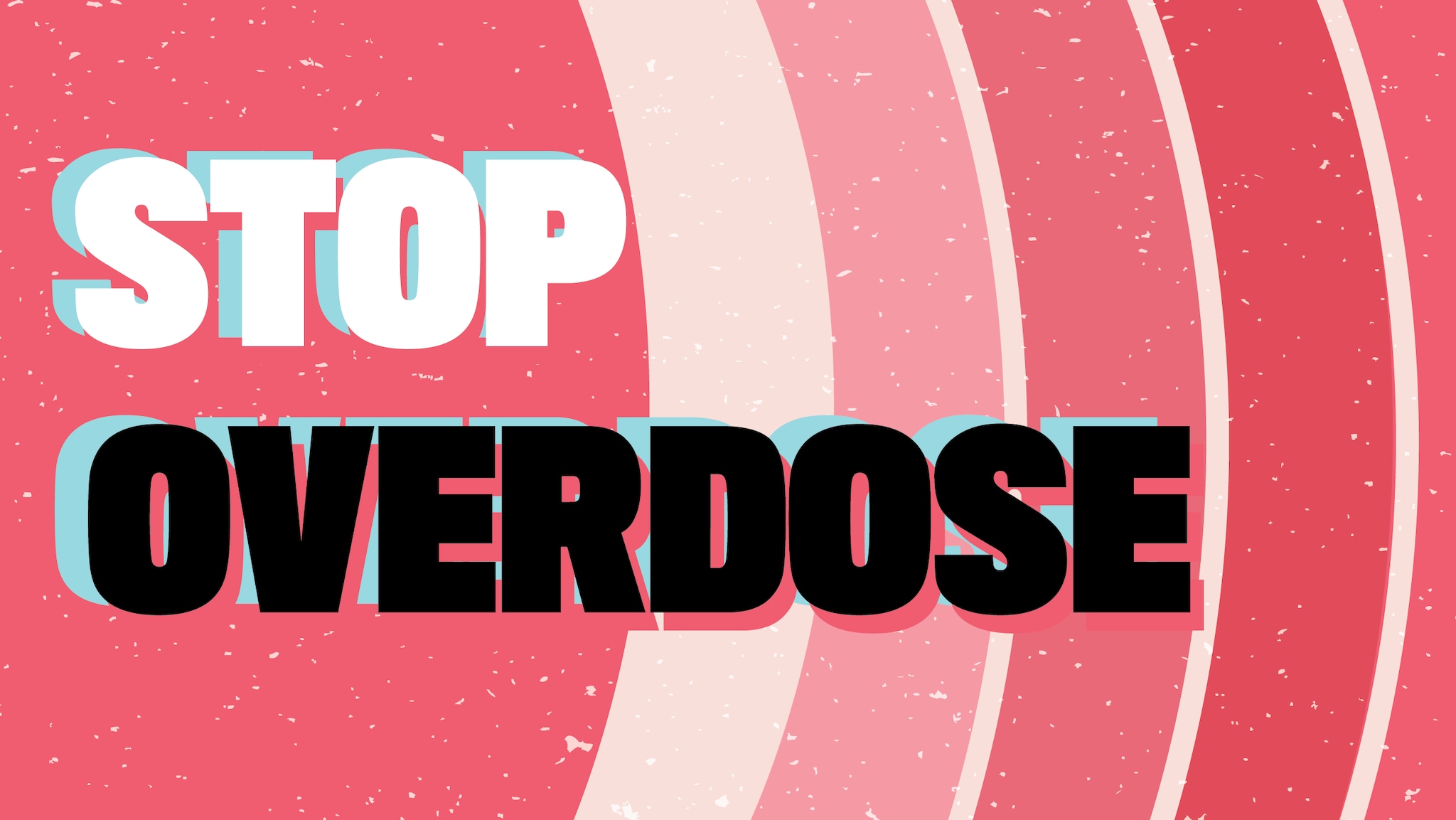
Drugs take nearly 300 lives every day. 1 To address the increasing number of overdose deaths related to both prescription opioids and illegal drugs, we created a website to educate people who use drugs about the dangers of illegally manufactured fentanyl, the risks and consequences of mixing drugs, the lifesaving power of naloxone, and the importance of reducing stigma around recovery and treatment options. Together, we can stop drug overdoses and save lives.
What you can do
- Get the facts on fentanyl
- Learn about lifesaving naloxone
- Understand the risks of polysubstance use
- Reduce stigma around recovery and treatment
Explore and download Stop Overdose and other educational materials on CDC's Overdose Resource Exchange .
- Centers for Disease Control and Prevention, National Center for Health Statistics. National Vital Statistics System, Mortality 2018-2021 on CDC WONDER Online Database, released in 2023. Data are from the Multiple Cause of Death Files, 2018-2021, as compiled from data provided by the 57 vital statistics jurisdictions through the Vital Statistics Cooperative Program. Accessed at http://wonder.cdc.gov/mcd-icd10-expanded.html on Mar 5, 2024
Every day, drugs claim hundreds of lives. The Stop Overdose website educates drug users on fentanyl, naloxone, polysubstance use, and dealing with stigma.
- Stand Up for Free Enterprise
B7 2024: Final Communiqué
B7 2024 final communiqué.
May 20, 2024
The below is the executive summary. You may download the full report on this page.
Maximizing the Benefits of AI
As a major catalyst for the transformation of our era, AI, in synergy with other enabling technologies, can enhance productivity and economic resilience, optimize the functioning of GVCs, and improve infrastructure planning, natural resource management, energy demand forecasting, and climate mitigation. Investing in AI and its applications ethically and inclusively will usher industries into the data economy, expand the labor market, and enhance progress in key sectors such as healthcare and life sciences. More effective public-private partnerships enhance education, skills, risk-based frameworks, interoperability, and capacity building, and make AI applications safer, and more secure and trustworthy.
Building upon the G7 AI Hiroshima Process, the B7 fully supports the G7 in establishing human-centered principles and standards to monitor and guide its evolution, while fostering innovation and interoperability for the benefit of all.
Enhancing Global Trade and GVCs’ Resilience
Critical dependencies should be addressed by coordinating policies, streamlining compliance costs associated with export controls and investment screening regimes, enhancing partnerships with, and investing in Least Developed Countries (LDCs), sharing frameworks to predict supply chain disruptions, and increasing preparedness and security.
In parallel, the G7 strive for a global level playing field by eliminating unjustified existing barriers and refraining from adopting new ones. The B7 is deeply concerned about the future of the WTO and reiterates its support for a rules-based multilateral trading system. Making the WTO Moratorium on Electronic Transmissions permanent is vital, while a sound reform of the Organization remains the overarching priority. The G7 policymakers should limit policy uncertainty and support businesses’ strategies, investments, and confidence. Delivering on the objectives of the PGII and the Build Back Better World (B3W) is paramount for revamping investments and supporting the sustainable growth of market economies.
Tackling Energy, Environmental, and Climate Challenges
The G7 countries’ industrial policies and regulatory frameworks should converge while ensuring energy security, competitiveness, and decarbonization. Investing in sustainable and low-carbon technologies, research and development-oriented projects, and strategic value chains according to the principle of full technological neutrality and enhancing the diversification of cost-efficient transition energy sources’ supplies and carriers would favor the affordability of energy prices during the transition phase. Public-private investment funds, convergent taxonomies and labeling, aligned incentives, and reduced divergencies in carbon markets would support the decarbonization of hard-to-abate industrial sectors, boost circular economy initiatives, energy efficiency, facilities’ reconversions and recycling, and new smart transmission and distribution infrastructures.
For a just transition, the G7 should make the Loss and Damage Mechanism operational, and promote voluntary cooperation and targeted technological transfer, while preserving intellectual and industrial property rights. It should also take into account that just transition requires the build-out of infrastructure to empower consumers and Micro, Small and Medium Enterprises (MSMEs) beyond the most advanced areas.
Embracing the Data Economy and Digital Technologies
The G7 should operationalize Data Free Flow with Trust (DFFT) through the Institutional Arrangement for Partnership (IAP) while promoting a continuous dialogue with the industry to craft regulations for a digital trust framework. Recognized evidence and risk-based standards for data flow transparency and accountability should be established, and incentives to favor trust, privacy, risk mitigation, cybersecurity, intellectual property, and interoperability should be prioritized. Promoting the development of a common G7 quantum computing ecosystem and joint research on post-quantum cryptography will enhance the security and reliability of digital infrastructures and technologies.
Also, the G7 should promote an ambitious connectivity agenda to further accelerate the take-up of advanced technologies, and enhance digitalization by spreading digital skills across businesses, administrations, and societies, notably by leveraging STEM-based pathways and the use of Digital Identity for G2B, G2C, B2B and B2C transactions.
Unleashing Talents’ Potential and Enhancing the Resilience of Welfare Systems
The B7 calls on the G7 to reform the educational systems at all levels to meet emerging job markets’ requirements and new technological trends and to facilitate the transition from education to work. The B7 renews its commitment to bridge between the workforce and employers by identifying labor market gaps, investing in skills development, and funding apprenticeship and mid-career reskilling programs. The B7 urges G7 governments to strengthen active labor market policies, promote entrepreneurship, encourage the social inclusion of underrepresented groups, particularly women and youth, and modernize welfare systems through farsighted fiscal and employment policies providing citizens with long-term equitable access to quality services in healthcare and education and address the old-age dependency.
Measuring Achievements
The B7 Italy 2024 introduces the adoption of leading Key Performance Indicators (KPIs) making the G7 and the other owners accountable for measurable outcomes. To track these KPIs over time and measure progress against the related targets, the B7 recommends the establishment of a joint G7-B7 Monitoring Committee.
- International
Recommended
- International Bolstering U.S.-Colombia Relations: Navigating Energy, Climate, Health, and Commerce As the United States and Colombia prepare for the 11th U.S.-Colombia High-Level Dialogue, it is a crucial moment to reflect on the robust and evolving partnership between the two countries. By Cesar Vence
- International Growing Green: Investing in African Agriculture Sustainably By Ellasandra Walsh
- International The Americas Act: Good Ideas, but More Work Is Needed By John G. Murphy
- International Brazil's Tax Evolution: Understanding New Opportunities for U.S. Businesses By Leonardo Abranches
- International Documents Show FTC and DOJ Influence Over U.S. Trade Policy
View this online

IMAGES
VIDEO
COMMENTS
Token economy systems have been widely used as an evidence-based classroom management strategy to reinforce and improve prosocial responses. While token economies have been widely applied to educational settings, there have been mixed results regarding the effectiveness depending on the classroom type.
Given the inherent complexity of a token economy, an analysis of the procedural descriptions may benefit future token economy research and practice. Articles published between 2000 and 2015 that included implementation of a token economy within an applied setting were identified and reviewed with a focus on evaluating the thoroughness of ...
The token economy is a well-established and widely used behavioral intervention. A token economy is comprised of six procedural components: the target response (s), a token that functions as a conditioned reinforcer, backup reinforcers, and three interconnected schedules of reinforcement. Despite decades of applied research, the extent to which ...
Recently, tokens, token sales, and the token economy were identified as topics of interest for academia within the bigger framework of blockchain-induced transformation. This includes the identification of design processes of token economy models (Kim & Chung, 2019 ) as well as the envisioning of a new business ecosystem that provides the legal ...
Although there are several meta-analyses measuring the effects of CM duration on abstinence, this is a largely understudied topic in token economy research, especially related to maintenance following the removal of a token economy intervention. The length of the token economy phases of the included studies were variable.
and evaluation on the research of token economies across settings. Key Words - Digital Search; Future Research; Literature Review; Research; Token Programs 1 Introduction This article presents a recent and inclusive review of the use of token economies in various settings. Digital and manual searches were carried using the following databases ...
This study explores the architectural framework of a value-driven token economy on a blockchain content platform and critically evaluates the relationship between blockchain's decentralization and sustainable economic practices. The existing literature often glorifies the rapid market expansion of cryptocurrencies but overlooks how underlying blockchain technology can fundamentally enhance ...
Token economies are a helpful, research-based tool for behavior intervention. However, the design and implementation process can be overwhelming. To address common challenges with token economy imp... Token economies are a helpful, research-based tool for behavior intervention. However, the design and implementation process can be overwhelming.
Token economies are made up of three interlocking contingencies including the token-production schedule, exchange-production schedule, and token-exchange schedule. Tokens must be paired with several unconditioned and conditioned reinforcers in order to serve as an effective generalized conditioned reinforcer. In this chapter, you will introduce ...
Token economy systems have been widely used as an evidence-based classroom management strategy to reinforce and improve prosocial responses. While token economies have been widely applied to educational settings, there have been mixed results regarding the effectiveness depending on the classroom type. ... Dive into the research topics of ...
A token economy is a form of behaviour modification technique intended to increase target behaviour and decrease undesirable behavior with the use of tokens. ... Research topics discussed include ...
A token economy is a system for providing positive reinforcement to a child or children by giving them tokens for completing tasks or behaving in desired ways. Token economies are used as a method of strengthening a behavior or increasing its frequency, because the tokens are a way of "rewarding" children for completing tasks. Research
both the student and the teacher. A token economy is a sys-. tem in which tokens that are earned for engaging in desired. behavior can be exchanged for items or activities that are. reinforcing ...
roots, the frequency of applied research on token economies has decreased markedly over time. If one were to conduct a keyword search with the terms token economy and token rein-forcement, one would find that the annual rate of published papers rose sharply in the late 1960s, peaked in the mid-1970s, and has since declined.
This research aims to examine the use of a token economy for education sustainability. It presents a recent review and evaluation of the token economy used among young learners and learners with special needs for behavior management and learning engagement in teaching. Online articles from Google Scholar, ERIC, and UKMLibrary were used. The terms used for reviewing the articles were token ...
Token Economy is a concept of paramount importance within the realm of behavioral psychology, holding a profound significance for the field and its practical applications. ... There is a growing interest in understanding the long-term effects of token economies on students. Research is needed to investigate whether the positive behaviors ...
Keywords: Token-based incentive systems, Tokenomics, Blockchain governance models, Decentralized systems, Sustainability aspects of BCT, Social impact of BCT . Important Note: All contributions to this Research Topic must be within the scope of the section and journal to which they are submitted, as defined in their mission statements.
A token is an object or symbol that is exchanged for goods or services. Tokens, in the form of clay coins, first appeared in human history in the transition from nomadic hunter-gatherer societies to agricultural societies, and the expansion from simple barter economies to more complex economies (Schmandt-Besserat, 1992).Since that time, token systems, in one form or another, have provided the ...
Abstract. This study investigates the effects of a token economy as an intervention to reduce disruptive and. off-task behavior of third grade students in an open concept setting. The intervention is combines. the use of a token reinforcement with a raffle style drawing. The students receive tokens on an.
A token economy is a system of contingency management based on the systematic reinforcement of target behavior. The reinforcers are symbols or tokens that can be exchanged for other reinforcers. A token economy is based on the principles of operant conditioning and behavioral economics and can be situated within applied behavior analysis.In applied settings token economies are used with ...
The token economy process in ABA is developed by Behavior Analysts (BCBAs) who tailor it to the specific needs of each learner or group. The process involves delivering tokens as a tangible conditioned reinforcer for demonstrating target behaviors. These behaviors can include tasks completion, following instructions, appropriate social ...
By Amelia Dalphonse. Within the field of Applied Behavior Analysis (ABA), professionals use reinforcement to strengthen behavior. Decades of research support the use of token economy as a means of delivering reinforcement in a variety of settings to address diverse behaviors (Matson & Boisjoli, 2009; Boniecki & Moore, 2203; Carnett et al., 2014).
Answer. It is difficult to predict with certainty what the major research topics in the fields of blockchain, token economy, metaverse, NFTs, and CBDCs will be in 2023, as these fields are ...
Dive into the research topics of 'Effects of Token Manipulation on Responding within a Token Economy Implemented with Children with Autism'. Together they form a unique fingerprint. ... One variable within a token economy that has not been thoroughly studied is token manipulation or the extent to which the learner physically manipulates a ...
Working Paper 32450. DOI 10.3386/w32450. Issue Date May 2024. This paper estimates that the macroeconomic damages from climate change are six times larger than previously thought. We exploit natural variability in global temperature and rely on time-series variation. A 1°C increase in global temperature leads to a 12% decline in world GDP.
The rent growth rate is projected to slow from 5.8 percent in the first quarter of 2024 to 4.2 percent by the end of the year. However, the model sees it picking up in the middle of 2024 and increasing to a peak of 5.4 percent in the third quarter of 2026. As in Figure 3, Figure 4 shows the 68 percent confidence interval around the forecast.
The stories that matter on money and politics in the race for the White House. The Biden administration plans to raise tariffs on Chinese electric vehicle imports from 25 per cent to 100 per cent ...
L and in Shenzhen, China's southern technology hub, is scarce. Plots in years past have grabbed sky-high prices. But when Vanke, one of the country's largest property firms, puts 19,000 square ...
Key points. Through preliminary research and strategic workshops, CDC identified four areas of focus to address the evolving drug overdose crisis. Stop Overdose resources speak to the reality of drug use, provide practical ways to prevent overdoses, educate about the risks of illegal drug use, and show ways to get help.
The B7 Italy 2024 introduces the adoption of leading Key Performance Indicators (KPIs) making the G7 and the other owners accountable for measurable outcomes. To track these KPIs over time and measure progress against the related targets, the B7 recommends the establishment of a joint G7-B7 Monitoring Committee. B7 2024 Final Communiqué.